Comparative Study Of Decision Tree With Different Evidential Uncertainty Measures
2018 CHINESE AUTOMATION CONGRESS (CAC)(2018)
摘要
The Decision tree is an effective classifier. In the decision tree, different levels of uncertainty may have to be handled. The theory of belief function is a useful tool to deal with uncertainty. There has been proposed several decision trees based on the theory of belief functions and one of these decision trees used a composite uncertainty measure as the criterion of selecting the appropriate attribute in the process of splitting the node. However, there have been various kinds of uncertainty measures in the theory of belief function, and the existing uncertainty measures may have different influence on the result of classification. In this paper, we use different uncertainty measures in the decision tree based on the theory of belief function to select the appropriate attribute. We provide comparative results and related analyses to check the impact of the uncertainty measure selection on the classification performance of the decision tree.
更多查看译文
关键词
decision trees, classification, belief functions, evidence theory, uncertainty measure
AI 理解论文
溯源树
样例
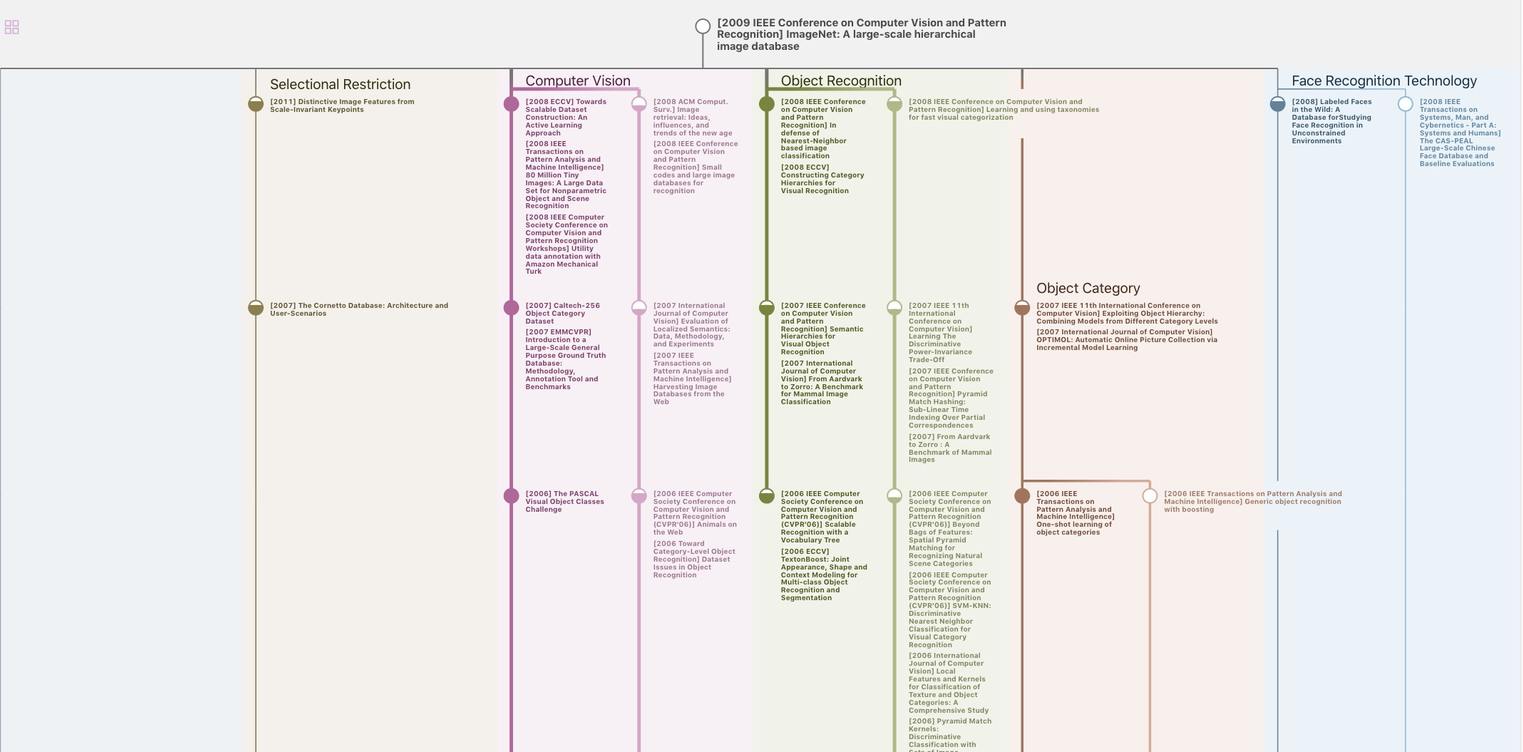
生成溯源树,研究论文发展脉络
Chat Paper
正在生成论文摘要