Reward Function Evaluation in a Reinforcement Learning Approach for Energy Management
2018 16th Biennial Baltic Electronics Conference (BEC)(2018)
摘要
In the past decade, the energy needs in WBANs have increased due to more information to be processed, more data to be transmitted and longer operational periods. On the other hand, battery technologies have not improved fast enough to cope with these needs. Thus, miniaturized energy harvesting technologies are increasingly used to complement the batteries in WBANs. However, this brings uncertainties in the system since the harvested energy varies a lot during the node operation. It has been shown that reinforcement learning algorithms can be used to manage the energy in the nodes since they are able to make decisions under uncertainty. But the efficiency of these algorithms depends on their reward function. In this paper we explore different reward functions and seek to identify the most suitable variables to use in such functions to obtain the expected behavior. Experimental results with four different reward functions illustrate how the choice thereof impacts the energy consumption of the nodes.
更多查看译文
关键词
reinforcement learning,WBAN,reward function,Q-learning,energy management
AI 理解论文
溯源树
样例
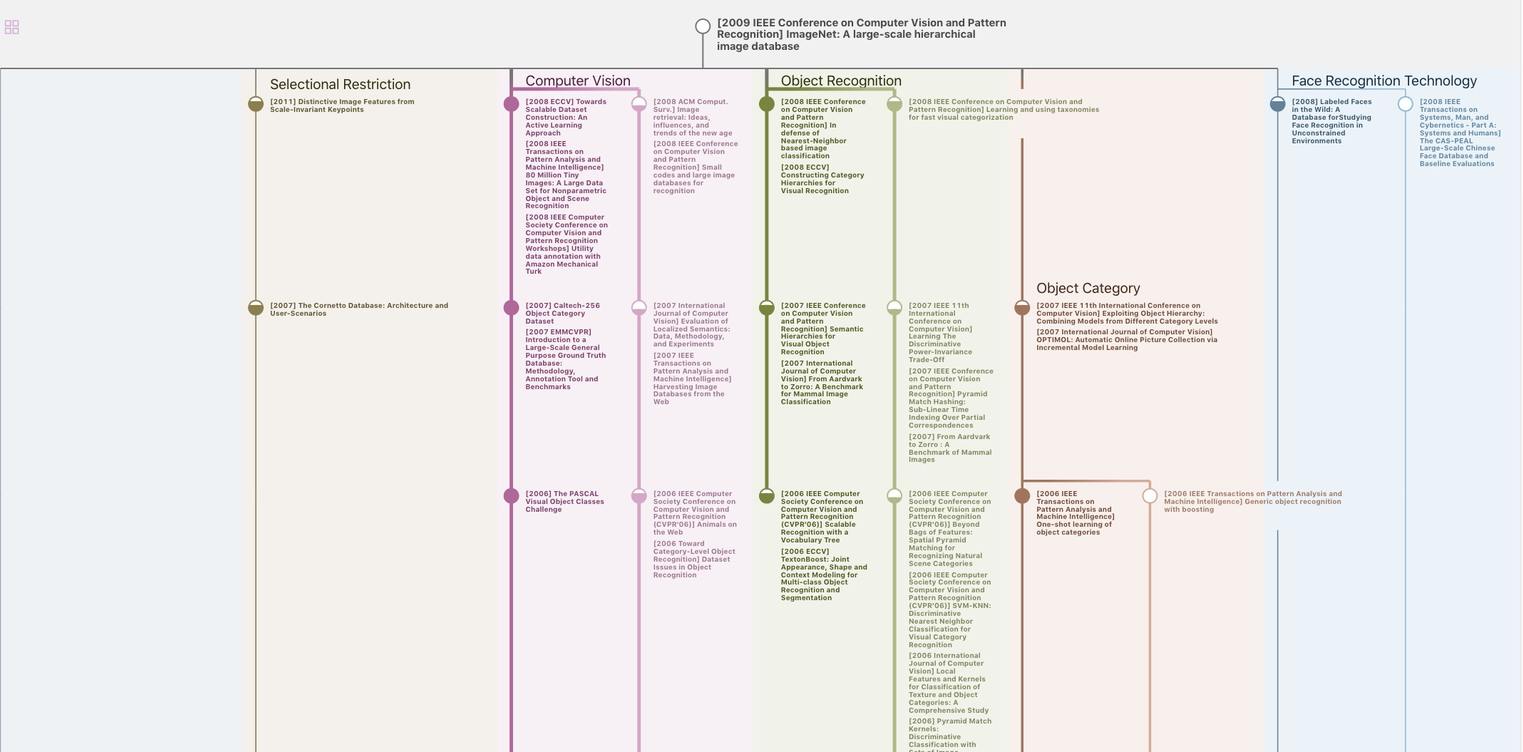
生成溯源树,研究论文发展脉络
Chat Paper
正在生成论文摘要