Human Action Recognition Using Multi-Kernel Learning For Temporal Residual Network
PROCEEDINGS OF THE 14TH INTERNATIONAL JOINT CONFERENCE ON COMPUTER VISION, IMAGING AND COMPUTER GRAPHICS THEORY AND APPLICATIONS (VISAPP), VOL 5(2019)
摘要
Deep learning has led to a series of breakthrough in the human action recognition field. Given the powerful representational ability of residual networks (ResNet), performance in many computer vision tasks including human action recognition has improved. Motivated by the success of ResNet, we use the residual network and its variations to obtain feature representation. Bearing in mind the importance of appearance and motion information for action representation, our network utilizes both for feature extraction. Appearance and motion features are further fused for action classification using a multi-kernel support vector machine (SVM). We also investigate the fusion of dense trajectories with the proposed network to boost up the network performance. We evaluate our proposed methods on a benchmark dataset (HMDB-51) and results shows the multi-kernel learning shows the better performance than the fusion of classification score from deep network SoftMax layer. Our proposed method also shows good performance as compared to the recent state-of-the-art methods.
更多查看译文
关键词
Deep Learning, Residual Network, Spatio-Temporal Network, Temporal Residual Network, Human Action Recognition
AI 理解论文
溯源树
样例
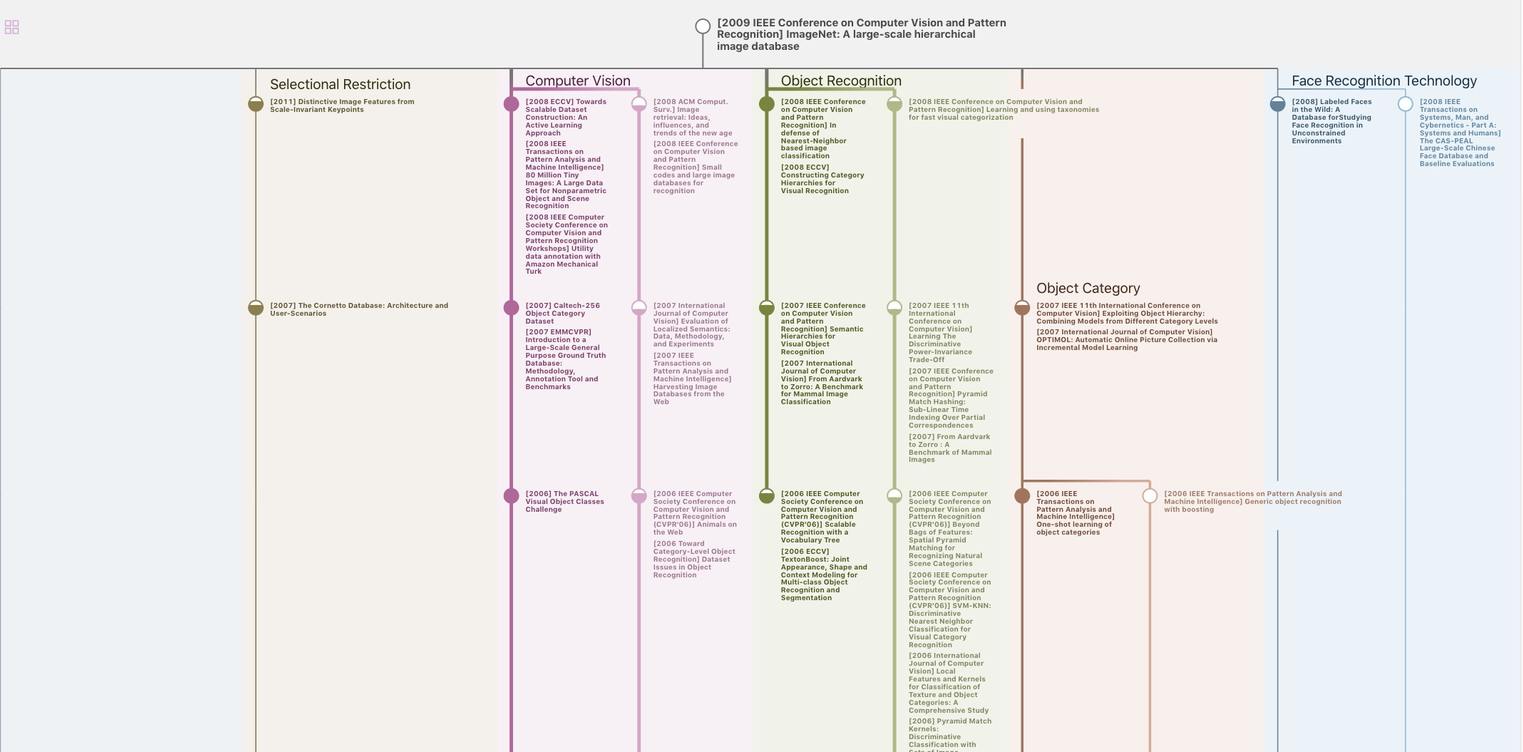
生成溯源树,研究论文发展脉络
Chat Paper
正在生成论文摘要