A nonparametric technique for analysis of state-trace functions
Journal of Mathematical Psychology(2019)
摘要
State-trace analysis provides a direct and transparent way of evaluating a question that is central to many studies of cognitive function: do one or two latent processes underlie performance on a particular task? This evaluation is made using a state-trace plot, which is a bivariate plot of two dependent variables over a dimensional variable that provides the basis for the hypothesized dissociation, and a trace variable, which enables the examination over a range of levels of performance. State-trace analysis has been used successfully in research on perception (Mccarley & Grant, 2008), attention (Verhaeghen & Cerella, 2002), memory (Dunn, 2008), and categorization (Newell, Dunn, & Kalish, 2010). However, inferential techniques for evaluating whether a given state-trace plot yields evidence for one versus two latent processes have only recently started to appear in the literature. Here we develop PIRST (Permuted Isotonic Regression for State-Trace), a fully nonparametric algorithm based on isotonic regression that can be applied to a state-trace plot and used to characterize the amount of evidence in support of one hypothesis or the other. The technique is benchmarked using simulated data, and is shown to recover the true underlying latent structure under conditions of adequate measurement. Finally, we compare PIRST to an extant technique for state-trace analysis (CMR) using ROC analysis and evaluate their respective strengths and weaknesses for diagnosing latent structure.
更多查看译文
AI 理解论文
溯源树
样例
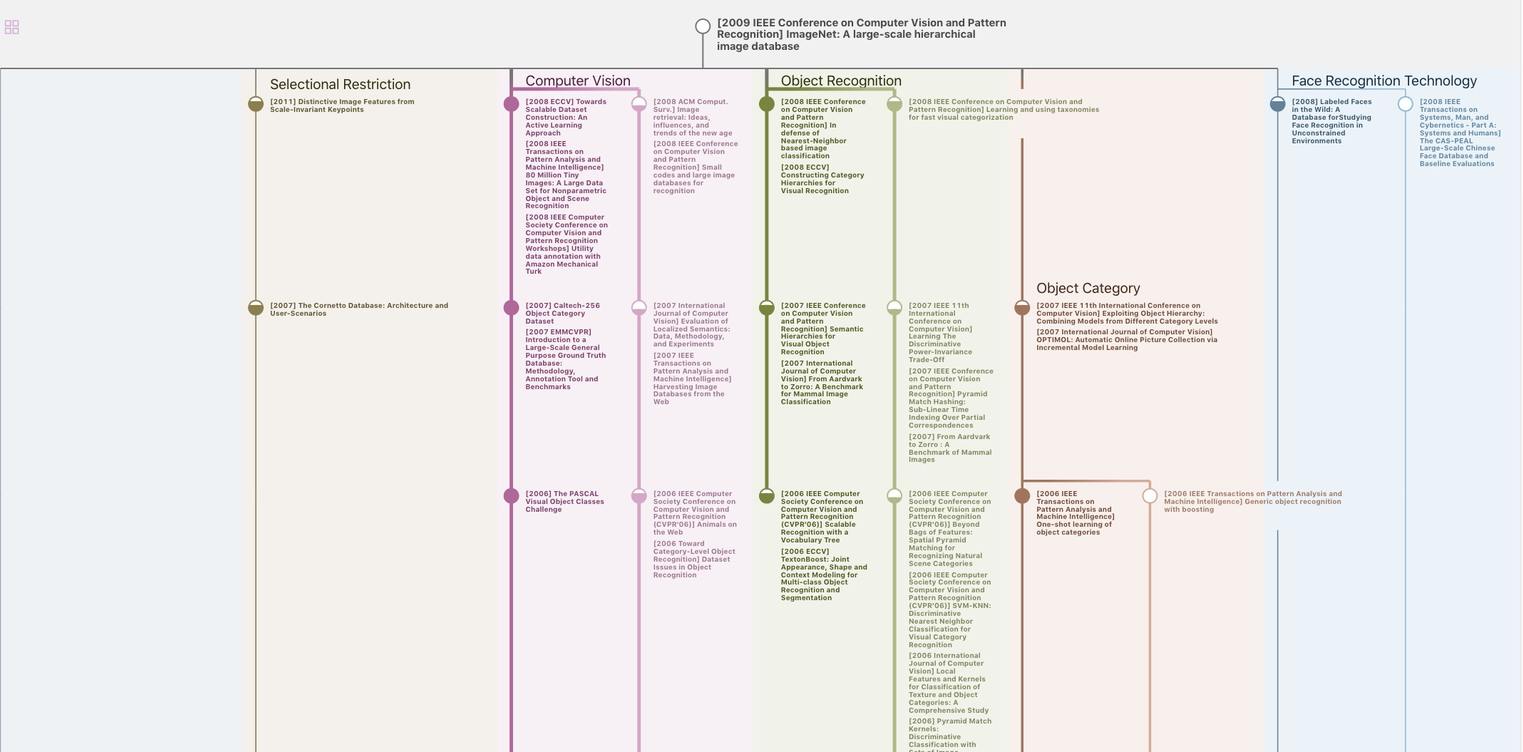
生成溯源树,研究论文发展脉络
Chat Paper
正在生成论文摘要