Streamlined Computing for Variational Inference with Higher Level Random Effects
JOURNAL OF MACHINE LEARNING RESEARCH(2020)
摘要
We derive and present explicit algorithms to facilitate streamlined computing for variational inference for models containing higher level random effects. Existing literature, such as Lee and Wand (2016), is such that streamlined variational inference is restricted to mean field variational Bayes algorithms for two-level random effects models. Here we provide the following extensions: (1) explicit Gaussian response mean field variational Bayes algorithms for three-level models, (2) explicit algorithms for the alternative variational message passing approach in the case of two-level and three-level models, and (3) an explanation of how arbitrarily high levels of nesting can be handled based on the recently published matrix algebraic results of the authors. A pay-off from (2) is simple extension to non-Gaussian response models. In summary, we remove barriers for streamlining variational inference algorithms based on either the mean field variational Bayes approach or the variational message passing approach when higher level random effects are present.
更多查看译文
关键词
Factor Graph Fragment,Longitudinal Data Analysis,Mixed Models,Multi-level Models,Variational Message Passing
AI 理解论文
溯源树
样例
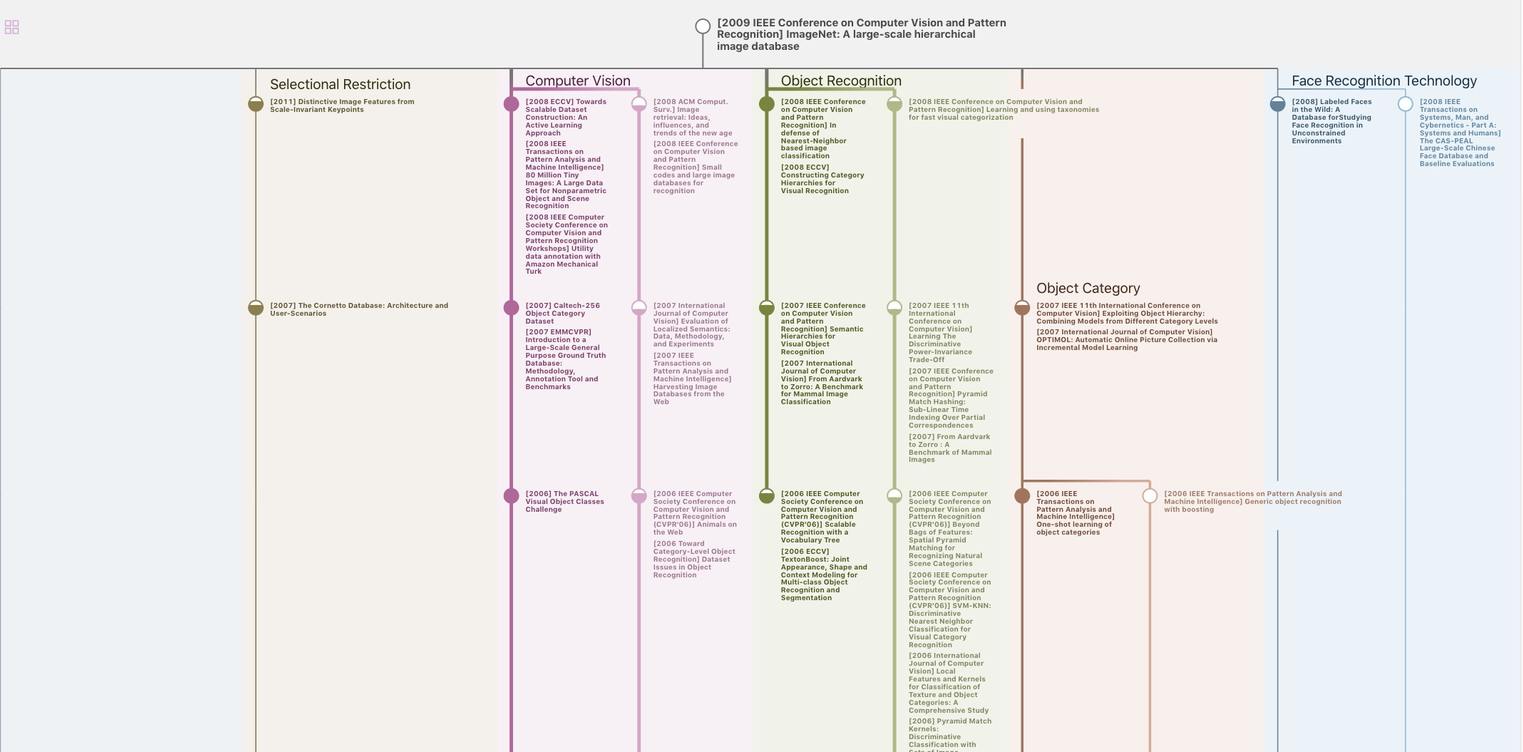
生成溯源树,研究论文发展脉络
Chat Paper
正在生成论文摘要