Exploiting label semantic relatedness for unsupervised image annotation with large free vocabularies
Multimedia Tools and Applications(2019)
摘要
Automatic Image Annotation (AIA) is the task of assigning keywords to images, with the aim to describe their visual content. Recently, an unsupervised approach has been used to tackle this task. Unsupervised AIA (UAIA) methods use reference collections that consist of the textual documents containing images. The aim of the UAIA methods is to extract words from the reference collection to be assigned to images. In this regard, by using an unsupervised approach it is possible to include large vocabularies because any word could be extracted from the reference collection. However, having a greater diversity of words for labeling entails to deal with a larger number of wrong annotations, due to the increasing difficulty for assigning a correct relevance to the labels. With this problem in mind, this paper presents a general strategy for UAIA methods that reranks assigned labels. The proposed method exploits the semantic-relatedness information among labels in order to assign them an appropriate relevance for describing images. Experimental results in different benchmark datasets show the flexibility of our method to deal with assignments from free-vocabularies, and its effectiveness to improve the initial annotation performance for different UAIA methods. Moreover, we found that (1) when considering the semantic-relatedness information among the assigned labels, the initial ranking provided by a UAIA method is improved in most of the cases; and (2) the robustness of the proposed method to be applied on different UAIA methods, will allow extending capabilities of state-of-the-art UAIA methods.
更多查看译文
关键词
Unsupervised image annotation,Label relevance,Semantic-relatedness estimation
AI 理解论文
溯源树
样例
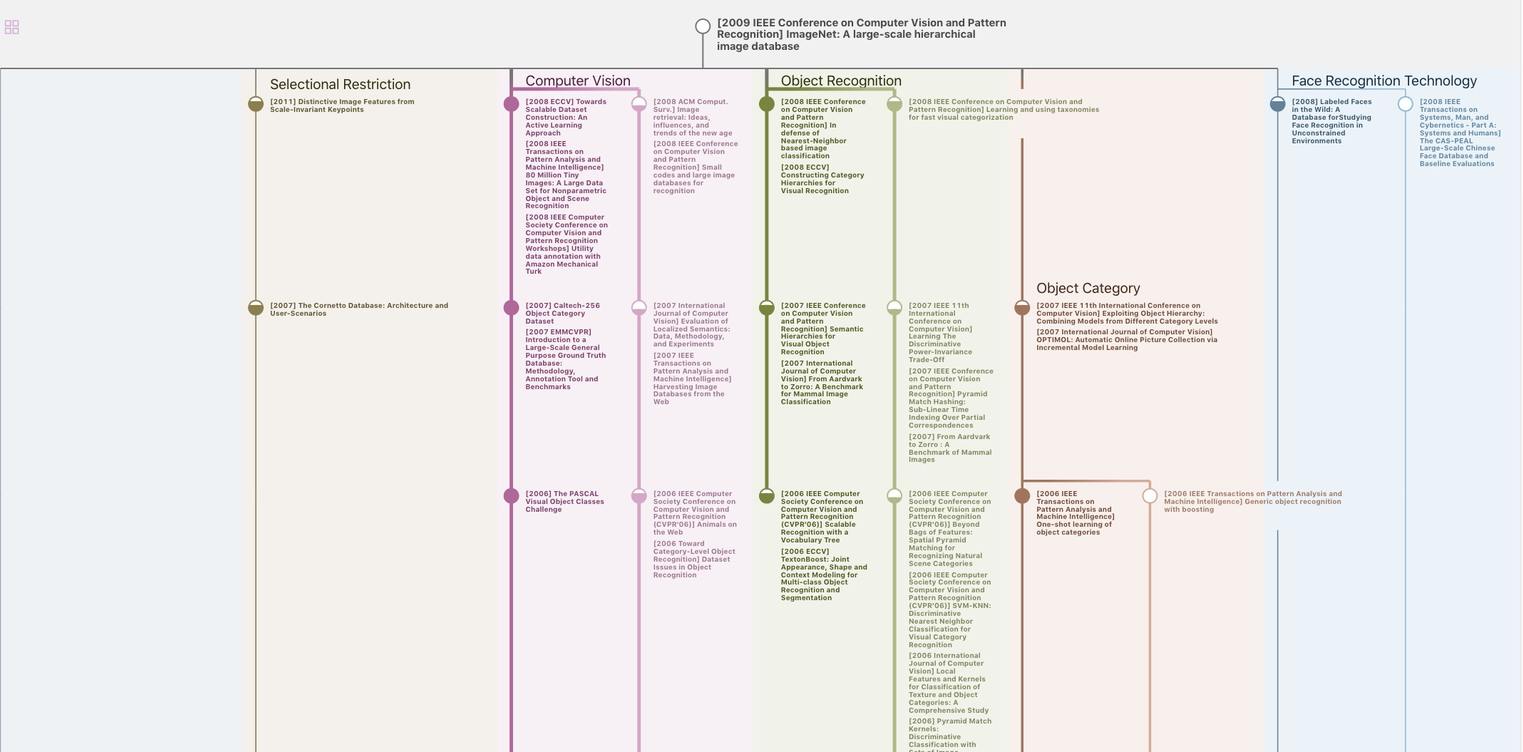
生成溯源树,研究论文发展脉络
Chat Paper
正在生成论文摘要