Approximating high-dimensional infinite-order $U$-statistics: statistical and computational guarantees.
ELECTRONIC JOURNAL OF STATISTICS(2019)
摘要
We study the problem of distributional approximations to high-dimensional non-degenerate U-statistics with random kernels of diverging orders. Infinite-order U-statistics (IOUS) are a useful tool for constructing simultaneous prediction intervals that quantify the uncertainty of ensemble methods such as subbagging and random forests. A major obstacle in using the IOUS is their computational intractability when the sample size and/or order are large. In this article, we derive non-asymptotic Gaussian approximation error bounds for an incomplete version of the IOUS with a random kernel. We also study data-driven inferential methods for the incomplete IOUS via bootstraps and develop their statistical and computational guarantees.
更多查看译文
关键词
Infinite-order U-statistics,incomplete U statistics,Gaussian approximation,bootstrap,random forests,uncertainty quantification
AI 理解论文
溯源树
样例
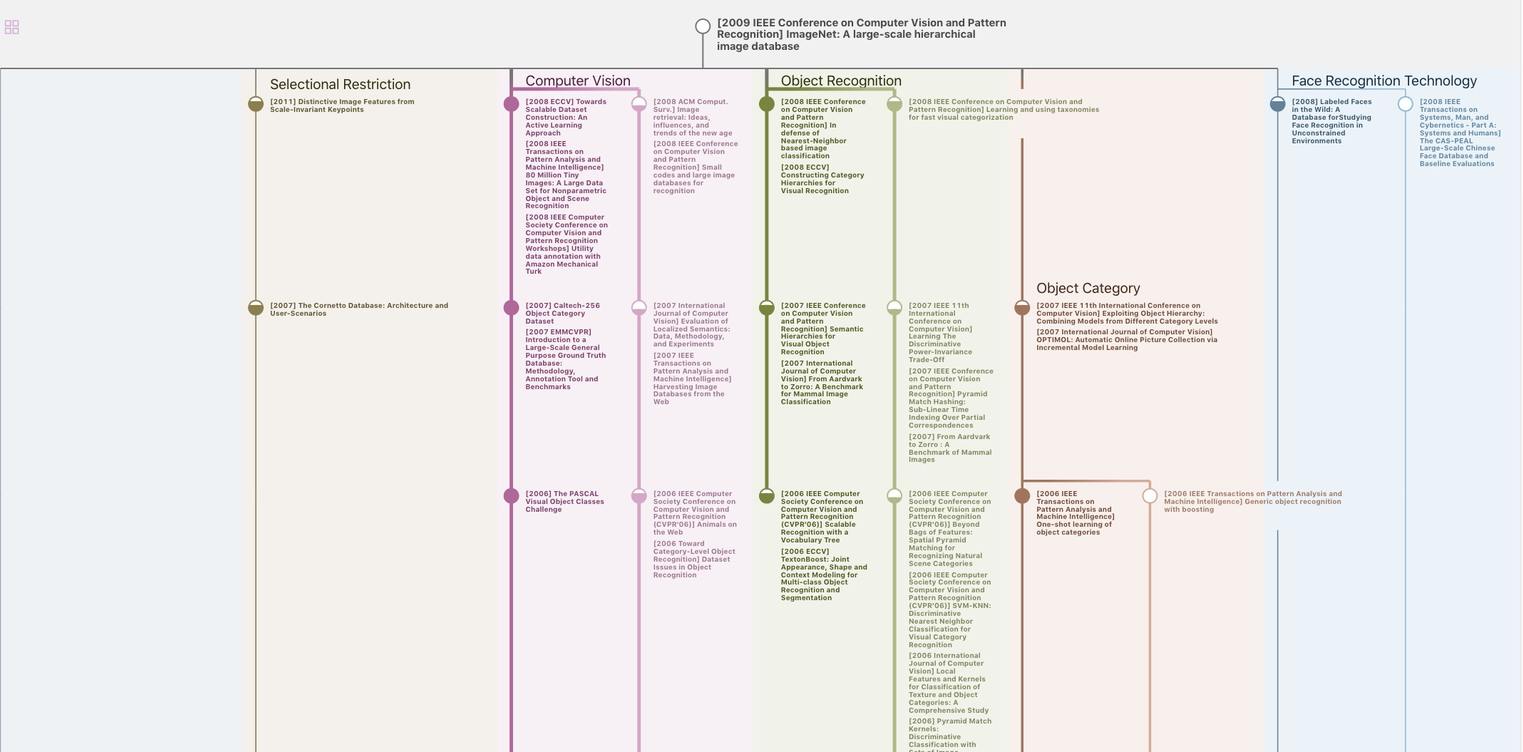
生成溯源树,研究论文发展脉络
Chat Paper
正在生成论文摘要