A parallel data generator for efficiently generating “realistic” social streams
Frontiers of Computer Science in China(2019)
摘要
A social stream refers to the data stream that records a series of social entities and the dynamic interactions between two entities. It can be employed to model the changes of entity states in numerous applications. The social streams, the combination of graph and streaming data, pose great challenge to efficient analytical query processing, and are key to better understanding users’ behavior. Considering of privacy and other related issues, a social stream generator is of great significance. A framework of synthetic social stream generator (SSG) is proposed in this paper. The generated social streams using SSG can be tuned to capture several kinds of fundamental social stream properties, including patterns about users’ behavior and graph patterns. Extensive empirical studies with several real-life social stream data sets show that SSG can produce data that better fit to real data. It is also confirmed that SSG can generate social stream data continuously with stable throughput and memory consumption. Furthermore, we propose a parallel implementation of SSG with the help of asynchronized parallel processing model and delayed update strategy. Our experiments verify that the throughput of the parallel implementation can increase linearly by increasing nodes.
更多查看译文
关键词
social stream, data generator, SSG, parallel generation
AI 理解论文
溯源树
样例
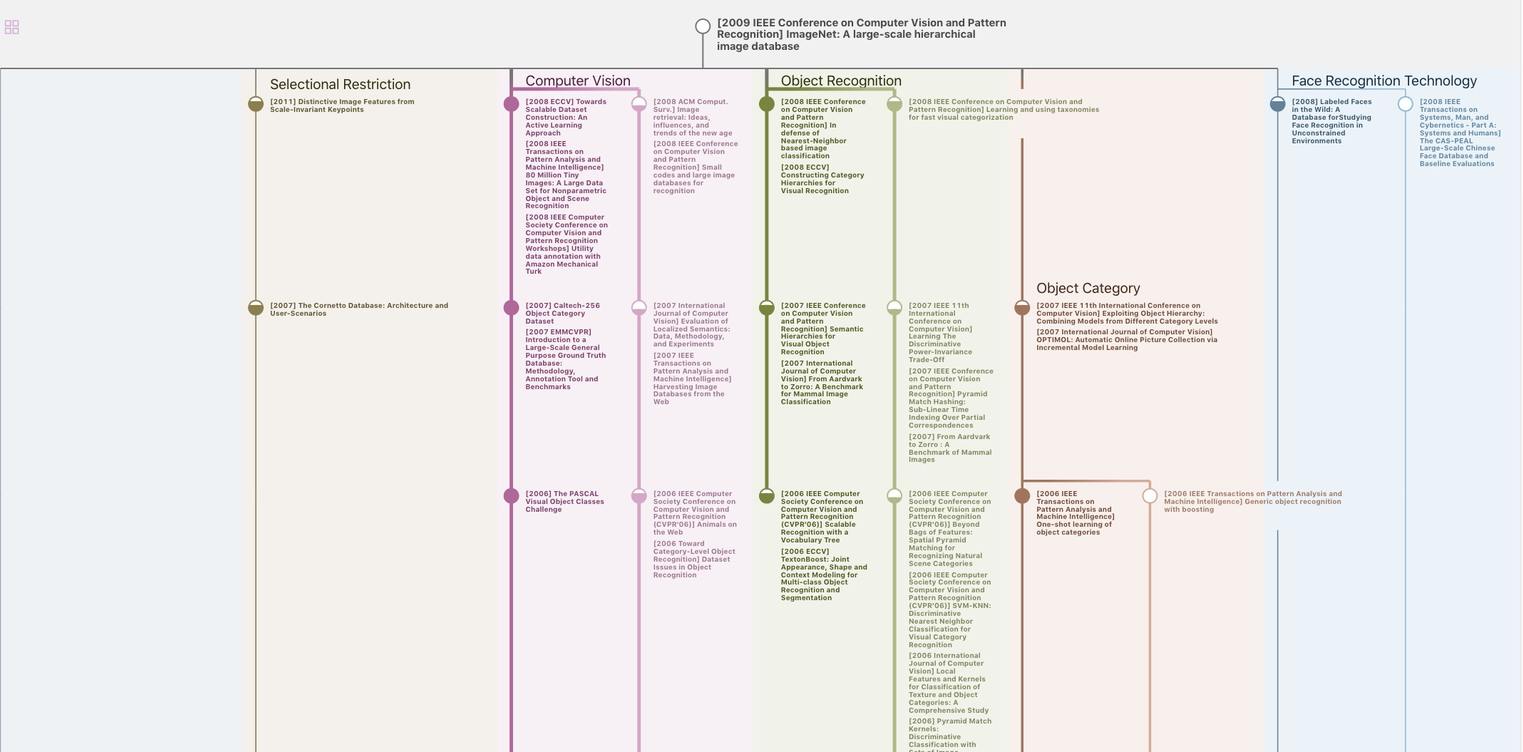
生成溯源树,研究论文发展脉络
Chat Paper
正在生成论文摘要