Learning Low Rank And Sparse Models Via Robust Autoencoders
2019 IEEE INTERNATIONAL CONFERENCE ON ACOUSTICS, SPEECH AND SIGNAL PROCESSING (ICASSP)(2019)
摘要
Robust principal component analysis (RPCA), decomposes a data matrix into a superposition of a low-rank matrix and a sparse matrix under certain incoherent conditions. In this paper, we propose a nonlinear generalization of RPCA that uses two autoencoder networks to achieve such a decomposition, in which one autoencoder accounts for the low-rank component and the other for the sparse component. To this end, we provide a principled way of constructing these autoencoders for low-rank and sparse components. The generality of the proposed model is demonstrated by applying it onto three applications, namely 1) music/voice separation 2) image denoising and 3) video foreground separation. Experimental results indicate the effectiveness of the proposed model on these application domains.
更多查看译文
关键词
Autoencoders, Low-rank, Sparsity
AI 理解论文
溯源树
样例
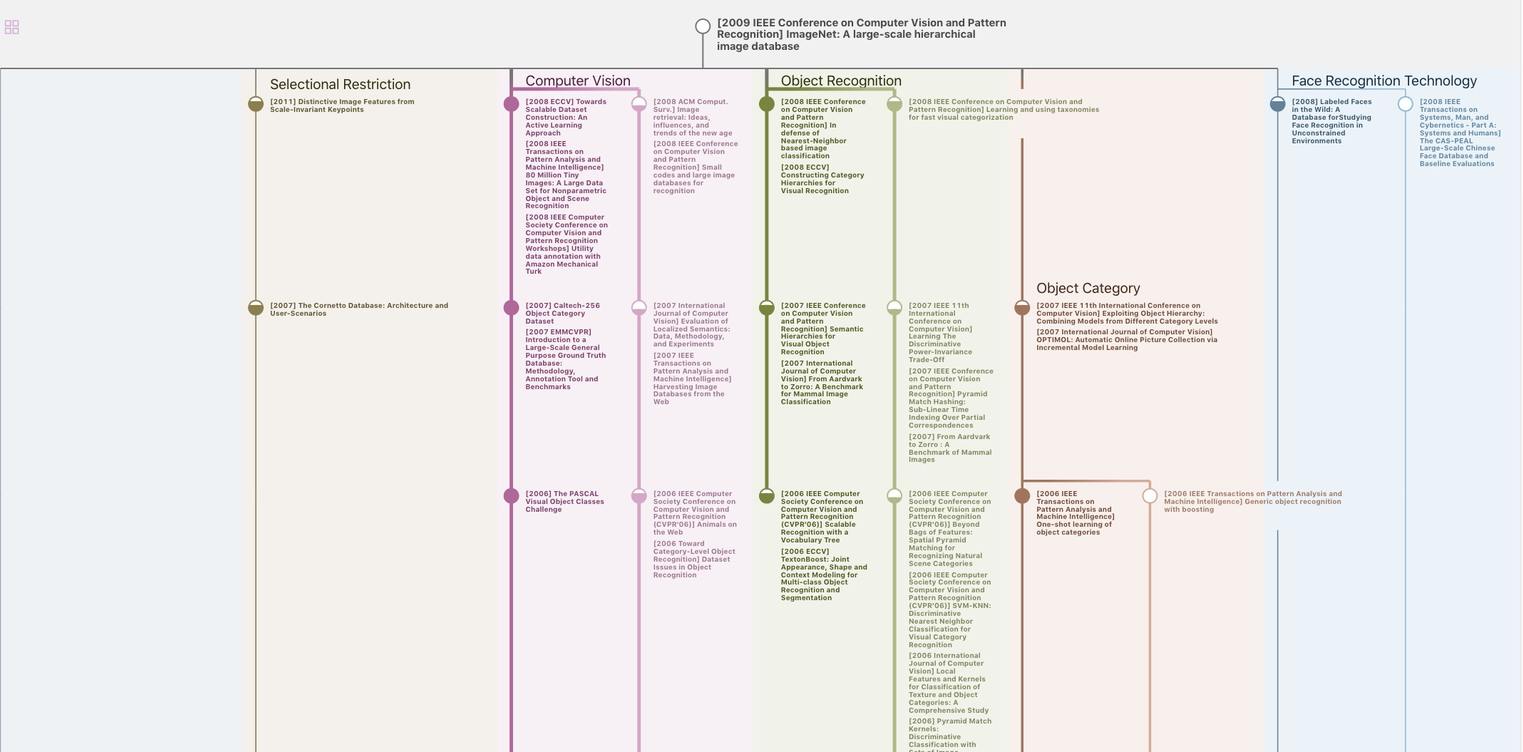
生成溯源树,研究论文发展脉络
Chat Paper
正在生成论文摘要