Prediction of condition-specific regulatory maps in Arabidopsis using integrated genomic data
bioRxiv(2019)
摘要
Recent advances in genomic technologies have generated large-scale protein-DNA interaction data and open chromatic regions for multiple plant species. To predict condition specific gene regulatory networks using these data, we developed the Condition Specific Regulatory network inference engine (ConSReg), which combines heterogeneous genomic data using sparse linear model followed by feature selection and stability selection to select key regulatory genes. Using Arabidopsis as a model system, we constructed maps of gene regulation under more than 50 experimental conditions including abiotic stresses, cell type-specific expression, and stress responses in individual cell types. Our results show that ConSReg accurately predicted gene expressions (average auROC of 0.84) across multiple testing datasets. We found that, (1) including open chromatin information from ATAC-seq data significantly improves the performance of ConSReg across all tested datasets; (2) choice of negative training samples and length of promoter regions are two key factors that affect model performance. We applied ConSReg to Arabidopsis single cell RNA-seq data of two root cell types (endodermis and cortex) and identified five regulators in two root cell types. Four out of the five regulators have additional experimental evidence to support their roles in regulating gene expression in Arabidopsis roots. By comparing regulatory maps in abiotic stress responses and cell type-specific experiments, we revealed that transcription factors that regulate tissue levels abiotic stresses tend to also regulate stress responses in individual cell types in plants.
更多查看译文
AI 理解论文
溯源树
样例
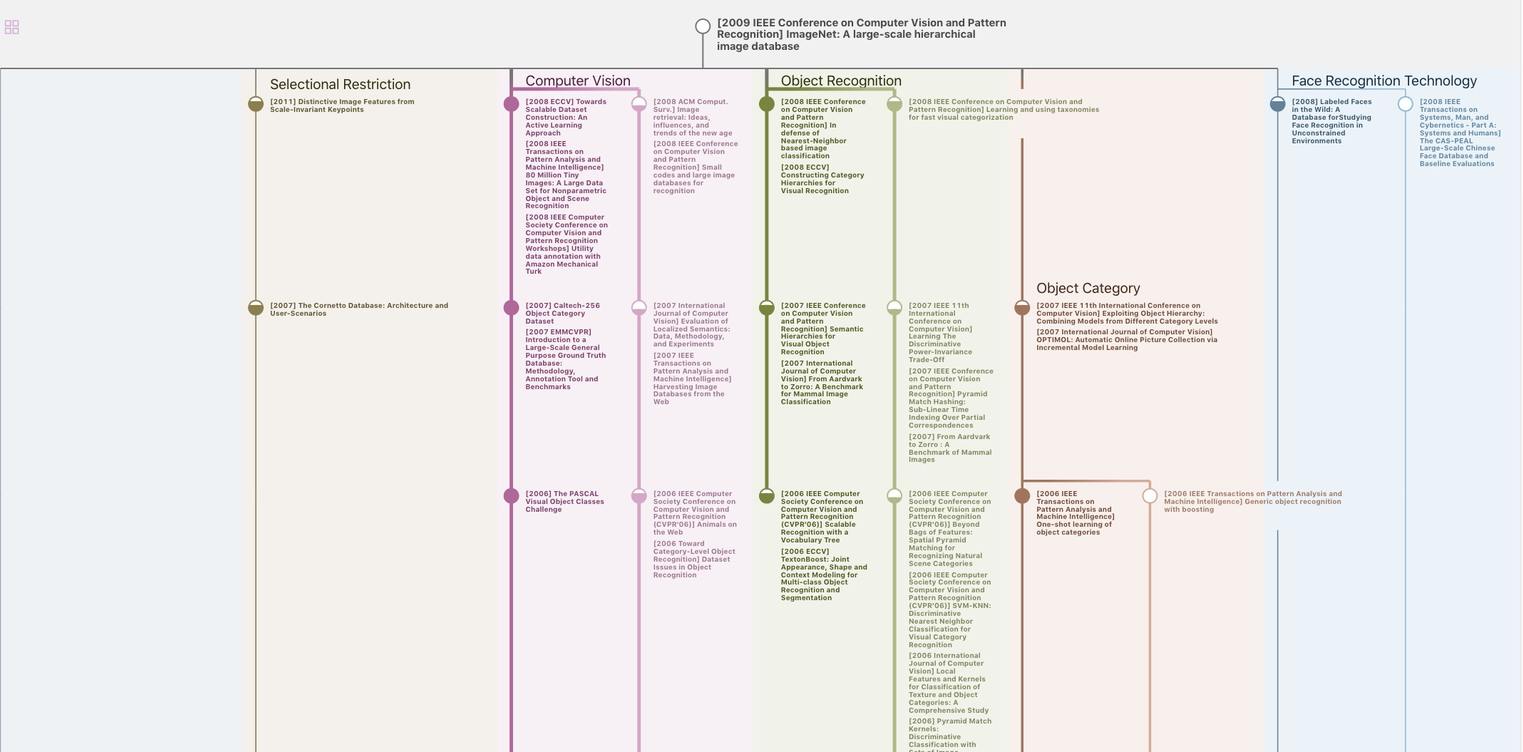
生成溯源树,研究论文发展脉络
Chat Paper
正在生成论文摘要