Analyze, Sense, Preprocess, Predict, Implement, and Deploy (ASPPID): An incremental methodology based on data analytics for cost-efficiently monitoring the industry 4.0
Engineering Applications of Artificial Intelligence(2019)
摘要
Industry 4.0 is revolutionizing decision making processes within the manufacturing industry. Among the technological portfolio enabling this revolution, the late literature has capitalized on the potential of data analytics for improving the production cycle at different stages, from resource provisioning to planning, delivery and storage. However, such a promising role of data analytics has been so far explored without a proper, quantitative inspection of the cost-improvement trade-off, nor has the process of acquiring sensors and extracting valuable information from their captured data formalized in a series of methodological steps. This paper introduces the Analyze, Sense, Preprocess, Predict, Implement and Deploy (ASPPID) methodology, an iterative decision workflow that spans from the acquisition of sensing equipment to the quantitative assessment of the contribution of their captured data to enhance the production step under focus. By placing the data scientist at the core of the workflow, this methodology helps improvement teams make informed decisions about which parts of the process need to be sensed, and how to exploit this information towards a verifiable improvement of the production cycle. The implementation of this methodology is exemplified in a real use case within the automotive industry, where the detection of defects in an annealing process can be modeled as a classification problem over a highly imbalanced dataset. Results obtained after applying the proposed ASPPID methodology show that the scrap ratio is reduced by sensing the correct part of the process at minimal investment costs, thus highlighting the crucial role of the data scientist in the management team of manufacturing plants.
更多查看译文
关键词
Industry 4.0,Methodological data analytics,Process monitoring,Cost efficiency,Imbalanced learning
AI 理解论文
溯源树
样例
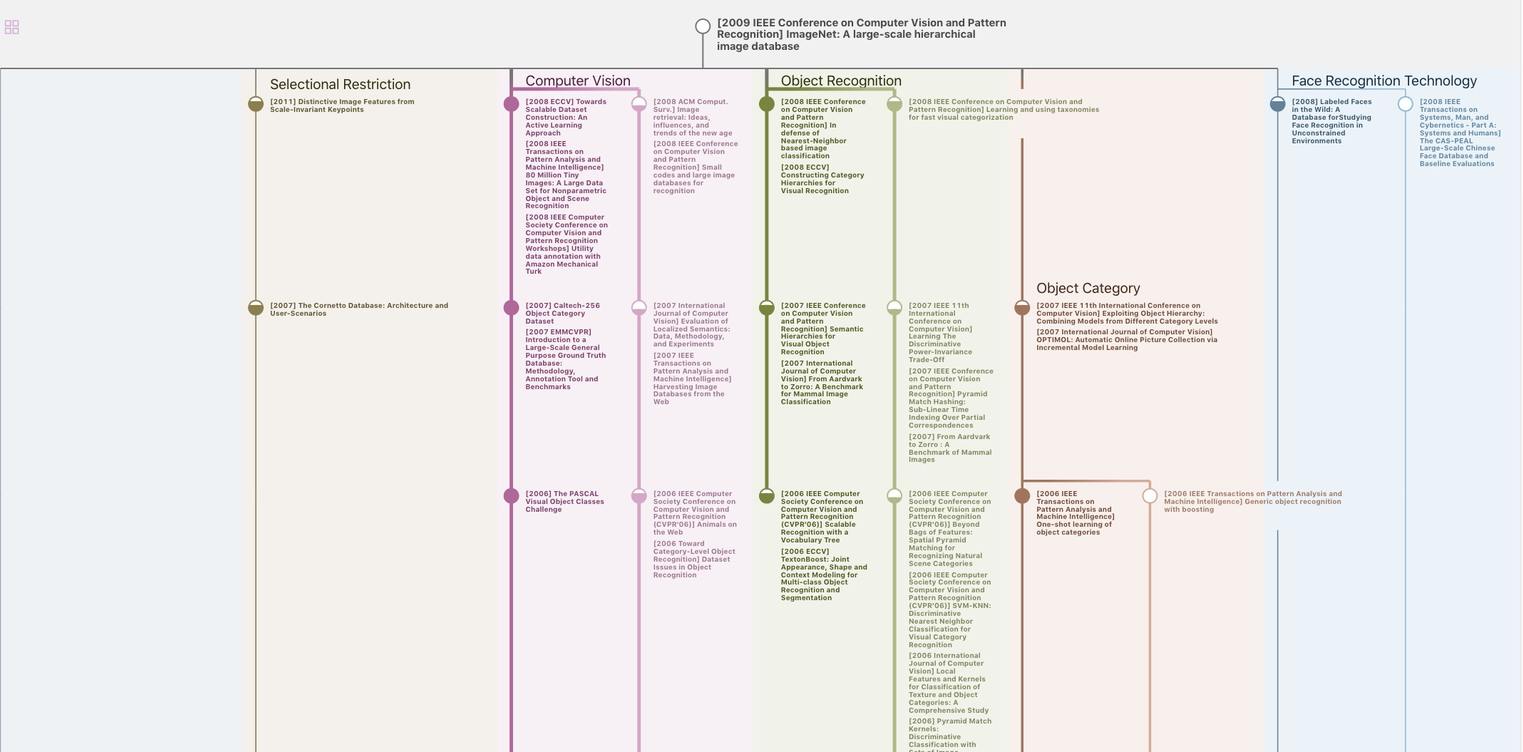
生成溯源树,研究论文发展脉络
Chat Paper
正在生成论文摘要