Deep Learning For Minimal-Context Block Tracking Through Side-Channel Analysis
2019 IEEE INTERNATIONAL CONFERENCE ON ACOUSTICS, SPEECH AND SIGNAL PROCESSING (ICASSP)(2019)
摘要
It is well known that electromagnetic and power side-channel attacks allow extraction of unintended information from a computer processor. However, little work has been done to quantify how small a sample is needed in order to glean meaningful information about a program's execution. This paper quantifies this minimum context by training a deep-learning model to track and classify program block types given small windows of side-channel data. We show that a window containing approximately four clock cycles suffices to predict block type with our experimental setup. This implies a high degree of information leakage through side channels, allowing for the external monitoring of embedded systems and Internet of Things devices.
更多查看译文
关键词
block tracking, side-channel analysis, machine learning, deep learning
AI 理解论文
溯源树
样例
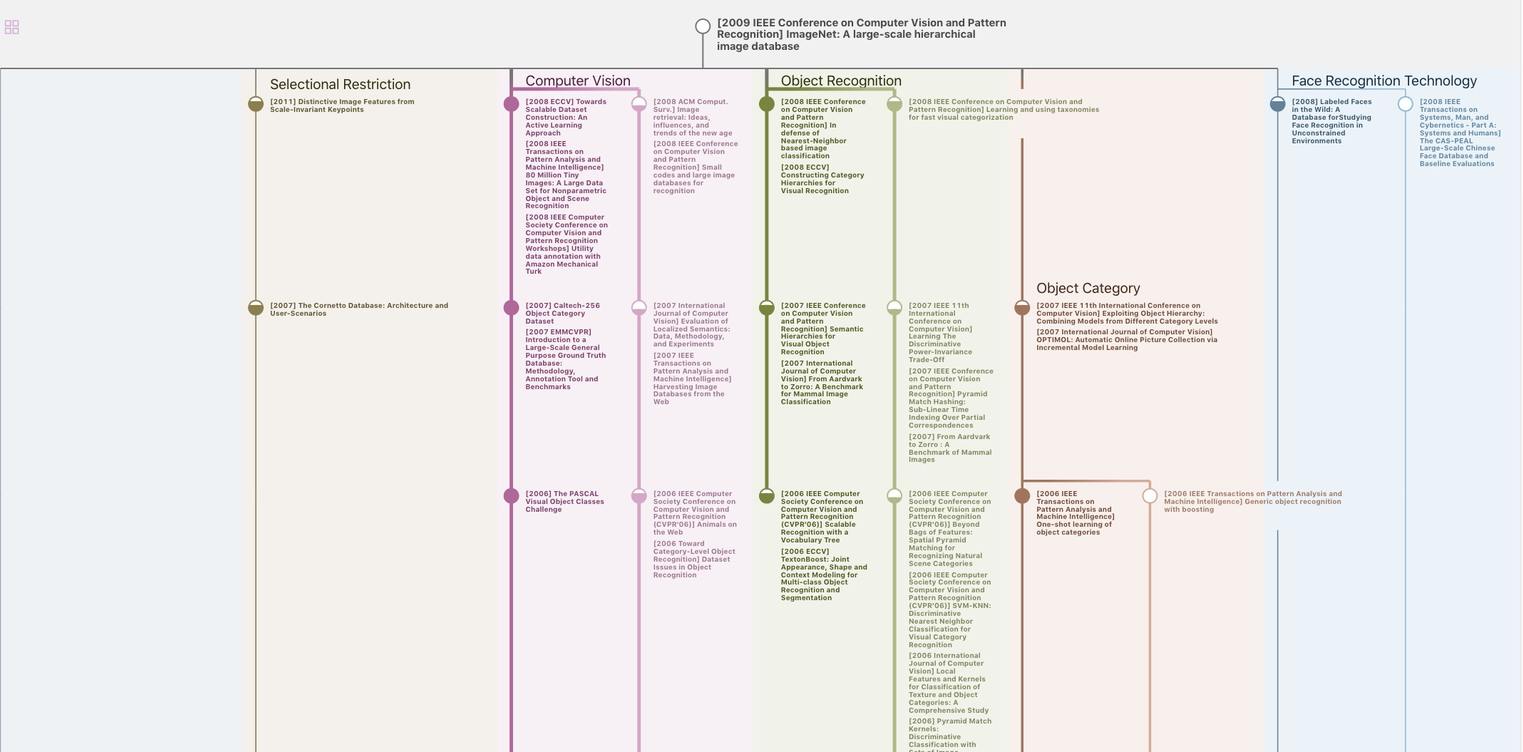
生成溯源树,研究论文发展脉络
Chat Paper
正在生成论文摘要