A Method Using EEMD and L-Kurtosis to Detect Faults in Roller Bearings
2018 Prognostics and System Health Management Conference (PHM-Chongqing)(2018)
摘要
The presence of periodical impulses in vibration signals usually indicates the occurrence of faults in roller bearings. Unfortunately, in the complex working condition with the heavy noises, fault detection in mechanical systems is often difficult. To solve this problem, a hybrid method of ensemble empirical mode decomposition (EEMD) and L-Kurtosis clustering-based segmentation is proposed. EEMD is similar to empirical mode decomposition (EMD), which can express the intrinsic essence using simple and understandable algorithm to solve the mode mixing phenomenon. L-Kurtosis is the improved version of kurtosis to recognize the impulses without the influence of outliers. Furthermore, the L-Kurtosis value is employed as an indicator in the clustering-based segmentation method to extract the fault features from the background noises. To illustrate the feasibility of utilizing the EEMD and L-Kurtosis based clustering segmentation method, benchmark data simulations and experimental investigations are performed to detect faults in bearings. The results show that the proposed method enables the efficient recognition of faults in bearings.
更多查看译文
关键词
ensemble empirical mode decomposition,L-Kurtosis,clustering-based segmentation,fault detection,roller bearings
AI 理解论文
溯源树
样例
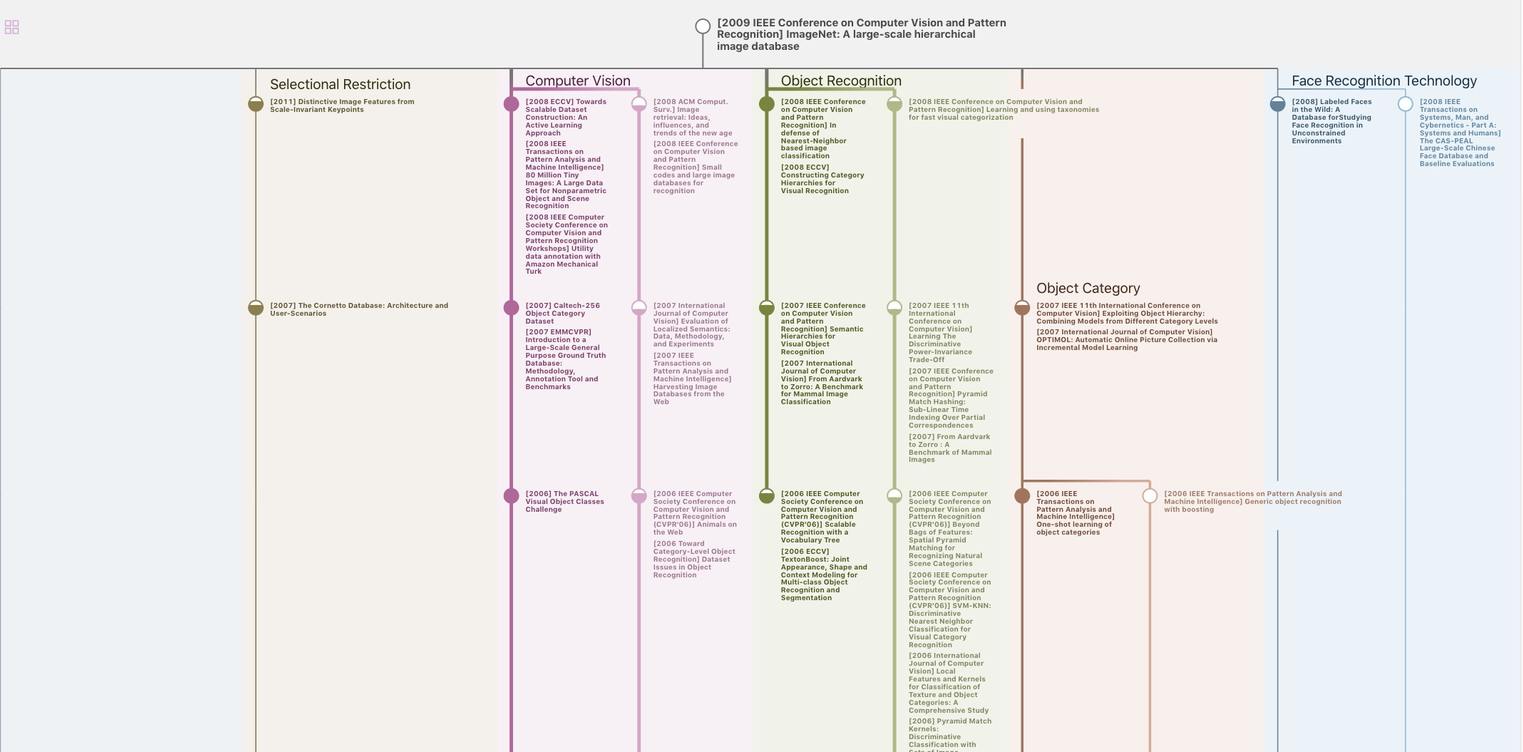
生成溯源树,研究论文发展脉络
Chat Paper
正在生成论文摘要