Systematic Characterization Of A Sequence Group
PROCEEDINGS OF THE 5TH INTERNATIONAL CONFERENCE ON INFORMATION SYSTEMS SECURITY AND PRIVACY (ICISSP)(2019)
摘要
Finding similarities in a group of sequences often involves studying their common subsequences or their common substrings. In our case, Android malware detection/classification, we study the event sequences coming from the dynamic analysis of applications. For several reasons, these sequences are mostly comprised of benign events. This specific set up makes classic sequence similarity criteria useless without any machine learning. The sequence membership to a group is characterized by subsequences of any length. Heuristic algorithms for extracting short subsequences already exist, but no attempt to solve the problem systematically has been proposed. We propose a new algorithm for building the Embedding Antichain from the set of common subsequences (noted A(Gamma)). We show that this mathematical representation is very compact and embed all common subsequences of a sequence set. It is a tool for characterizing a group of sequences. The construction of this representation reveals several complex subproblems. A few of them are solved in this article, along with practical implementations. Moreover, we solved different reduced problems and provided suboptimal solutions for the others. This article opens a new path that has cross-domain applications. Specifically, in the malware detection/classification domain the Systematic Characterization of Sequence Groups is a tool that can be used for automatic generation of malware family signatures and detection heuristics. We experimented A(Gamma) for building an Android malware family detector, on the sequences of executed Android API calls and it yields an accuracy of 97.74%.
更多查看译文
关键词
Sequence, Antichain, Android Malware Detection, Clustering, Classification
AI 理解论文
溯源树
样例
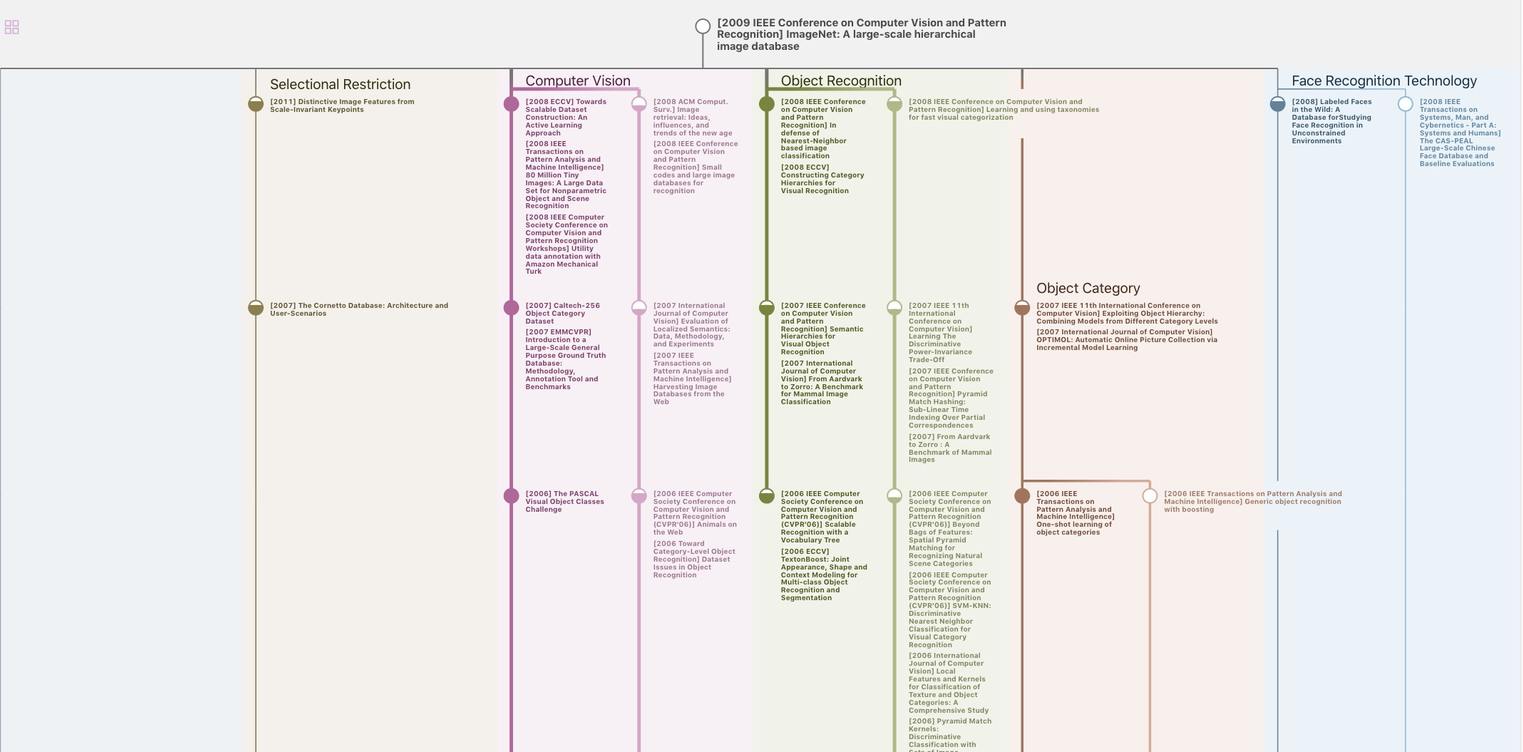
生成溯源树,研究论文发展脉络
Chat Paper
正在生成论文摘要