Traffic Scene Classification on a Representation Budget
IEEE Transactions on Intelligent Transportation Systems(2020)
摘要
Visual cues can be used alongside GPS positioning and digital maps to improve understanding of vehicle environment in fleet management systems. Such systems are limited both in terms of bandwidth and storage space, so minimizing the size of transmitted and stored visual data is a priority. In this paper, we present efficient strategies for computing very short image representations suitable for classifying various types of traffic scenes in fleet management systems. We anticipate that the set of interesting classes will change over time, so we consider image representations that can be trained without knowing the labels of the target dataset. We empirically evaluate and compare the presented methods on a contributed dataset of 11447 labeled traffic scenes. Our results indicate that excellent classification results can be achieved with very short image representations, and that fine-tuning on the target dataset image data is not mandatory. Image descriptors can be as short as 128 components while still offering good performance, even in presence of adverse weather or illumination conditions.
更多查看译文
关键词
Visualization,Training,Feature extraction,Image representation,Servers,Global Positioning System,Architecture
AI 理解论文
溯源树
样例
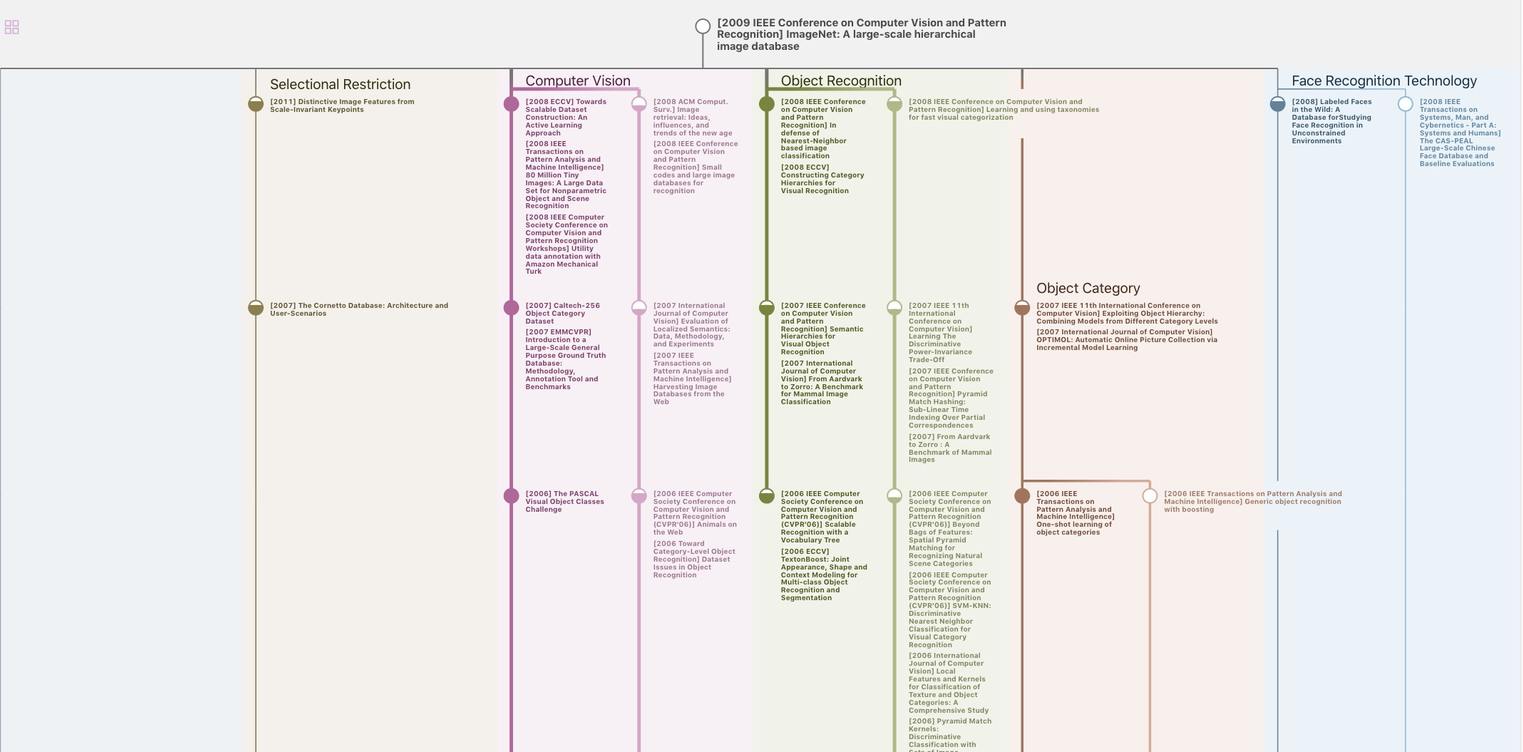
生成溯源树,研究论文发展脉络
Chat Paper
正在生成论文摘要