A Joint Two-Phase Time-Sensitive Regularized Collaborative Ranking Model for Point of Interest Recommendation
IEEE Transactions on Knowledge and Data Engineering(2020)
摘要
The popularity of location-based social networks (LBSNs) has led to a tremendous amount of user check-in data. Recommending points of interest (POIs) plays a key role in satisfying users needs in LBSNs. While recent work has explored the idea of adopting collaborative ranking (CR) for recommendation, there have been few attempts to incorporate temporal information for POI recommendation using CR. In this article, we propose a two-phase CR algorithm that incorporates the geographical influence of POIs and is regularized based on the variance of POIs popularity and users activities over time. The time-sensitive regularizer penalizes user and POIs that have been more time-sensitive in the past, helping the model to account for their long-term behavioral patterns while learning from user-POI interactions. Moreover, in the first phase, it attempts to rank visited POIs higher than the unvisited ones, and at the same time, apply the geographical influence. In the second phase, our algorithm tries to rank users favorite POIs higher on the recommendation list. Both phases employ a collaborative learning strategy that enables the model to capture complex latent associations from two different perspectives. Experiments on real-world datasets show that our proposed time-sensitive collaborative ranking model beats state-of-the-art POI recommendation methods.
更多查看译文
关键词
Collaboration,Data models,Motion pictures,Social networking (online),Urban areas,Sparse matrices,Heuristic algorithms
AI 理解论文
溯源树
样例
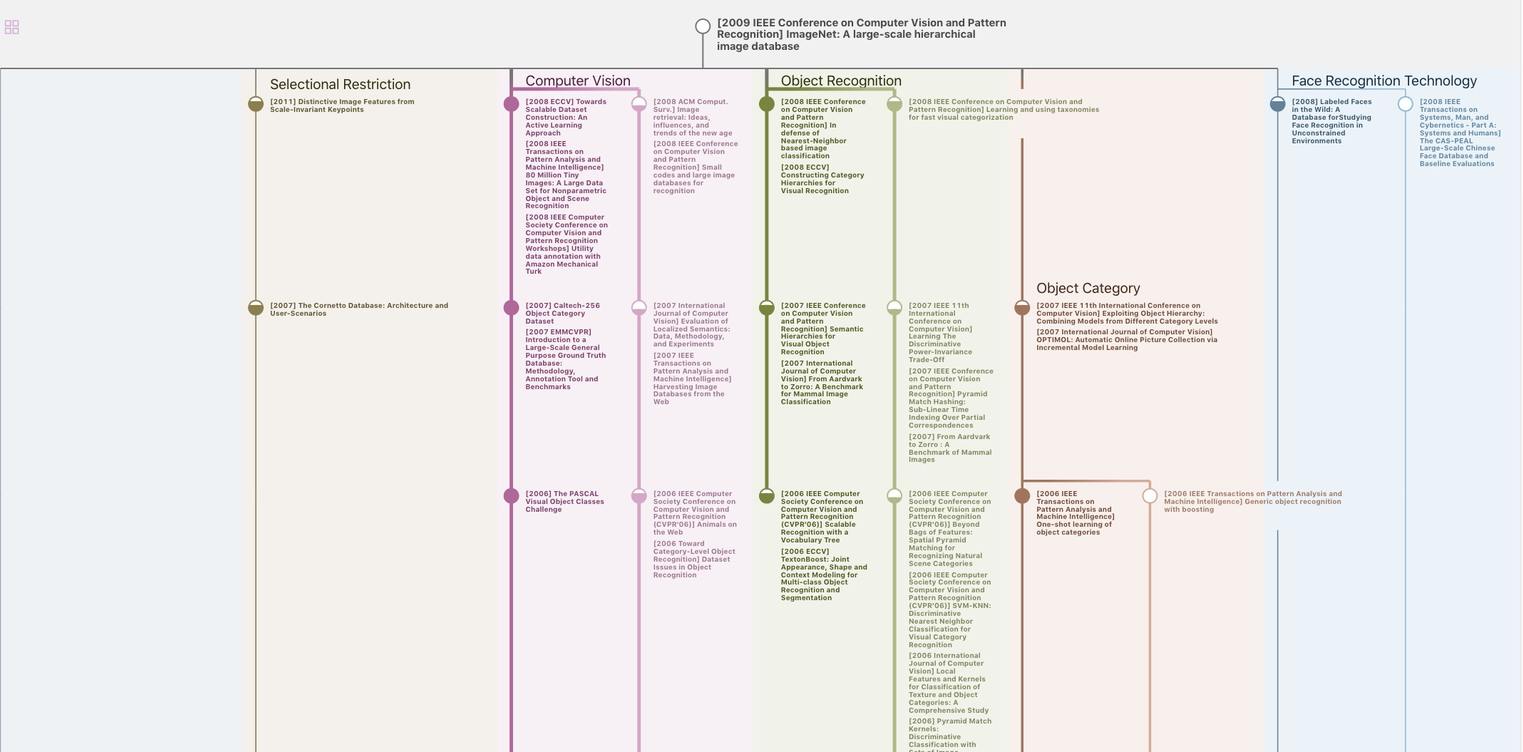
生成溯源树,研究论文发展脉络
Chat Paper
正在生成论文摘要