A Novel Discrete Particle Swarm Optimization Algorithm For Solving Bayesian Network Structures Learning Problem
INTERNATIONAL JOURNAL OF COMPUTER MATHEMATICS(2019)
摘要
Bayesian network is an effective representation tool to describe the uncertainty of the knowledge in artificial intelligence. One important method to learning Bayesian network from data is to employ a search procedure to explore the space of networks and a scoring metric to evaluate each candidate structure. In this paper, a novel discrete particle swarm optimization algorithm has been designed to solve the problem of Bayesian network structures learning. The proposed algorithm not only maintains the search advantages of the classical particle swarm optimization but also matches the characteristics of Bayesian networks. Meanwhile, mutation and neighbor searching operators have been used to overcome the drawback of premature convergence and balance the exploration and exploitation abilities of the particle swarm optimization. The experimental results on benchmark networks illustrate the feasibility and effectiveness of the proposed algorithm, and the comparative experiments indicate that our algorithm is highly competitive compared to other algorithms.
更多查看译文
关键词
Bayesian networks, structure learning, particle swarm optimization, heuristic algorithm
AI 理解论文
溯源树
样例
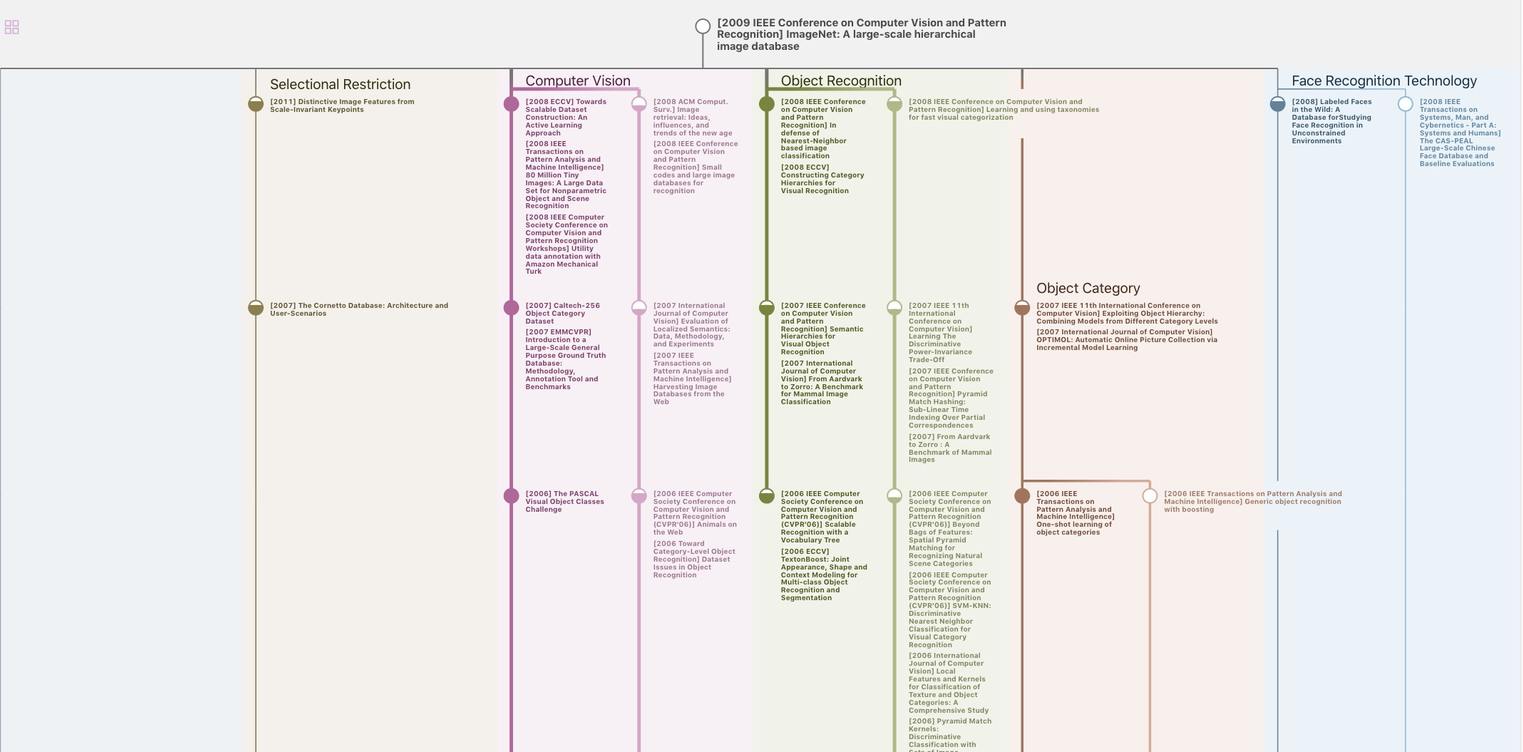
生成溯源树,研究论文发展脉络
Chat Paper
正在生成论文摘要