Semi-supervised deep learning for hyperspectral image classification
REMOTE SENSING LETTERS(2019)
摘要
Recently, a series of deep learning methods based on the convolutional neural networks (CNNs) have been introduced for classification of hyperspectral images (HSIs). However, in order to obtain the optimal parameters, a large number of training samples are required in the CNNs to avoid the overfitting problem. In this paper, a novel method is proposed to extend the training set for deep learning based hyperspectral image classification. First, given a small-sample-size training set, the principal component analysis based edge-preserving features (PCA-EPFs) and extended morphological attribute profiles (EMAPs) are used for HSI classification so as to generate classification probability maps. Second, a large number of pseudo training samples are obtained by the designed decision function which depends on the classification probabilities. Finally, a deep feature fusion network (DFFN) is applied to classify HSI with the training set consists of the original small-sample-size training set and the added pseudo training samples. Experiments performed on several hyperspectral data sets demonstrate the state-of-the-art performance of the proposed method in terms of classification accuracies.
更多查看译文
AI 理解论文
溯源树
样例
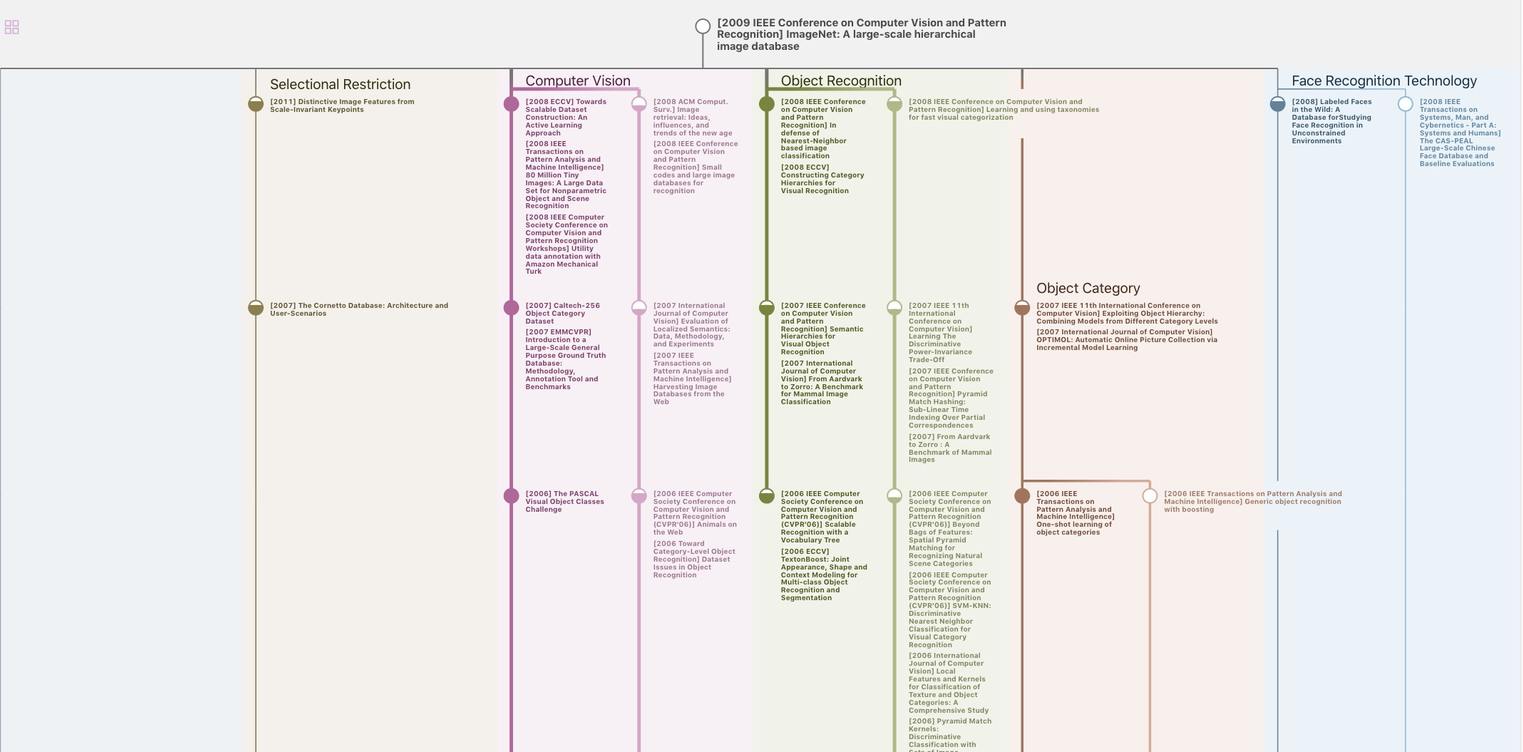
生成溯源树,研究论文发展脉络
Chat Paper
正在生成论文摘要