24.5 A Twin-8T SRAM Computation-In-Memory Macro for Multiple-Bit CNN-Based Machine Learning
international solid-state circuits conference(2019)
摘要
Computation-in-memory (CIM) is a promising avenue to improve the energy efficiency of multiply-and-accumulate (MAC) operations in AI chips. Multi-bit CNNs are required for high-inference accuracy in many applications [1–5]. There are challenges and tradeoffs for SRAM-based CIM: (1) tradeoffs between signal margin, cell stability and area overhead; (2) the high-weighted bit process variation dominates the end-result error rate; (3) trade-off between input bandwidth, speed and area. Previous SRAM CIM macros were limited to binary MAC operations for fully connected networks [1], or they used CIM for multiplication [2] or weight-combination operations [3] with additional large-area near-memory computing (NMC) logic for summation or MAC operations.
更多查看译文
关键词
AI chips,SRAM-based CIM,binary MAC operations,weight-combination operations,Twin-8T SRAM Computation-In-Memory,multiple-bit CNN-based machine learning,energy efficiency,multiply-and-accumulate operations,large-area near-memory computing,SRAM CIM macros
AI 理解论文
溯源树
样例
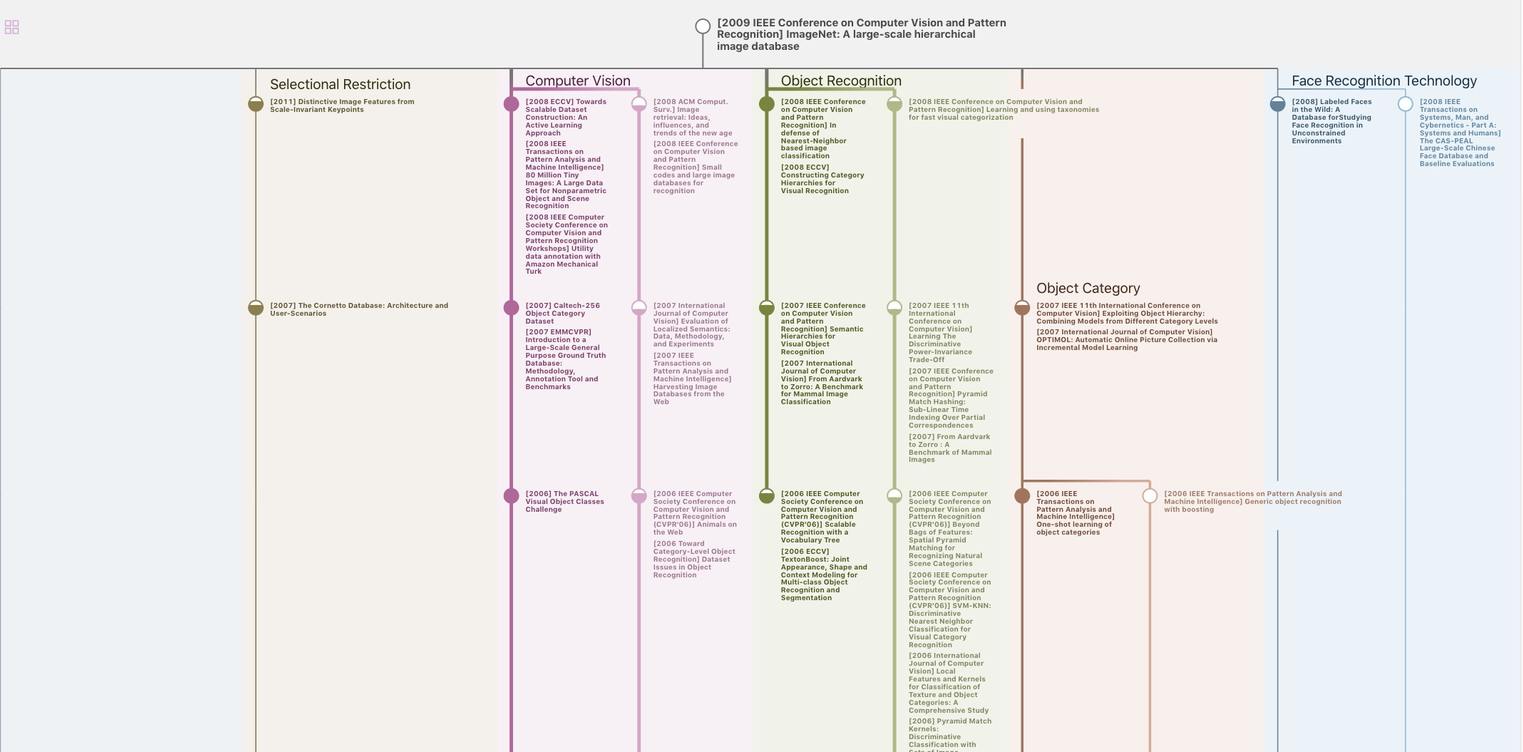
生成溯源树,研究论文发展脉络
Chat Paper
正在生成论文摘要