Fuzzy Support Vector Machine with Relative Density Information for Classifying Imbalanced Data
IEEE Transactions on Fuzzy Systems(2019)
摘要
Fuzzy support vector machine (FSVM) has been combined with class imbalance learning (CIL) strategies to address the problem of classifying skewed data. However, the existing approaches hold several inherent drawbacks, causing the inaccurate prior data distribution estimation, further decreasing the quality of the classification model. To solve this problem, we present a more robust prior data distribution information extraction method named relative density, and two novel FSVM-CIL algorithms based on the relative density information in this paper. In our proposed algorithms, a K-nearest neighbors-based probability density estimation (KNN-PDE) alike strategy is utilized to calculate the relative density of each training instance. In particular, the relative density is irrelevant with the dimensionality of data distribution in feature space, but only reflects the significance of each instance within its class; hence, it is more robust than the absolute distance information. In addition, the relative density can better seize the prior data distribution information, no matter the data distribution is easy or complex. Even for the data with small injunctions or a large class overlap, the relative density information can reflect its details well. We evaluated the proposed algorithms on an amount of synthetic and real-world imbalanced datasets. The results show that our proposed algorithms obviously outperform to some previous work, especially on those datasets with sophisticated distributions.
更多查看译文
关键词
Training,Classification algorithms,Estimation,Sun,Support vector machine classification,Density measurement
AI 理解论文
溯源树
样例
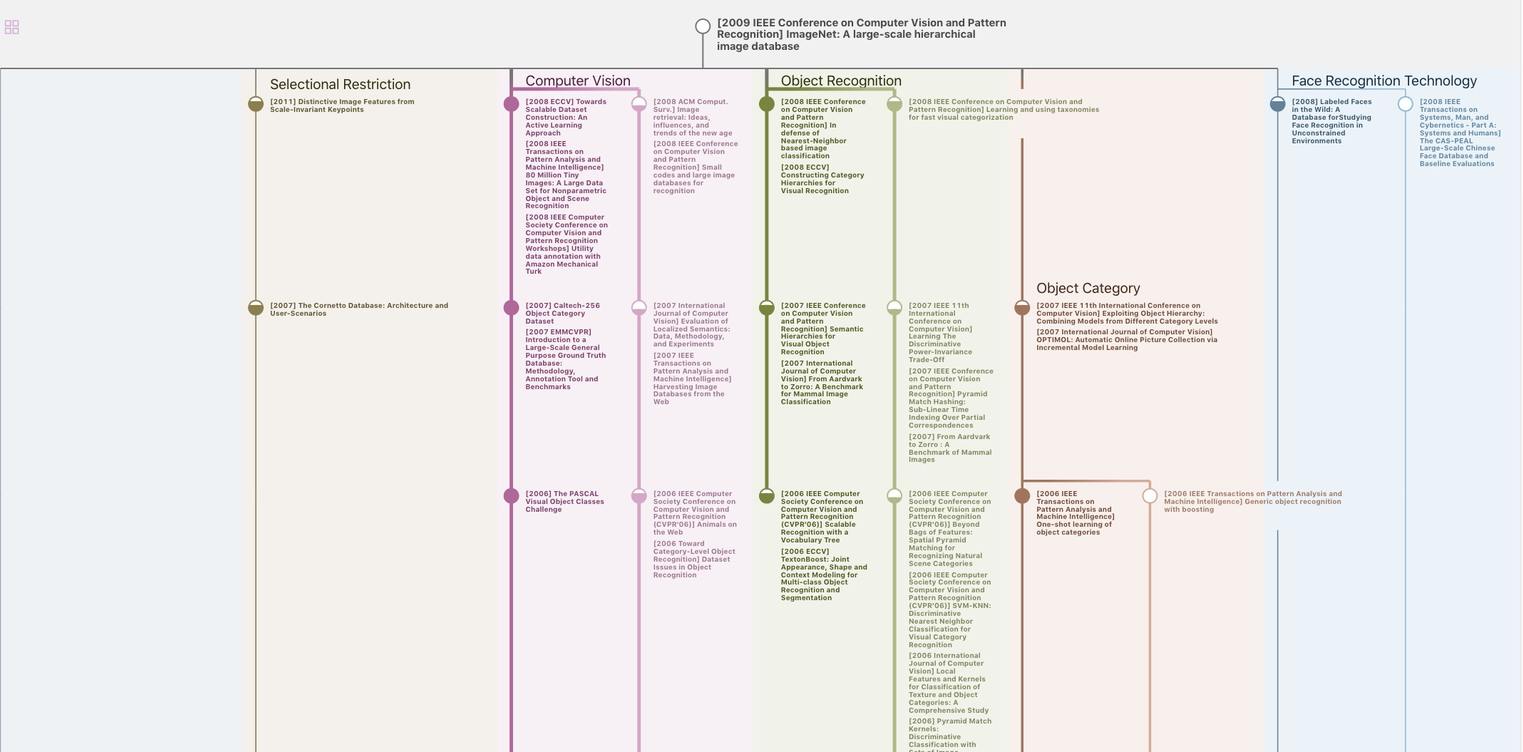
生成溯源树,研究论文发展脉络
Chat Paper
正在生成论文摘要