Fast Local Outlier Detection Algorithm Using K Kernel Space
JOURNAL OF COMPUTATIONAL METHODS IN SCIENCES AND ENGINEERING(2019)
摘要
Outlier detection can detect a small amount of data which containing valuable information from a large number of data, and it has become a hot topic in data mining. In this article, a new algorithm is proposed, which is fast local outlier detection algorithm using K kernel space. It is proposed to solve problem of the detection efficiency is not high because of the unevenness of density distribution in the outlier detection algorithm based on density, and the running time of the algorithm is obviously increased after introducing the reverse K nearest neighbors algorithm. By introducing K kernel space this algorithm divides the objects in data set into near K neighborhood points and far K neighborhood points, and reduces the number of data points which computation of the reverse K neighborhood, so as to reduce the running time of the algorithm. The accuracy of outlier detection is improved by introducing reachable distance and reachable density to reduce the statistical fluctuation of distance. Finally, the effectiveness of the proposed algorithm is shown by simulation data set and real data set.
更多查看译文
关键词
Data mining, outlier detection, K kernel space, reachable density
AI 理解论文
溯源树
样例
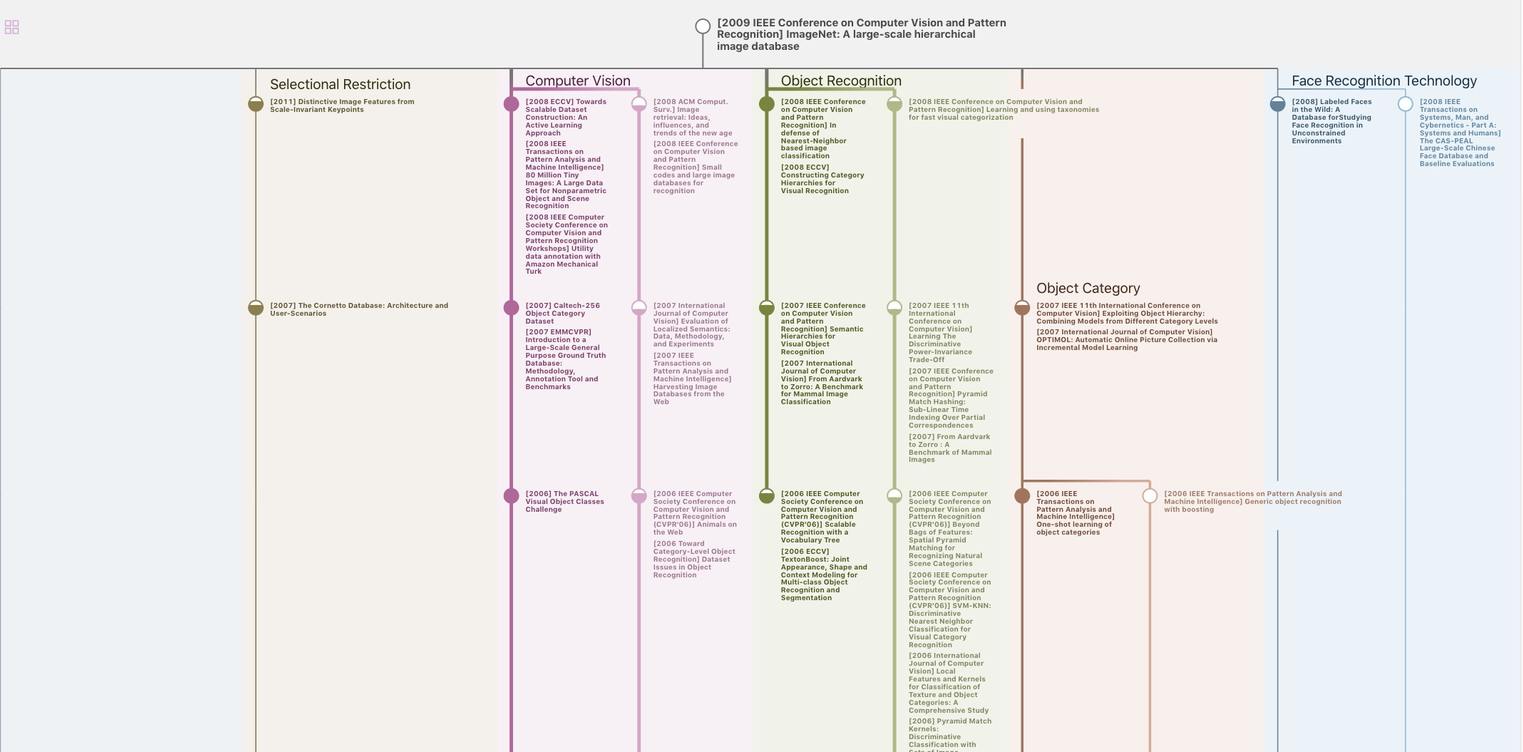
生成溯源树,研究论文发展脉络
Chat Paper
正在生成论文摘要