An Efficient Linearly Convergent Regularized Proximal Point Algorithm For Fused Multiple Graphical Lasso Problems
SIAM JOURNAL ON MATHEMATICS OF DATA SCIENCE(2021)
摘要
Nowadays, analyzing data from different classes or over a temporal grid has attracted a great deal of interest. As a result, various multiple graphical models for learning a collection of graphical models simultaneously have been derived by introducing sparsity in graphs and similarity across multiple graphs. This paper focuses on the fused multiple graphical Lasso model, which encourages not only shared pattern of sparsity but also shared values of edges across different graphs. For solving this model, we develop an efficient regularized proximal point algorithm, where the subproblem in each iteration of the algorithm is solved by a superlinearly convergent semismooth Newton method. To implement the semismooth Newton method, we derive an explicit expression for the generalized Jacobian of the proximal mapping of the fused multiple graphical Lasso regularizer. Unlike those widely used first order methods, our approach has heavily exploited the underlying second order information through the semismooth Newton method. This not only can accelerate the convergence of the algorithm but also can improve its robustness. The efficiency and robustness of our proposed algorithm are demonstrated by comparing it with some state-of-the-art methods on both synthetic and real data sets.
更多查看译文
关键词
fast linear convergence, network estimation, semismooth Newton method, sparse Jacobian
AI 理解论文
溯源树
样例
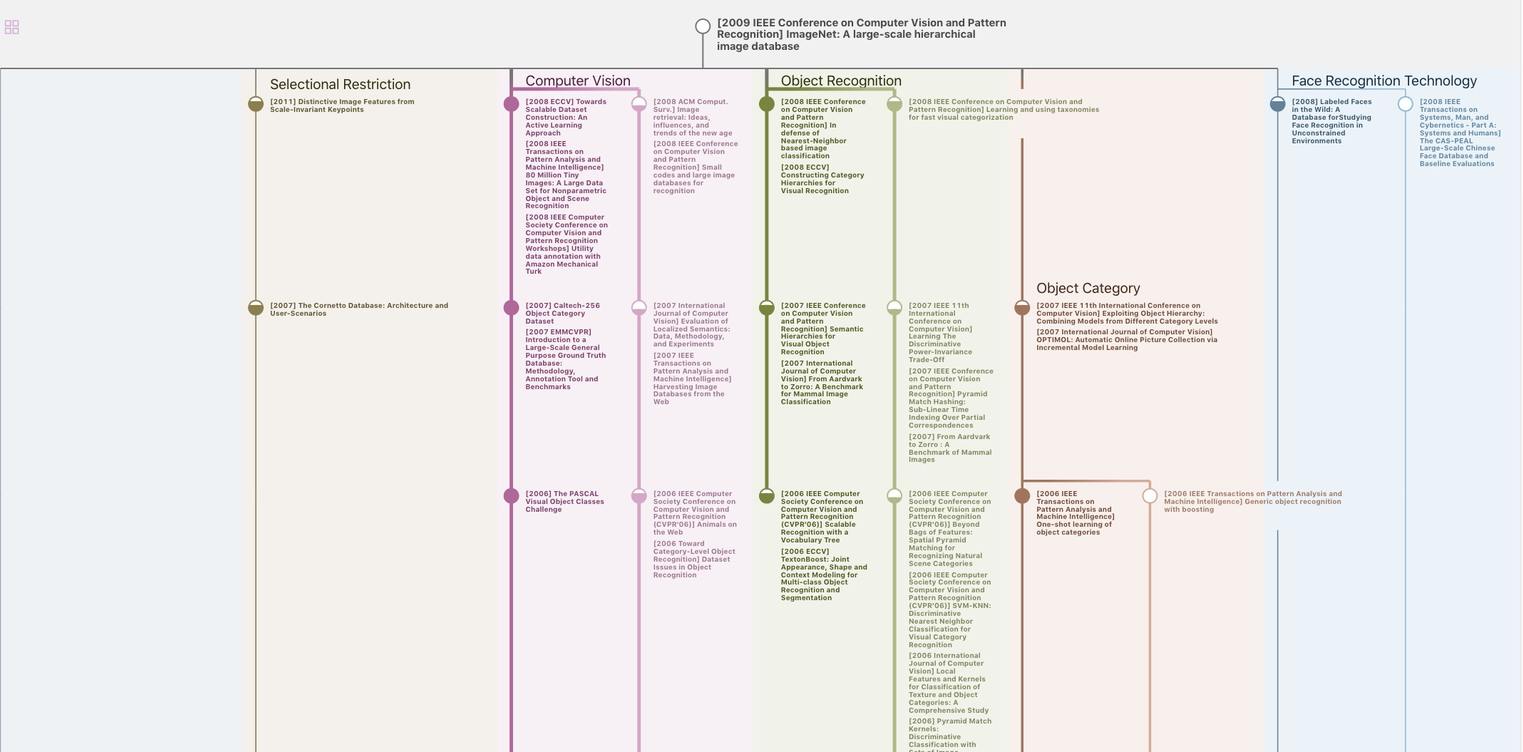
生成溯源树,研究论文发展脉络
Chat Paper
正在生成论文摘要