Boosting Memory-Based Collaborative Filtering Using Content-Metadata
SYMMETRY-BASEL(2019)
摘要
Recommendation systems are widely used in conjunction with many popular personalized services, which enables people to find not only content items they are currently interested in, but also those in which they might become interested. Many recommendation systems employ the memory-based collaborative filtering (CF) method, which has been generally accepted as one of consensus approaches. Despite the usefulness of the CF method for successful recommendation, several limitations remain, such as sparsity and cold-start problems that degrade the performance of CF systems in practice. To overcome these limitations, we propose a content-metadata-based approach that uses content-metadata in an effective way. By complementarily combining content-metadata with conventional user-content ratings and trust network information, our proposed approach remarkably increases the amount of suggested content and accurately recommends a large number of additional content items. Experimental results show a significant enhancement of performance, especially under a sparse rating environment.
更多查看译文
关键词
collaborative filtering,content-metadata,user-content rating
AI 理解论文
溯源树
样例
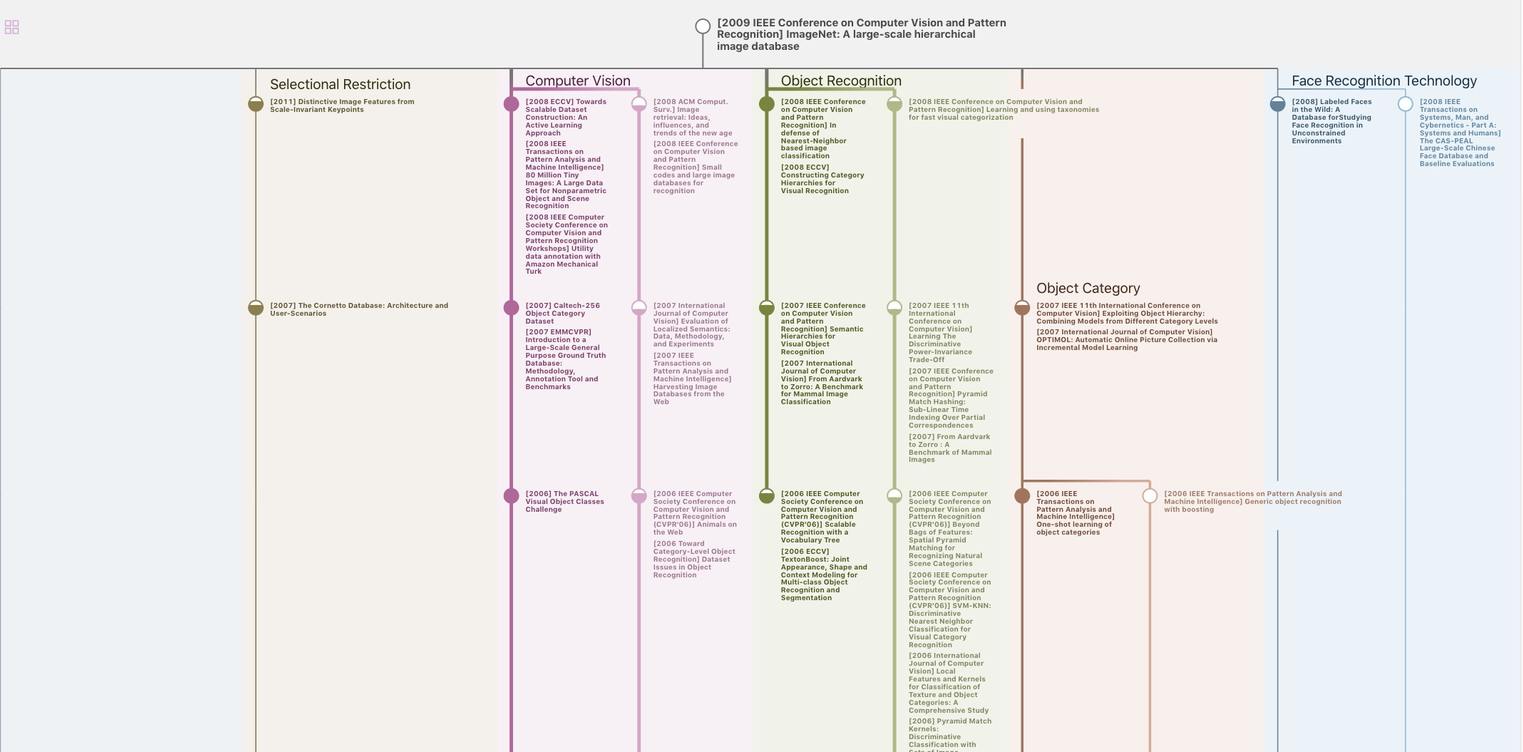
生成溯源树,研究论文发展脉络
Chat Paper
正在生成论文摘要