Machine Learning Estimates of Global Marine Nitrogen Fixation
JOURNAL OF GEOPHYSICAL RESEARCH-BIOGEOSCIENCES(2019)
摘要
Marine nitrogen (N-2) fixation supplies "new" nitrogen to the global ocean, supporting uptake and sequestration of carbon. Despite its central role, marine N-2 fixation and its controlling factors remain elusive. In this study, we compile over 1,100 published observations to identify the dominant predictors of marine N-2 fixation and derive global estimates based on the machine learning algorithms of random forest and support vector regression. We find that no single environmental property predicts N-2 fixation at global scales. Our random forest and support vector regression algorithms, trained with sampling coordinates and month, solar radiation, wind speed, sea surface temperature, sea surface salinity, surface nitrate, surface phosphate, surface excess phosphorus, minimum oxygen in upper 500m, photosynthetically available radiation, mixed layer depth, averaged photosynthetically available radiation in the mixed layer, and chlorophyll-a concentration, estimate global marine N-2 fixation ranging from 68 to 90TgN/year. Comparison of our machine learning estimates and 11 other model outputs currently available in literature shows substantial discrepancies in the global magnitude and spatial distribution of marine N-2 fixation, especially in the tropics and in high latitudes. The large uncertainties in marine N-2 fixation highlighted in our study argue for increased and more coordinated efforts using geochemical tracers, modeling, and observations over broad ocean regions.
更多查看译文
关键词
N-2 fixation,machine-learning,ocean
AI 理解论文
溯源树
样例
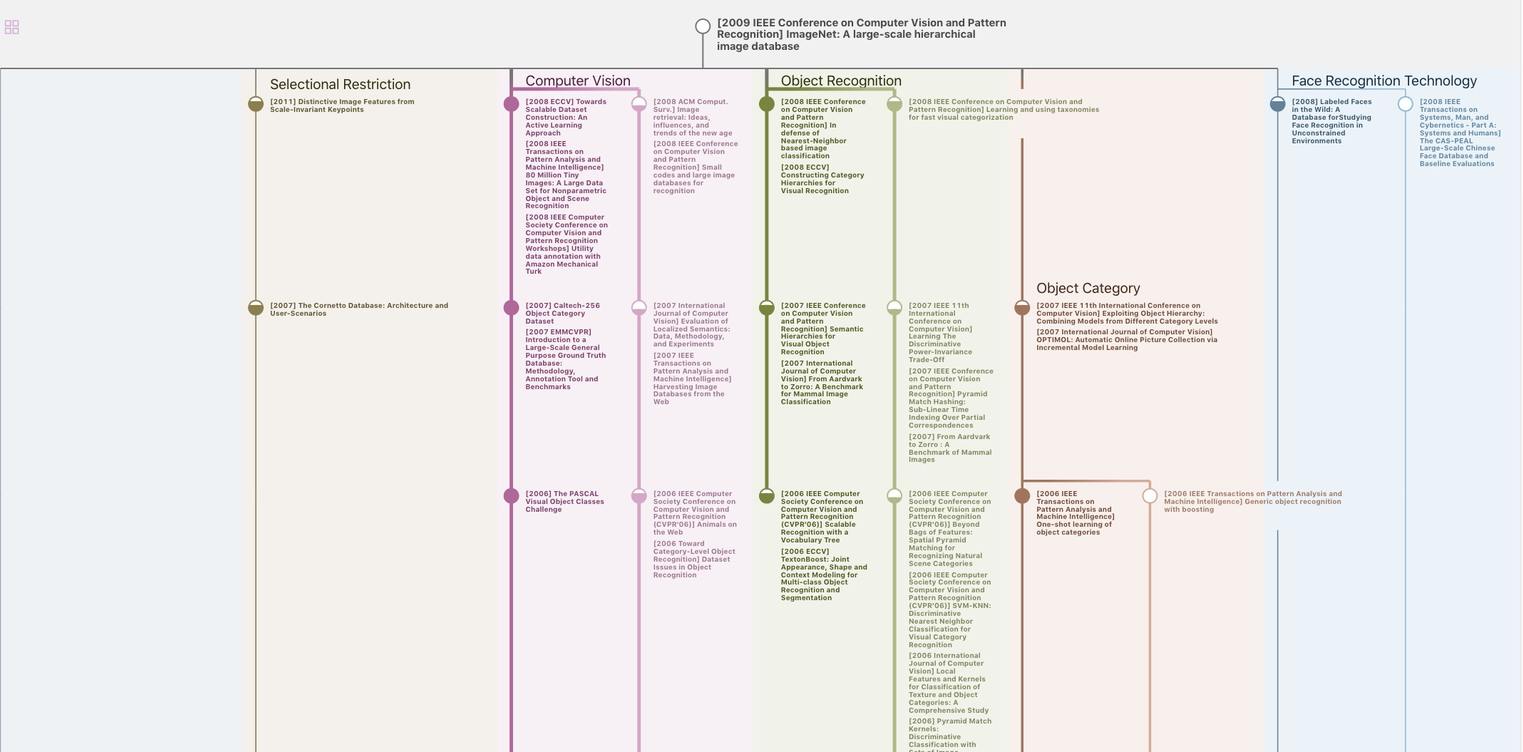
生成溯源树,研究论文发展脉络
Chat Paper
正在生成论文摘要