Mind: Model Independent Neural Decoder
2019 IEEE 20TH INTERNATIONAL WORKSHOP ON SIGNAL PROCESSING ADVANCES IN WIRELESS COMMUNICATIONS (SPAWC 2019)(2019)
摘要
Standard decoding approaches rely on model-based channel estimation methods to compensate for varying channel effects, which degrade in performance whenever there is a model mismatch. Recently proposed Deep learning based neural decoders address this problem by leveraging a model-free approach via gradient-based training. However, they require large amounts of data to retrain to achieve the desired adaptivity, which becomes intractable in practical systems.In this paper, we propose a new decoder: Model Independent Neural Decoder (MIND), which builds on the top of neural decoders and equips them with a fast adaptation capability to varying channels. This feature is achieved via the methodology of Model-Agnostic Meta-Learning (MAML). Here the decoder: (a) learns a 'good' parameter initialization in the meta-training stage where the model is exposed to a set of archetypal channels and (b) updates the parameter with respect to the observed channel in the meta-testing phase using minimal adaptation data and pilot bits. Building on top of existing state-of-the-art neural Convolutional and Turbo decoders, MIND outperforms the static benchmarks by a large margin and shows minimal performance gap when compared to the neural (Convolutional or Turbo) decoders designed for that particular channel. In addition, MIND also shows strong learning capability for channels not exposed during the meta training phase.
更多查看译文
关键词
standard decoding approaches,model-based channel estimation methods,channel effects,model-free approach,gradient-based training,archetypal channels,model independent neural decoder,model-agnostic meta-learning,MIND,deep learning based neural decoders,meta-testing phase,neural convolutional,turbo decoders
AI 理解论文
溯源树
样例
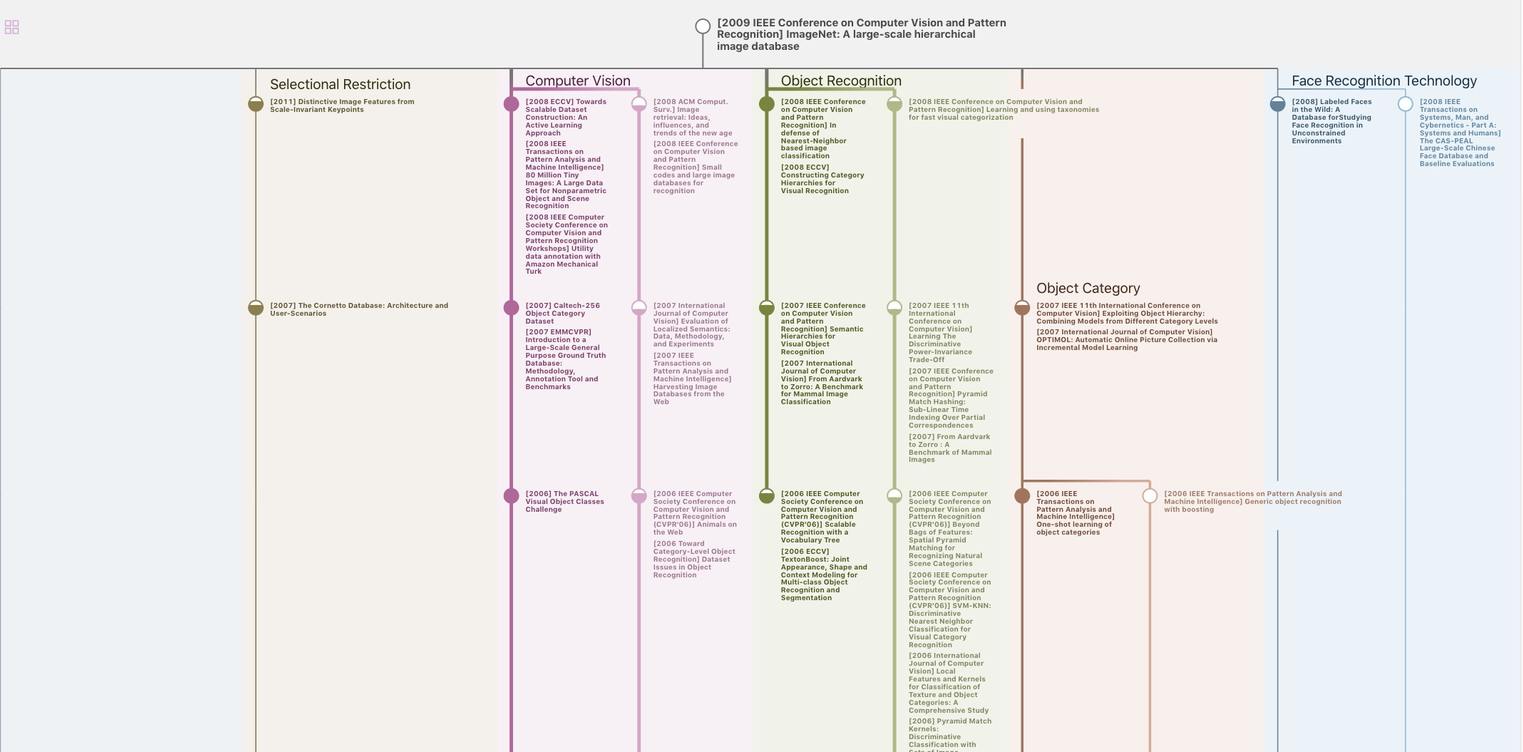
生成溯源树,研究论文发展脉络
Chat Paper
正在生成论文摘要