Curiosity-Driven Reinforcement Learning For Dialogue Management
2019 IEEE INTERNATIONAL CONFERENCE ON ACOUSTICS, SPEECH AND SIGNAL PROCESSING (ICASSP)(2019)
摘要
In this paper we describe the use of curiosity rewards for dialogue policy learning of goal oriented dialogues via reinforcement learning. Using curiosity improves state-action space exploration and helps overcome reward sparsity. Additionally, for goal oriented dialogues it makes sense to perform inherently curious actions in order to gain knowledge about the user goal. We show that intrinsic curiosity rewards can replace random epsilon-greedy exploration and stabilize training. The best results are achieved when curiosity rewards are combined with epsilon-greedy exploration.
更多查看译文
关键词
curiosity-driven, reinforcement learning, dialogue management, intrinsic rewards, exploration
AI 理解论文
溯源树
样例
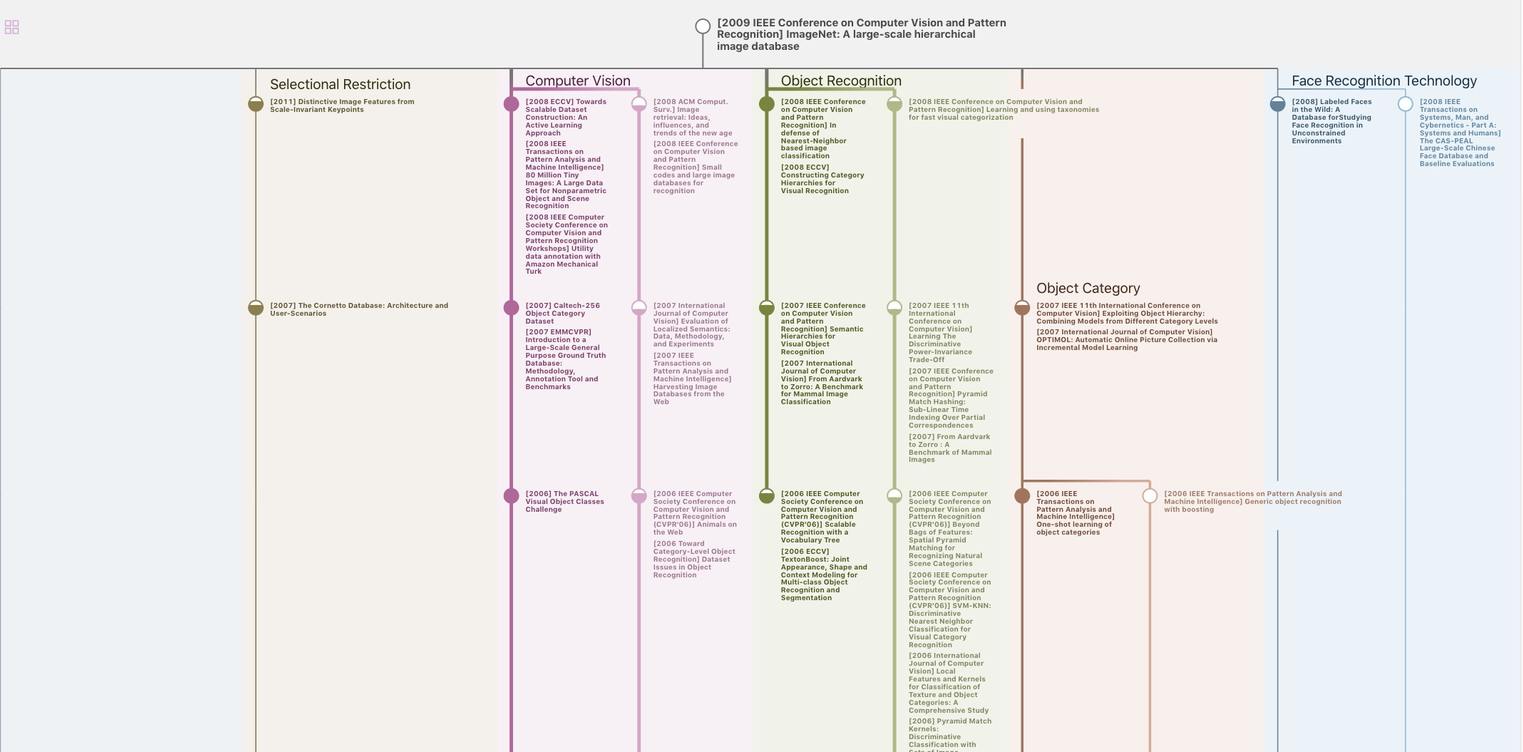
生成溯源树,研究论文发展脉络
Chat Paper
正在生成论文摘要