The Suppression Of Transient Artifacts In Time Series Via Convex Analysis
2018 IEEE SIGNAL PROCESSING IN MEDICINE AND BIOLOGY SYMPOSIUM (SPMB)(2018)
摘要
For the suppression of transient artifacts in time series data, we propose a non-convex generalized fused lasso penalty for the estimation of signals comprising a low-pass signal, a sparse piecewise constant signal, and additive white Gaussian noise. The proposed non-convex penalty is designed so as to preserve the convexity of the total cost function to be minimized, thereby realizing the benefits of a convex optimization framework (reliable, robust algorithms, etc.). Compared to the conventional use of L1 norm penalty, the proposed non-convex penalty does not underestimate the true amplitude of signal values. We derive a fast proximal algorithm to implement the method. The proposed method is demonstrated on the suppression of artifacts in near infrared spectroscopic (NIRS) measures.
更多查看译文
关键词
convex analysis,transient artifacts,time series data,lasso penalty,low-pass signal,sparse piecewise constant signal,additive white Gaussian noise,nonconvex penalty,total cost function,convex optimization framework,L1 norm penalty,signal values,near infrared spectroscopic measures,NIRS
AI 理解论文
溯源树
样例
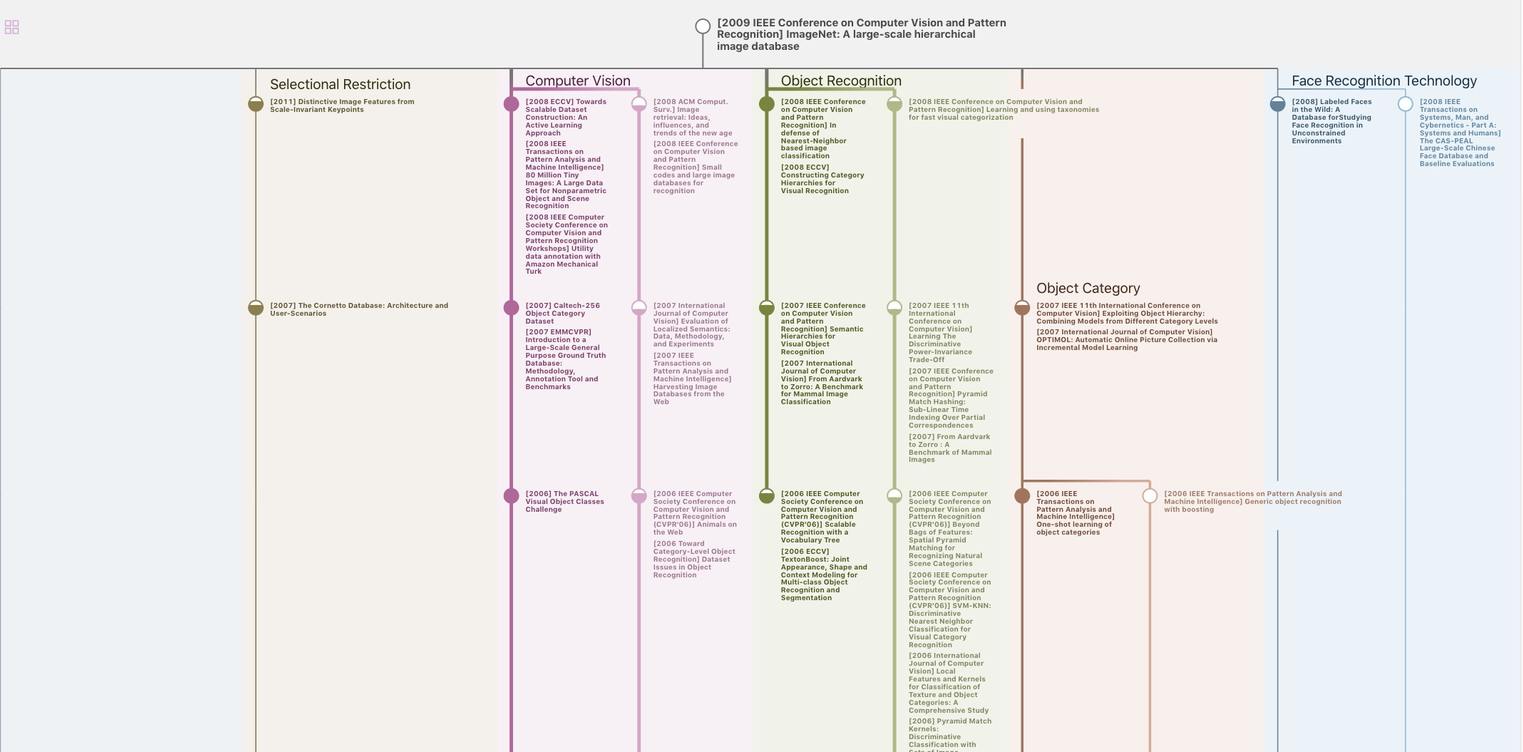
生成溯源树,研究论文发展脉络
Chat Paper
正在生成论文摘要