Accelerate Convolutional Neural Network With A Customized Vliw Dsp
PROCEEDINGS OF 2018 IEEE 9TH INTERNATIONAL CONFERENCE ON SOFTWARE ENGINEERING AND SERVICE SCIENCE (ICSESS)(2018)
摘要
Convolutional neural networks (CNN s) have achieved outstanding performance in many domains. However, the stateof-the-art CNN models also introduce massive computation and huge memory footprint. To facilitate the deployment of CNN on embedded platforms, many existing studies focus on designing dedicated hardware accelerators. But there still exists many legacy DSP-based platforms which can also be exploited to accelerate the inference of CNN. In this work, we study the computation of CNN on MaPU, which is a customized VLIW DSP. MaPU is empowered with a multi-granularity parallel memory system and a flexible program model, which is very suitable for compute-intensive tasks. Through an in-depth analysis of CNN's parallelism and the hardware architecture, we propose a kernel-expanded scheduling scheme, which can handle different kernel size uniformly. Based on our experiment on a face recognition network, MaPU achieves great performance and power efficiency.
更多查看译文
关键词
component, CNN, OSR, Accelerate, MaPU
AI 理解论文
溯源树
样例
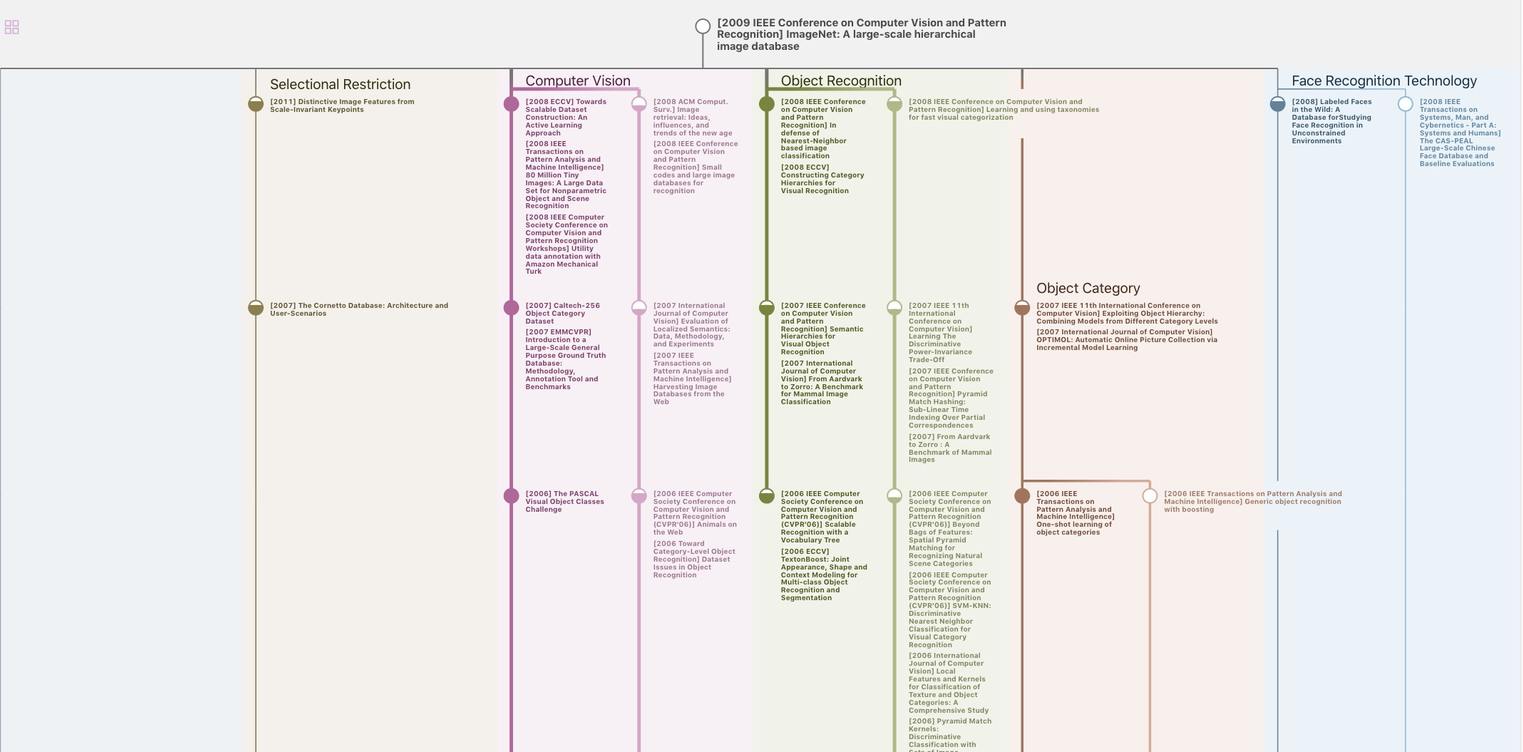
生成溯源树,研究论文发展脉络
Chat Paper
正在生成论文摘要