Do Features From Short Durational Segments Classify Epileptic EEG Signals Effectively?
2018 IEEE Region 10 Humanitarian Technology Conference (R10-HTC)(2018)
摘要
Automated analysis of electroencephalogram (EEG) signal is the current trend in epilepsy diagnosis. Machine learning based automated analysis enables the fast and accurate detection of epileptic diseases. Recent studies have concentrated on developing efficient algorithms for the EEG analysis and diagnosis of epilepsy. A major bottleneck during the algorithm development is that, whether to consider a lengthy signal to analyze or a part of the signal for producing an adequate classification result. In this work, we analyzed the epileptic classification performance of the features from short durational segment of the signal. Two well-known entropy features are extracted from the random segments. The results of a benchmarking epilepsy EEG data set shows that features from short durational segments promisingly classify the epilepsy signals.
更多查看译文
关键词
EEG,epilepsy,short durational segments,approximate entropy,sample entropy,classification
AI 理解论文
溯源树
样例
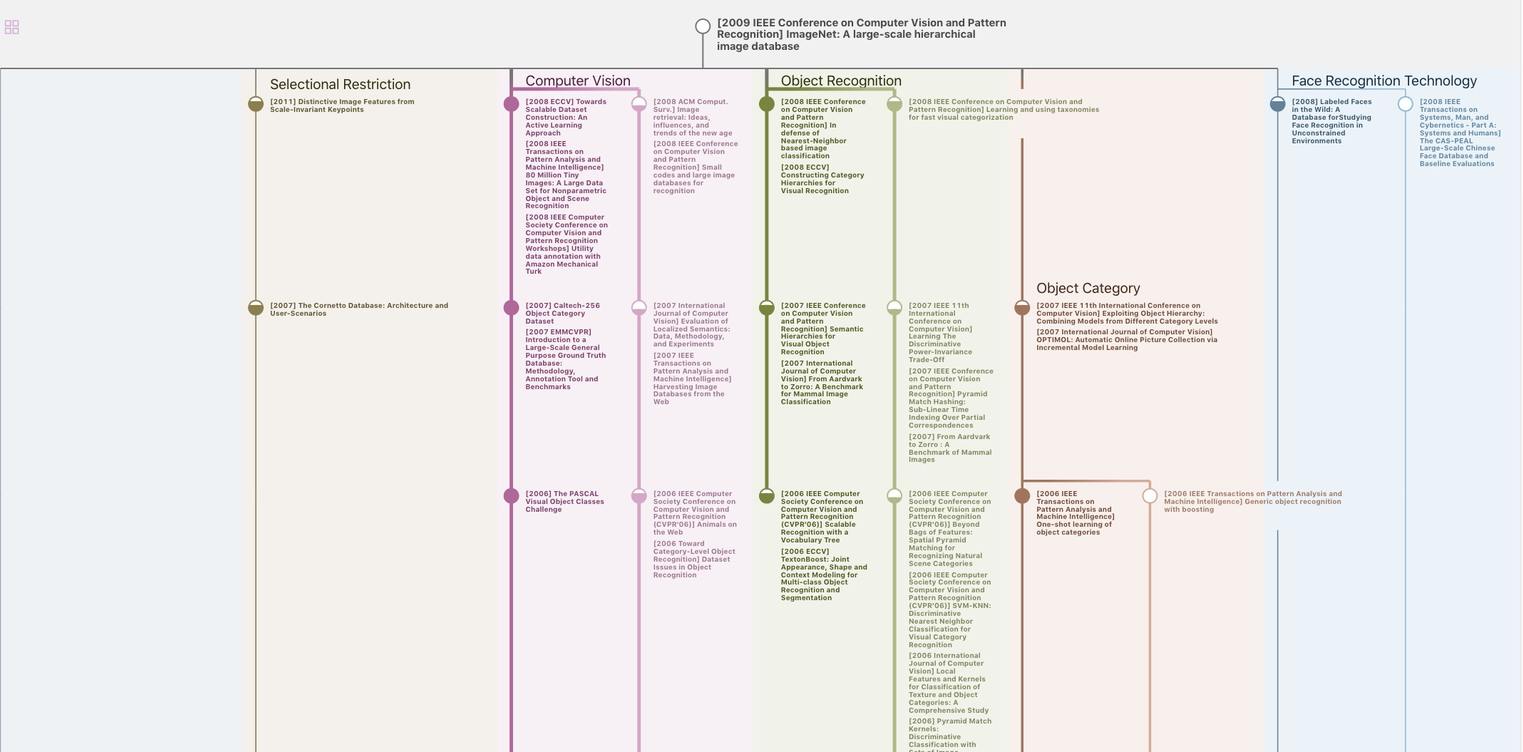
生成溯源树,研究论文发展脉络
Chat Paper
正在生成论文摘要