Diagnostic measures for kernel ridge regression on reproducing kernel Hilbert space
Journal of the Korean Statistical Society(2019)
摘要
The aim of this paper is to define and develop diagnostic measures with respect to kernel ridge regression in a reproducing kernel Hilbert space (RKHS). To identify influential observations, we define a particular version of Cook’s distance for the kernel ridge regression model in RKHS, which is conceptually consistent with Cook’s distance in a classical regression model. Then, by using the perturbation formula for the regularized conditional expectation of the outcome in RKHS, we develop an approximate version of Cook’’s distance in RKHS because the original definition requires intensive computations. Such anapproximated Cook’’s distance is represented in terms of basic building blocks such as residuals and leverages of the kernel ridge regression. The results of the simulation and real application demonstrate that our diagnostic measure successfully detects potentially influential observations on estimators in kernel ridge regression.
更多查看译文
关键词
Bootstrap,Variance operator,Empirical minimization,Reproducing kernel,Singular value decomposition
AI 理解论文
溯源树
样例
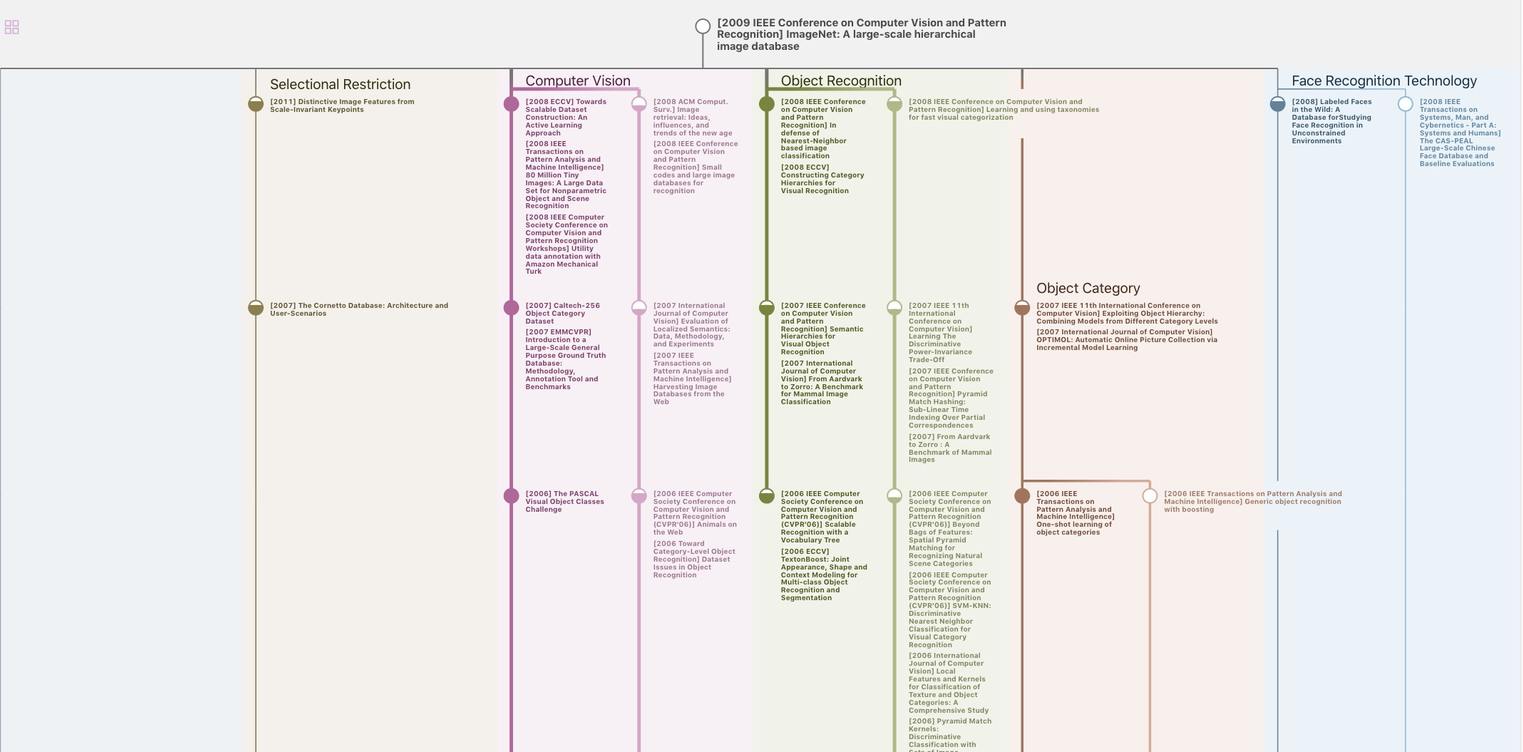
生成溯源树,研究论文发展脉络
Chat Paper
正在生成论文摘要