Comparison Of Human And Machine Learning Based Facial Weakness Detection
Stroke(2019)
摘要
Background: Prehospital stroke screening tools are essential to rapid diagnosis and treatment, but variable recognition of common stroke deficits by EMS providers limits the accuracy and reliability of these tools. An automated screening tool could yield more consistent assessments and reduce operator variability. We hypothesized that a video-based, machine learning algorithm can detect facial weakness with comparable accuracy to EMS providers. Methods: Videos with normal smile and facial weakness were collected from open access, online repositories. Two senior neurology residents independently rated videos using a 5-point scale to denote the likely presence or absence of pathological facial weakness. Only videos rated concurrently by both raters were included for training and testing of the algorithm. Facial landmarks were extracted per frame using an open-source face-detector and landmark detector followed by normalization of landmarks and intensity values. Optical-flow based features and facial landmark based configural features were calculated for each video and classified with a logistic regression model. Accuracy was calculated using a 5-fold cross validation scheme. To interpret algorithm performance, we analyzed ratings of three EMS providers against the resident ratings. Results: Of 160 videos with concordant ratings, 24 were excluded due to issues with muscle activation or head orientation, resulting in 31, 36, and 69 videos with left, right, and no facial weakness, respectively. Mean accuracy for the algorithm was 84.6% (95% Cl [74.3-94.9]) compared with 89.0% (95% Cl [86.0-92.0]) for EMS providers. More severe facial weakness compromised the performance of the landmark detector, limiting detection to optical flow analysis alone. Conclusion: Our study demonstrates that a learning algorithm can detect facial weakness in videos, however, the algorithm was not as accurate as EMS. Videos with more pronounced weakness presented a challenge for the algorithm whereas human examiners can more easily identify weakness in these cases. Future research will include refining the algorithm for severe facial weakness and expanding to detect other stroke deficits such as limb weakness and ataxia.
更多查看译文
AI 理解论文
溯源树
样例
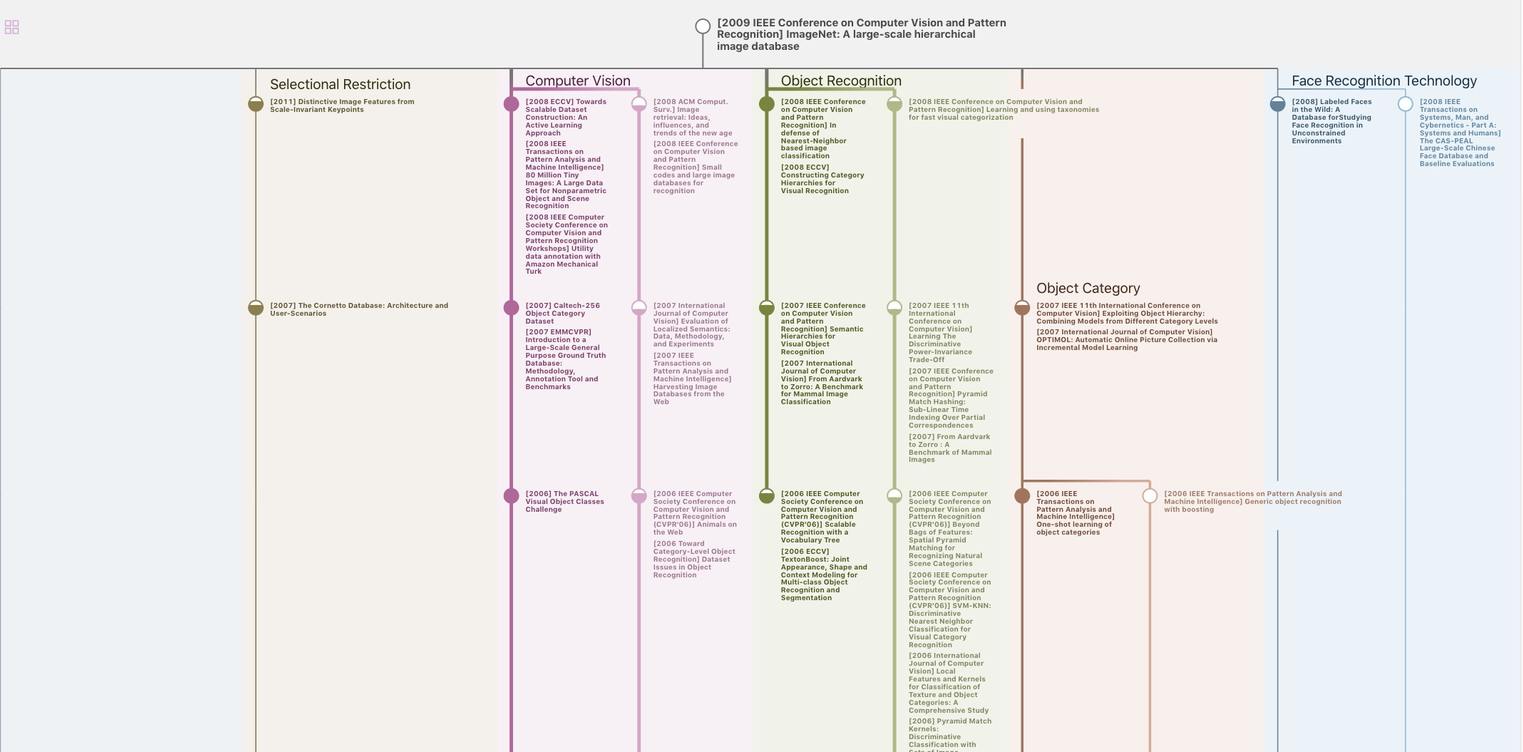
生成溯源树,研究论文发展脉络
Chat Paper
正在生成论文摘要