Label-free Method for Classification of T cell Activation
bioRxiv(2019)
摘要
T cells have a range of cytotoxic and immune-modulating functions, depending on activation state and subtype. However, current methods to assess T cell function use exogenous labels that often require cell permeabilization, which is limiting for time-course studies of T cell activation and non-destructive quality control of immunotherapies. Label-free optical imaging is an attractive solution. Here, we use autofluorescence imaging of NAD(P)H and FAD, co-enzymes of metabolism, to quantify optical imaging endpoints in quiescent and activated T cells. Machine learning classification models were developed for label-free, non-destructive determination of T cell activation state. T cells were isolated from the peripheral blood of human donors, and a subset were activated with a tetrameric antibody against CD2/CD3/CD28 surface ligands. NAD(P)H and FAD autofluorescence intensity and lifetime of the T cells were imaged using a multiphoton fluorescence lifetime microscope. Significant differences in autofluorescence imaging endpoints were observed between quiescent and activated T cells. Feature selection methods revealed that the contribution of the short NAD(P)H lifetime (α 1 ) is the most important feature for classification of activation state, across multiple donors and T cell subsets. Logistic regression models achieved 97-99% accuracy for classification of T cell activation from the autofluorescence imaging endpoints. Additionally, autofluorescence imaging revealed NAD(P)H and FAD autofluorescence differences between CD3 + CD8 + and CD3 + CD4 + T cells, and random forest models of the autofluorescence imaging endpoints achieved 97+% accuracy for four-group classification of quiescent and activated CD3 + CD8 + and CD3 + CD4 + T cells. Altogether these results indicate that autofluorescence imaging of NAD(P)H and FAD is a powerful method for label-free, non-destructive determination of T cell activation and subtype, which could have important applications for the treatment of cancer, autoimmune, infectious, and other diseases.
更多查看译文
AI 理解论文
溯源树
样例
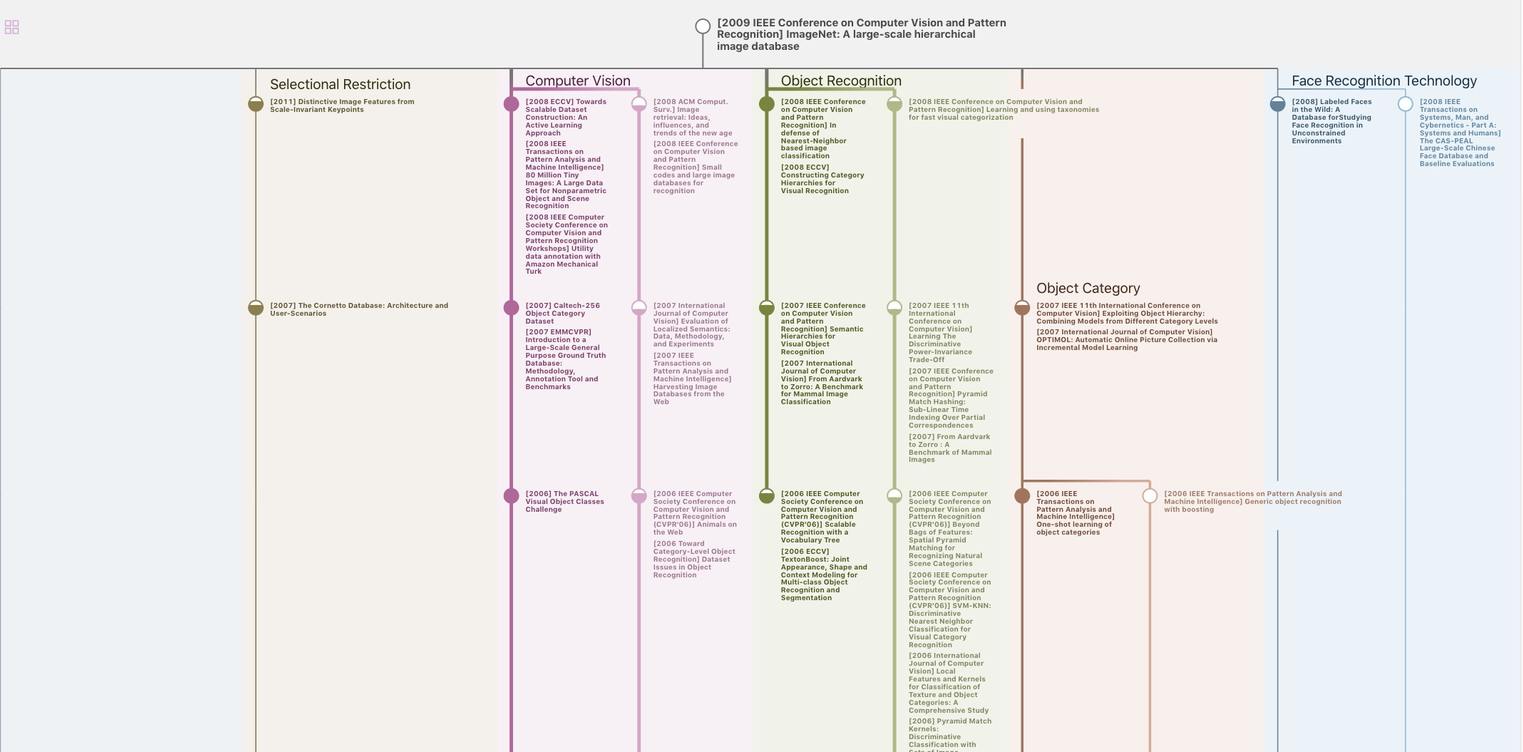
生成溯源树,研究论文发展脉络
Chat Paper
正在生成论文摘要