Support vector machine of wavelet packet spectral features for identification of obstructive sleep apnea
2018 5th International Conference on Electrical and Electronic Engineering (ICEEE)(2018)
摘要
Sleep apnea is a complete or partial cessation of breathing during sleep. Obstructive sleep apnea (OSA) is one of the most common breathing-related sleep disorders. The well-known reliable and standard diagnosis test used by specialized physicians is the polysomnographic sleep study. However, this test is complex and time consuming and expensive. Therefore, a non-invasive technique applying signal-processing algorithms is of more benefits for identification of OSA patients from normal subjects. Any identification algorithm has two parts: feature extraction part and feature matching part. In this paper, the feature extraction part depends on the wavelet-packet decomposition technique of the Heart Rate Variability (HRV) signal. The feature matching part uses the support vector machine (SVM). The highest performance on MIT standard data is achieved by the linear support vector machine with 5 stages wavelet decomposition using db1 filters with specificity, sensitivity, and accuracy of 100%, 90% and 93.34%, respectively.
更多查看译文
关键词
OSA,identification,HRV,wavelet packet decomposition,SVM
AI 理解论文
溯源树
样例
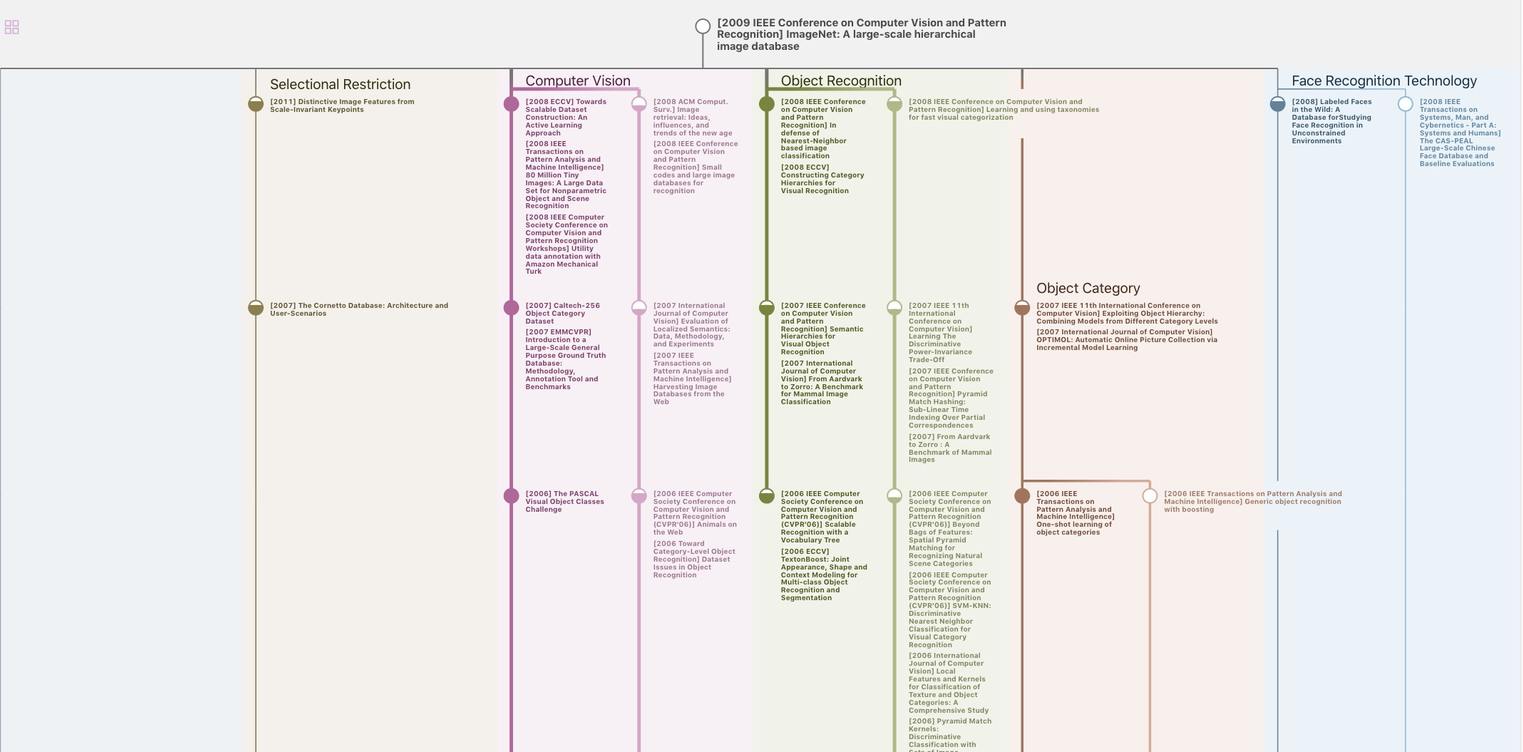
生成溯源树,研究论文发展脉络
Chat Paper
正在生成论文摘要