A novel directional and non-local-convergent particle swarm optimization based workflow scheduling in cloud–edge environment
Future Generation Computer Systems(2019)
摘要
With the increasing popularity of Internet of Things (IoT), edge computing has become the key driving force to provide computing resources, storage and network services closer to the edge on the basis of cloud computing. Workflow scheduling in such distributed environment is regarded as an NP-hard problem, and the existing approaches may not work well for task scheduling with multiple optimization goals in complex applications. As an intelligent algorithm, particle swarm optimization (PSO) has the advantages of fewer parameters, simpler algorithm and faster convergence speed, which is widely applied to workflow scheduling. However, there are also some shortcomings such as easy to fall into local optimum and sometimes difficult to obtain real optimal solution. To address this issue, first, the scheduling problem of workflow applications and objective function based on two optimized factors are clearly formalized, which can provide a theoretical foundation for workflow scheduling strategy. Then this paper proposes a novel directional and non-local-convergent particle swarm optimization (DNCPSO) that employs non-linear inertia weight with selection and mutation operations by directional search process, which can reduce the makespan and cost dramatically and obtain a compromising result. The results of simulation experiments based on various real and random workflow examples show that our DNCPSO can achieve better performance than other classical and improved algorithms, which sufficiently demonstrate the effectiveness and efficiency of DNCPSO.
更多查看译文
关键词
Cloud computing,Edge computing,Workflow scheduling,Particle swarm optimization,Makespan and cost
AI 理解论文
溯源树
样例
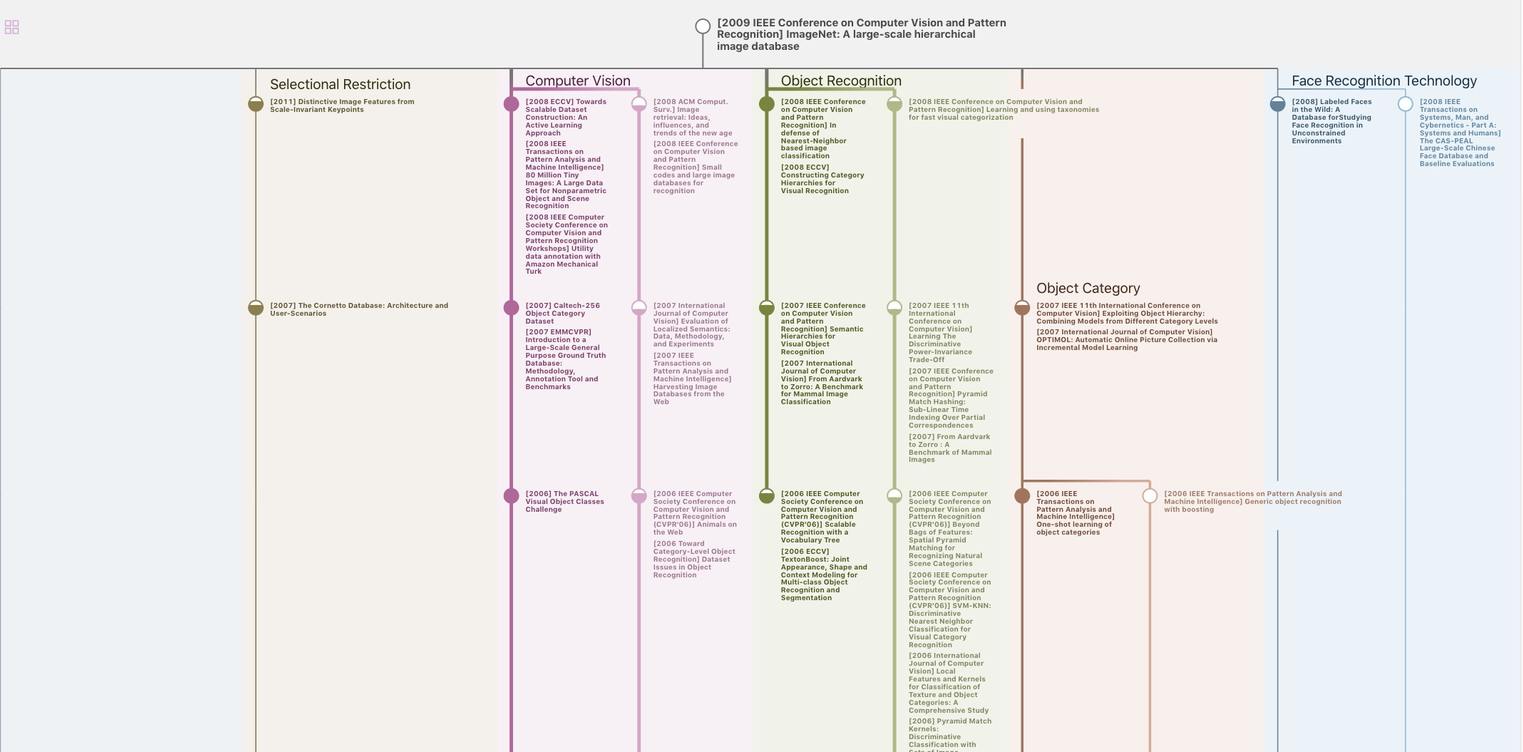
生成溯源树,研究论文发展脉络
Chat Paper
正在生成论文摘要