Convolutional neural network: Deep learning-based classification of building quality problems
Advanced Engineering Informatics(2019)
摘要
The rapid development of the construction industry in China has introduced unprecedented quality-related problems in the country’s building industry. In response to this issue, the government has established various complaint channels to report quality problems. Therefore, building quality complaints (BQCs) need to be classified and solved by respective agencies or departments rapidly for avoiding adverse impact on the safety, health, and well-being of people. However, the current process of classifying BQCs is labor intensive, time consuming, and error prone. An automatic complaint classification is required to improve the effectiveness and efficiency of complaint handling, but studies on this issue are limited. Prevailing text classification research in construction has focused on utilizing conventional shallow machine learning. By contrast, this study explores a novel convolutional neural network (CNN)-based approach that incorporates a deep-learning method to automatically classify the short texts contained within BQCs. The presented approach enables capturing the semantic features in BQC texts and automatic classification of the BQCs into predefined categories. After the model optimization, tests are conducted to examine the practical application of the text classification approach compared with Bayes-based and support vector machine classifiers. Results indicate that the developed CNN-based approach performs well in the Chinese BQC classification with limited manual intervention and few complicated feature engineering.
更多查看译文
关键词
Building quality complaints,Text classification,Convolutional neural network,Deep learning
AI 理解论文
溯源树
样例
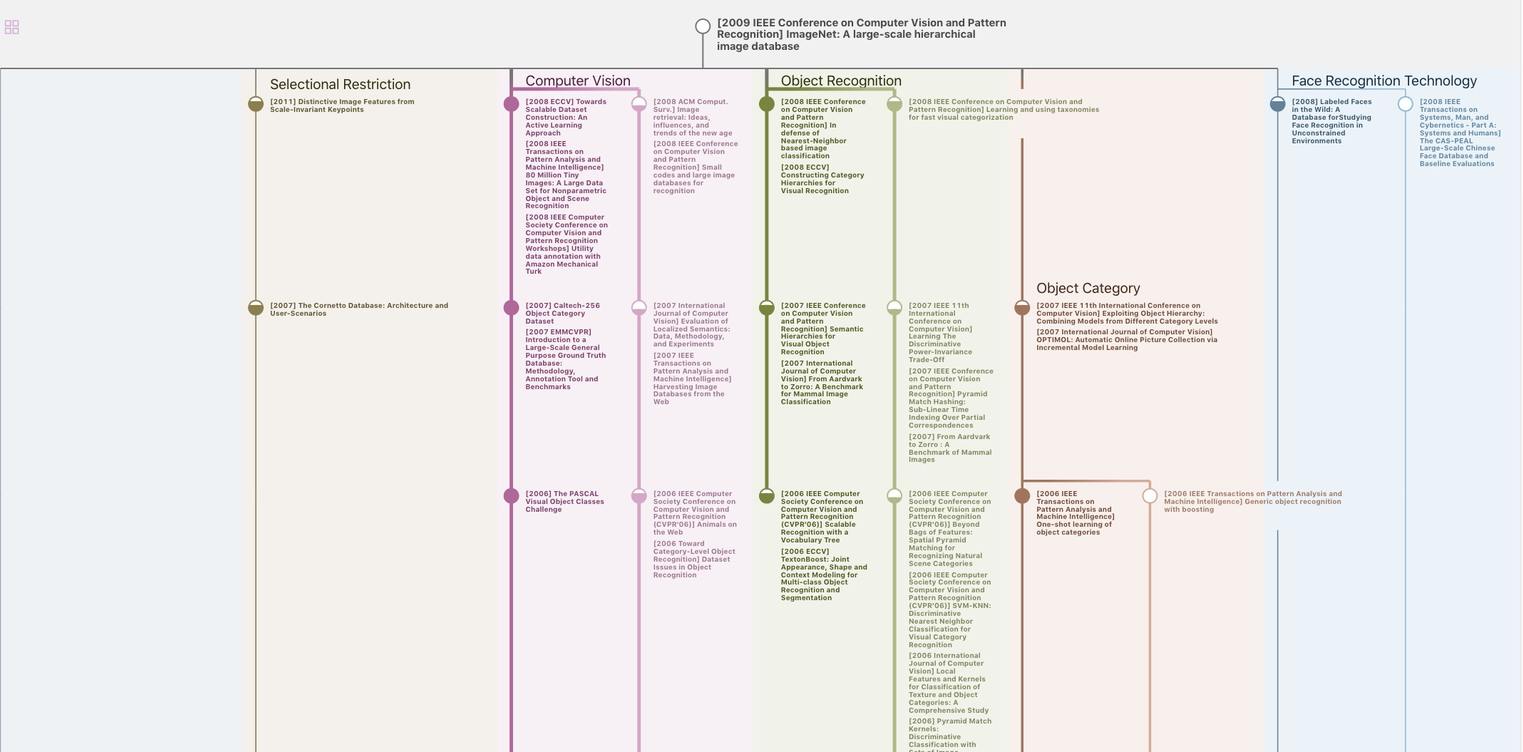
生成溯源树,研究论文发展脉络
Chat Paper
正在生成论文摘要