Machine Learning on High-Dimensional Data to Predict Bleeding Post Percutaneous Coronary Intervention.
JOURNAL OF THE AMERICAN COLLEGE OF CARDIOLOGY(2020)
摘要
Introduction. The purpose of the current study is to determine the accuracy of machine learning in predicting bleeding outcomes post percutaneous coronary intervention (PCI) in comparison with the American College of Cardiology CathPCI bleeding risk (ACC-BR) model. Methods. Mayo Clinic CathPCI registry data were retrospectively analyzed from January, 2003 to June, 2018, including 15,603 patients who underwent PCI. The cohort was randomly divided into a training sample of 11,703 patients (75%) and a unique test sample of 3900 patients (25%) prior to model generation. The risk-prediction model was generated utilizing a boosted classification tree algorithm of 105 unique variables to predict the risk of major and minor bleeding complications within 72 hours after PCI or before hospital discharge. The receiver operating characteristic (ROC) curves and areas under the curve (AUC) for the boosted classification tree algorithm (AI-BR) model and ACC-BR model were compared for the test cohort. Results. The mean age of the patient cohort was 67 +/- 12.7 years, and women constituted 30% of the cohort. The rate of major bleeding complications in the entire cohort was 1.8%. The sensitivity and specificity of the AI-BR model were 77.3% and 80.9%, respectively. The ROC-AUC for the AI-BR model (0.873) was superior vs the ACC-BR model (0.764; P=.02) in predicting major bleeding for the test cohort. Conclusion. The AI-BR model accurately predicts bleeding post PCI and outperforms the ACC-BR model in predicting the risk of bleeding in patients undergoing PCI.
更多查看译文
关键词
bleeding,percutaneous coronary Intervention,postprocedural complications,prediction model
AI 理解论文
溯源树
样例
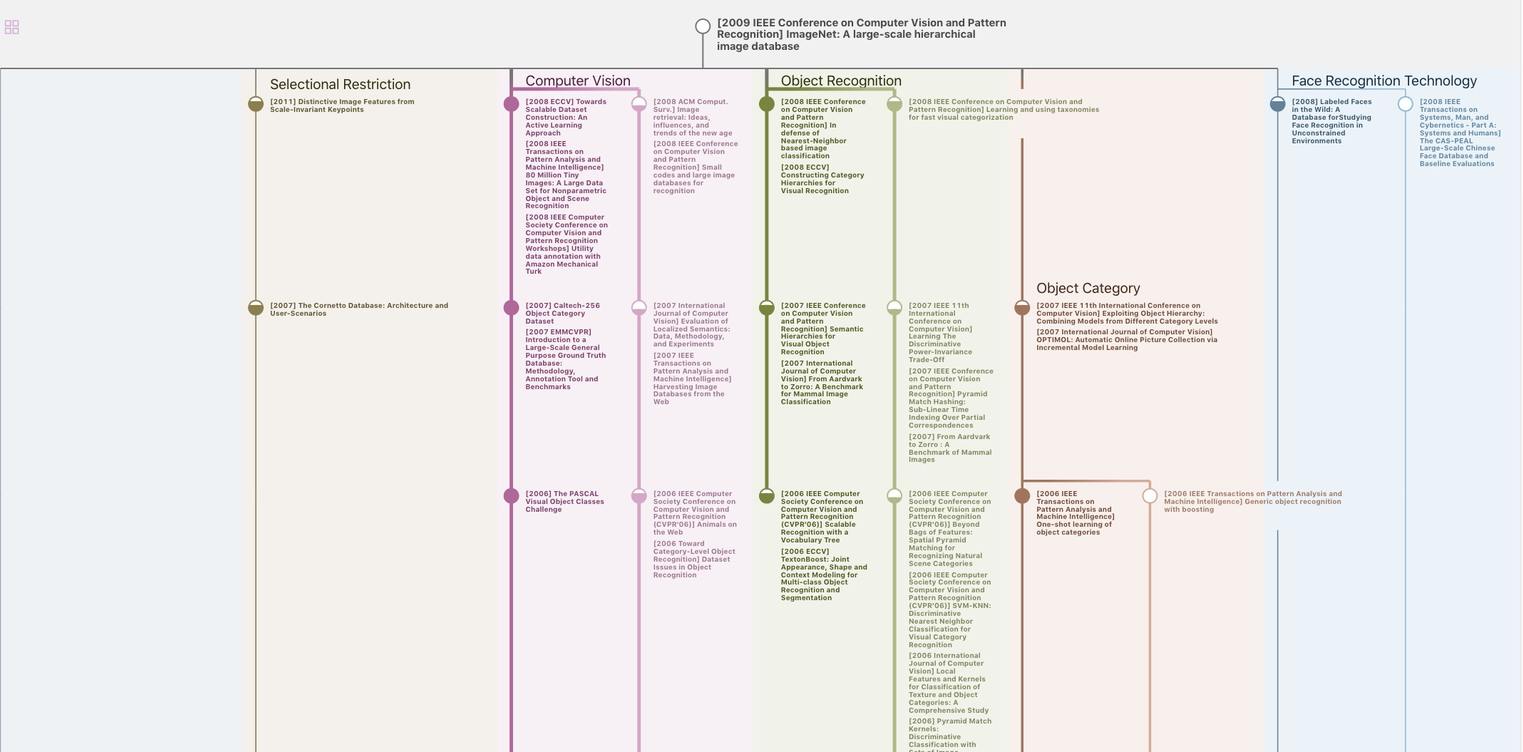
生成溯源树,研究论文发展脉络
Chat Paper
正在生成论文摘要