Affinity Regularized Non-negative Matrix Factorization for Lifelong Topic Modeling
IEEE Transactions on Knowledge and Data Engineering(2020)
摘要
Lifelong topic model (LTM), an emerging paradigm for never-ending topic learning, aims to yield higher-quality topics as time passes through knowledge accumulated from the past yet learned for the future. In this paper, we propose a novel lifelong topic model based on non-negative matrix factorization (NMF), called Affinity Regularized NMF for LTM (NMF-LTM), which to our best knowledge is distinct...
更多查看译文
关键词
Data models,Semantics,Task analysis,Graphics processing units,Big Data,Convergence,Maintenance engineering
AI 理解论文
溯源树
样例
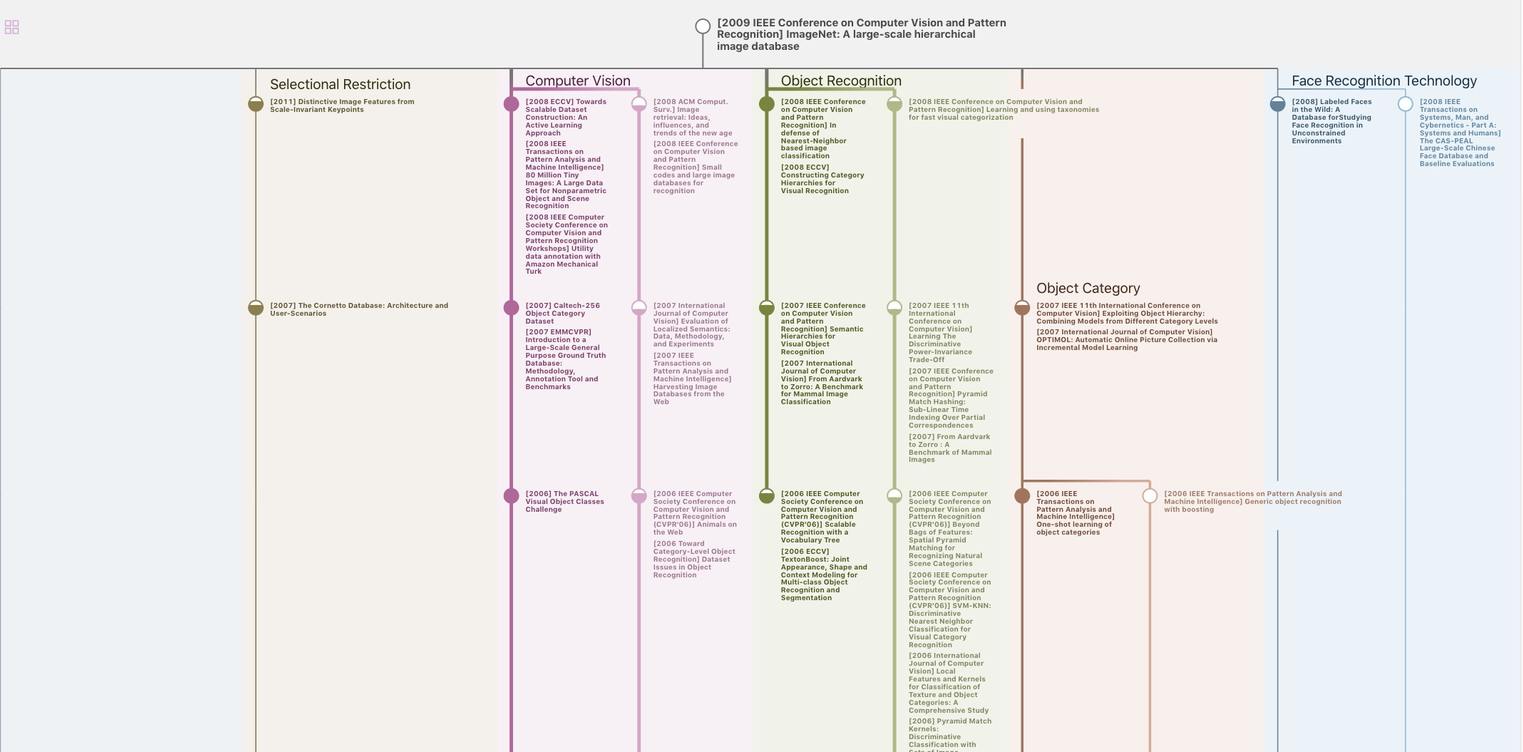
生成溯源树,研究论文发展脉络
Chat Paper
正在生成论文摘要