An adaptive sampling strategy for quasi real time crack characterization on eddy current testing signals
NDT & E International(2019)
摘要
This work describes quasi real time flaw(s) characterization in conductive plate(s) through the inversion of eddy current testing (ECT) signals using learning by examples (LBE) paradigm. Within the framework of LBE, a fast and accurate learning model is fitted on an optimal training set based on simulated eddy current testing data and the corresponding set of parameters during a preliminary offline phase. More specially, the optimal training set has been generated in the offline phase by adopting an adaptive sampling strategy through exploiting Partial Least Squares (PLS) feature extraction and output space filling (OSF). Subsequently, a non linear model is fitted on the training set and used to predict the set of parameters associated to an unknown (possibly large) test set during the so-called online phase. Different models, i.e., learning algorithms, such as Support Vector Regression (SVR), Kernel Ridge Regression (KRR), Relevance Vector Regression (RVR) and Augmented Radial Basis Function (A-RBF) have been adopted in order to build different accurate predictors. Afterwards, quasi real-time inversion has been performed on unknown test set by utilizing the corresponding trained models. Comparative results are reported through numerical and experimental data sets to assess the inversion performance of different learning algorithms based on the PLS-OSF sampling strategy.
更多查看译文
关键词
Eddy current testing,Real time inversion,Learning by examples,Partial least squares,Output-space-filling,Support vector regression,Kernel ridge regression,Relevance vector regression,Augmented radial basis function
AI 理解论文
溯源树
样例
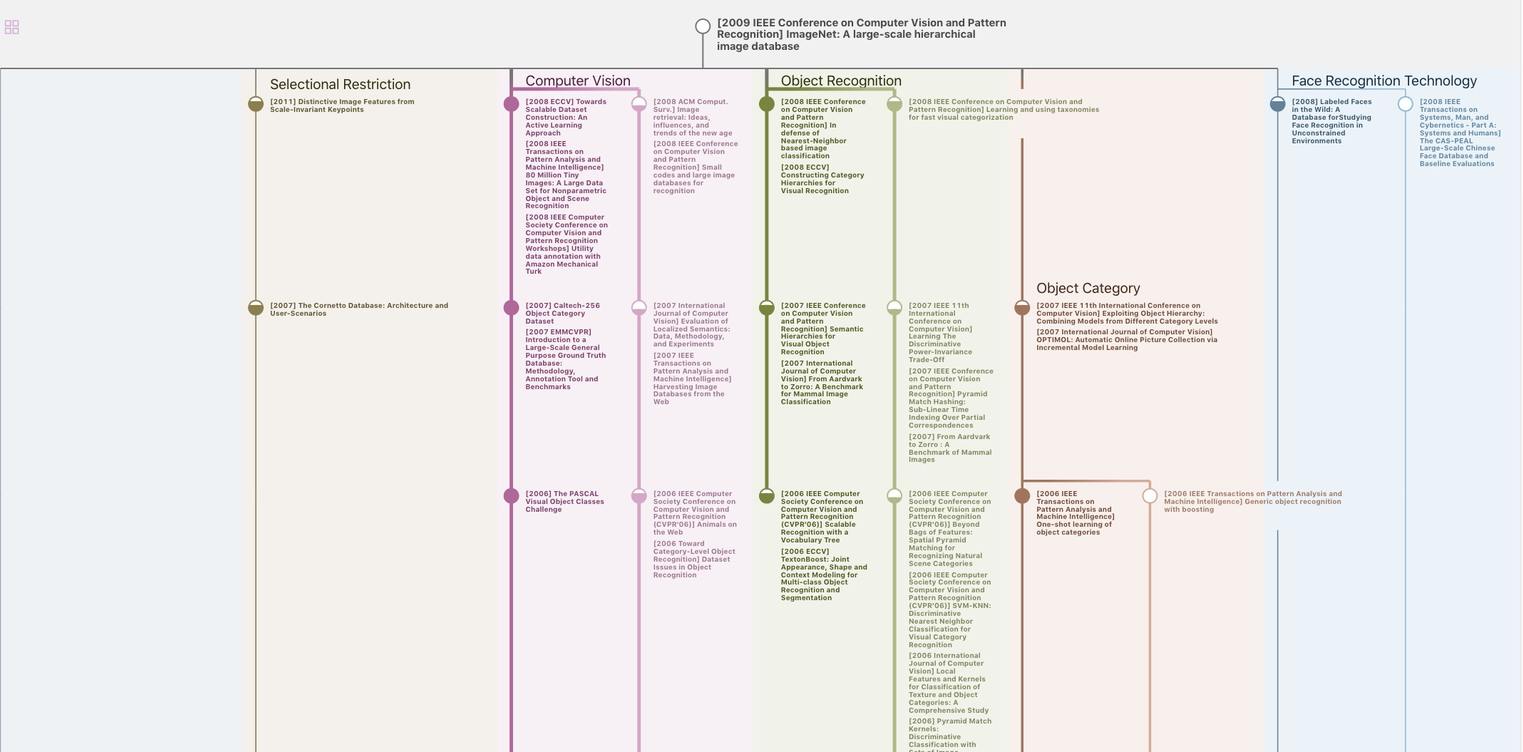
生成溯源树,研究论文发展脉络
Chat Paper
正在生成论文摘要