An Interpretable Neural Model with Interactive Stepwise Influence
pacific-asia conference on knowledge discovery and data mining(2019)
摘要
Deep neural networks have achieved promising prediction performance, but are often criticized for the lack of interpretability, which is essential in many real-world applications such as health informatics and political science. Meanwhile, it has been observed that many shallow models, such as linear models or tree-based models, are fairly interpretable though not accurate enough. Motivated by these observations, in this paper, we investigate how to fully take advantage of the interpretability of shallow models in neural networks. To this end, we propose a novel interpretable neural model with Interactive Stepwise Influence (ISI) framework. Specifically, in each iteration of the learning process, ISI interactively trains a shallow model with soft labels computed from a neural network, and the learned shallow model is then used to influence the neural network to gain interpretability. Thus ISI could achieve interpretability in three aspects: importance of features, impact of feature value changes, and adaptability of feature weights in the neural network learning process. Experiments on both synthetic and two real-world datasets demonstrate that ISI could generate reliable interpretation with respect to the three aspects, as well as preserve prediction accuracy by comparing with other state-of-the-art methods.
更多查看译文
关键词
Neural network, Interpretation, Stepwise Influence
AI 理解论文
溯源树
样例
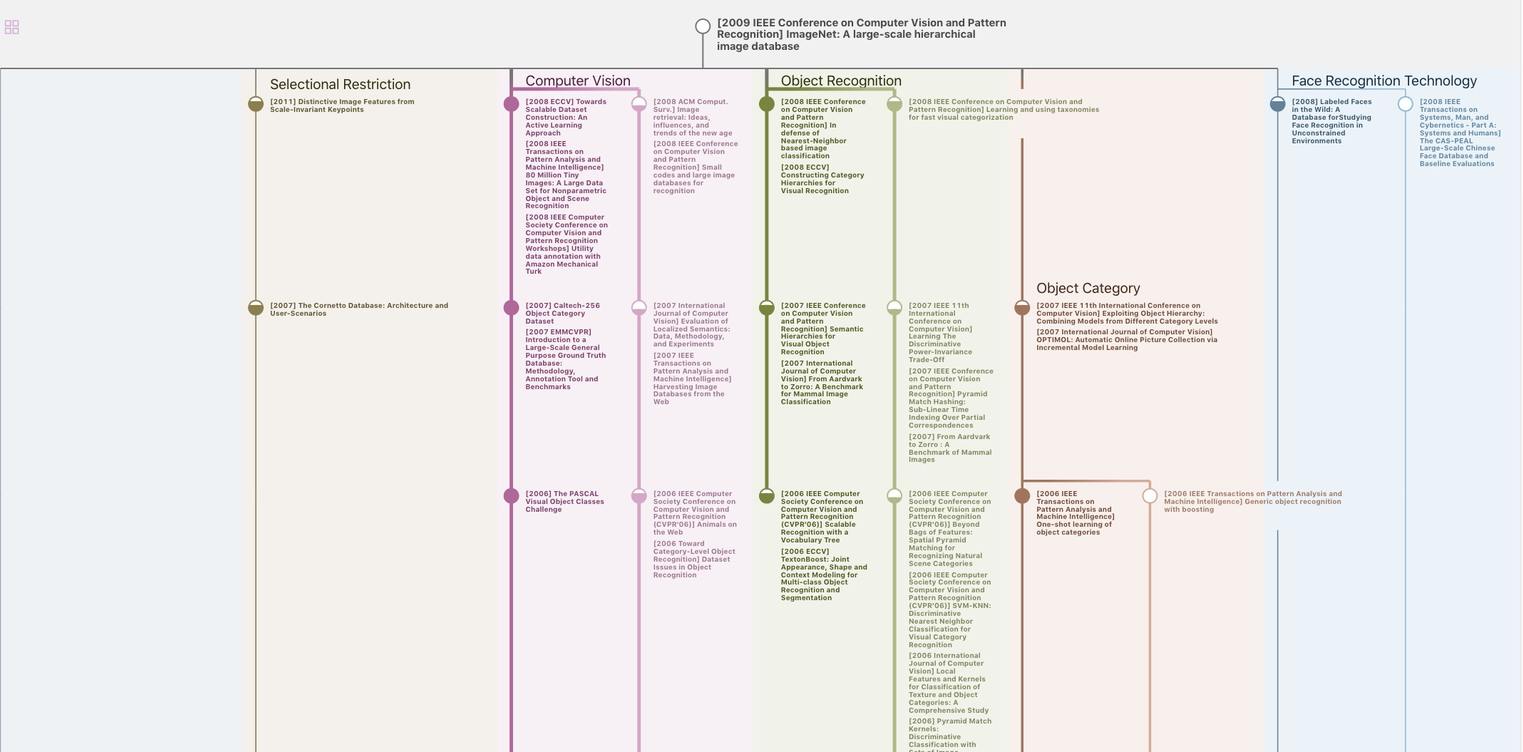
生成溯源树,研究论文发展脉络
Chat Paper
正在生成论文摘要