Learning To Remove Rain In Traffic Surveillance By Using Synthetic Data
VISAPP: PROCEEDINGS OF THE 14TH INTERNATIONAL JOINT CONFERENCE ON COMPUTER VISION, IMAGING AND COMPUTER GRAPHICS THEORY AND APPLICATIONS, VOL 4(2019)
摘要
Rainfall is a problem in automated traffic surveillance. Rain streaks occlude the road users and degrade the overall visibility which in turn decrease object detection performance. One way of alleviating this is by artificially removing the rain from the images. This requires knowledge of corresponding rainy and rain-free images. Such images are often produced by overlaying synthetic rain on top of rain-free images. However, this method fails to incorporate the fact that rain fall in the entire three-dimensional volume of the scene. To overcome this, we introduce training data from the SYNTHIA virtual world that models rain streaks in the entirety of a scene. We train a conditional Generative Adversarial Network for rain removal and apply it on traffic surveillance images from SYNTHIA and the AAU RainSnow datasets. To measure the applicability of the rain-removed images in a traffic surveillance context, we run the YOLOv2 object detection algorithm on the original and rain-removed frames. The results on SYNTHIA show an 8% increase in detection accuracy compared to the original rain image. Interestingly, we find that high PSNR or SSIM scores do not imply good object detection performance.
更多查看译文
关键词
Rain Removal, Traffic Surveillance, Image Denoising
AI 理解论文
溯源树
样例
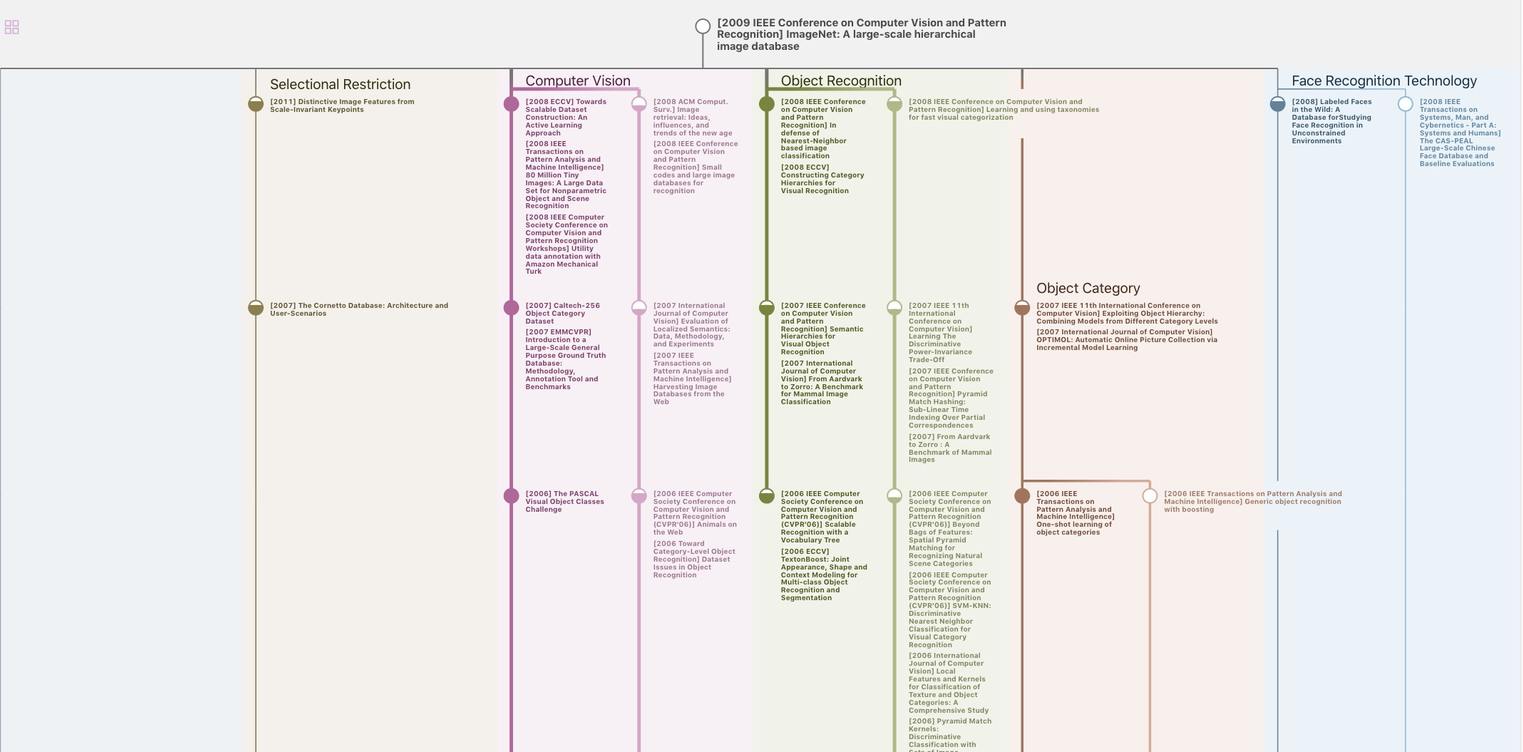
生成溯源树,研究论文发展脉络
Chat Paper
正在生成论文摘要