Noise correction to improve data and model quality for crowdsourcing
Engineering Applications of Artificial Intelligence(2019)
摘要
In supervised learning, obtaining expert labeling of data is expensive and time-consuming in many cases. Crowdsourcing services provide a cheap and efficient way to acquire the labels of data. In crowdsourcing scenario, each instance obtains a multiple noisy label set from multiple different labelers, then ground truth inference algorithms are employed to obtain integrated labels of instances. In spite of the effectiveness of ground truth inference methods, a certain level of class (label) noise still exists in integrated labels, and thus class noise correction methods are dedicated to mitigate the effects of the class noise. However, to our best knowledge, there is little work, up to the present, on exploiting the information in the crowdsourcing scenario. This paper proposes a novel class noise correction method which takes advantage of the information of the multiple noisy label sets. We call our method between-class margin-based noise correction (BMNC). In BMNC, a preliminary filtering is performed before building filters to filter class noise. By the preliminary filtering, some potentially noise instances are first removed. Afterwards, a filter is built to further filter out noise instances. After these two filtering steps, a clean set and a noise set are obtained, and then a classifier is built on the clean set to relabel the instances in noise set. Experimental results on 22 simulated benchmark data sets and eight real-world crowdsourced data sets show that BMNC can significantly reduce the class noise level in integrated labels and thereby enhance the performance of target classifiers.
更多查看译文
关键词
Crowdsourcing learning,Integrated labels,Class noise,Noise correction
AI 理解论文
溯源树
样例
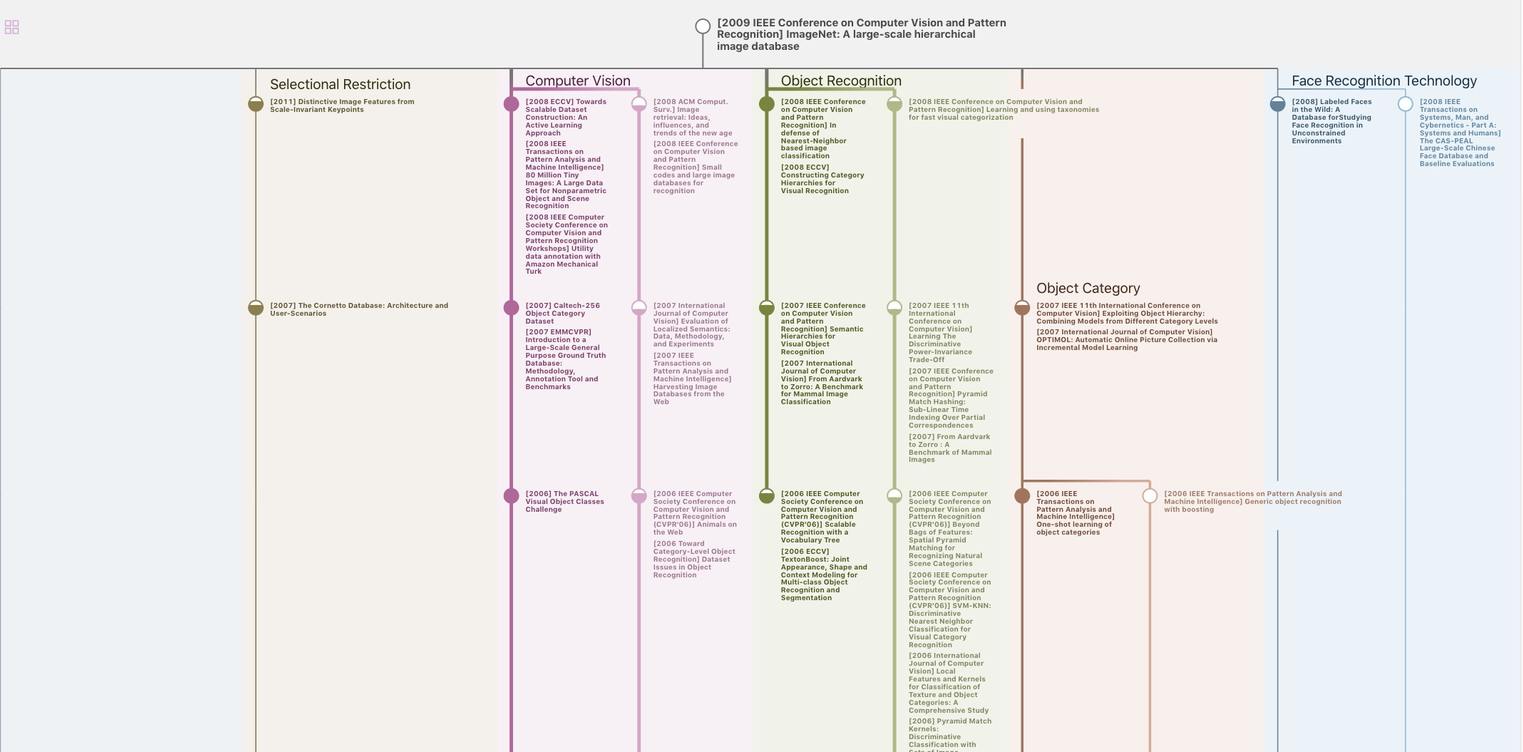
生成溯源树,研究论文发展脉络
Chat Paper
正在生成论文摘要