Learning Of Nonnegative Matrix Factorization Models For Inconsistent Resolution Dataset Analysis
IEICE TRANSACTIONS ON INFORMATION AND SYSTEMS(2019)
摘要
Due to the need to protect personal information and the impracticality of exhaustive data collection, there is increasing need to deal with datasets with various levels of granularity, such as user-individual data and user-group data. In this study, we propose a new method for jointly analyzing multiple datasets with different granularity. The proposed method is a probabilistic model based on nonnegative matrix factorization, which is derived by introducing latent variables that indicate the high-resolution data underlying the low-resolution data. Experiments on purchase logs show that the proposed method has a better performance than the existing methods. Furthermore, by deriving an extension of the proposed method, we show that the proposed method is a new fundamental approach for analyzing datasets with different granularity.
更多查看译文
关键词
inconsistent resolution dataset, probabilistic model, nonnegative matrix factorization, collective matrix factorization
AI 理解论文
溯源树
样例
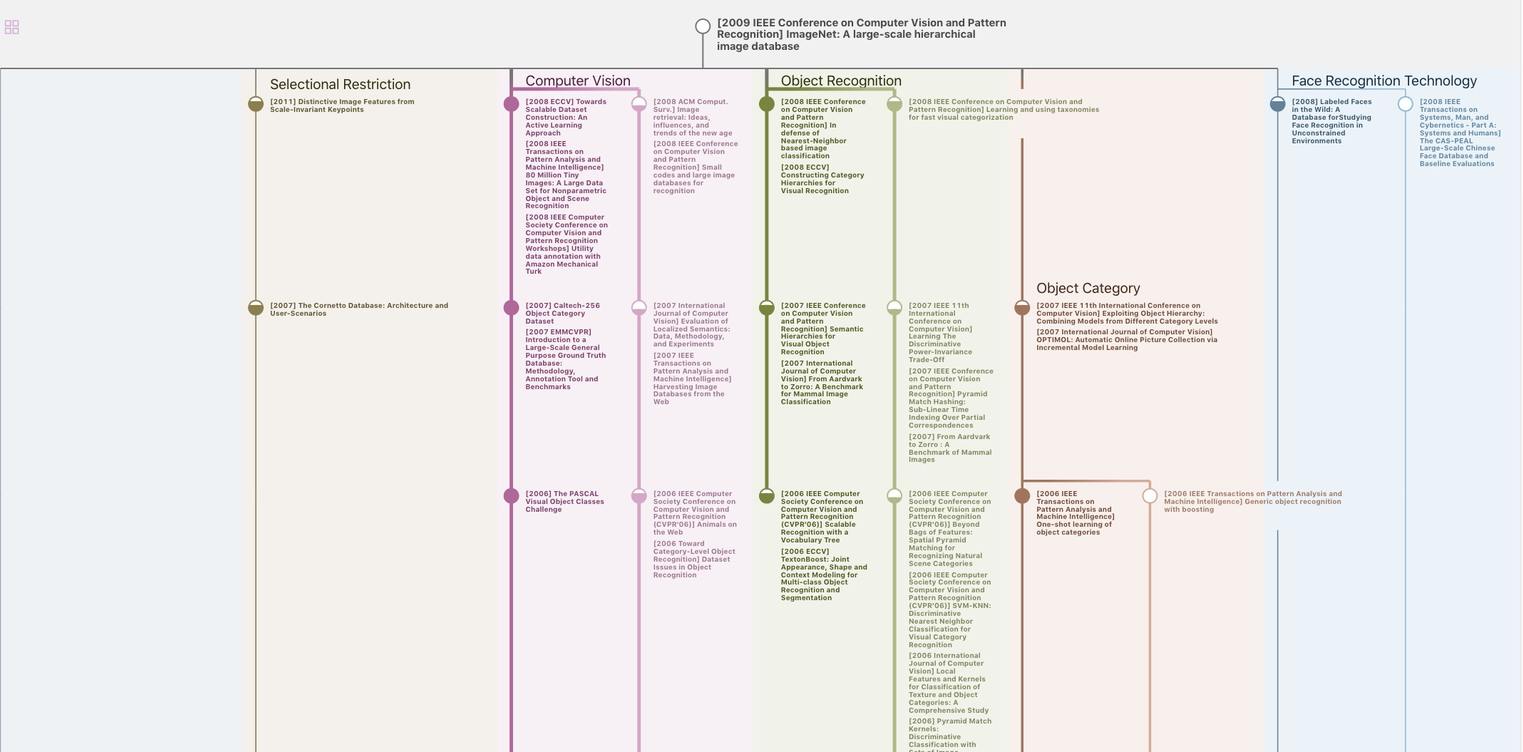
生成溯源树,研究论文发展脉络
Chat Paper
正在生成论文摘要