A Deep Learning Approach to Synthetic Aperture Vector Flow Imaging
2018 IEEE INTERNATIONAL ULTRASONICS SYMPOSIUM (IUS)(2018)
摘要
Many cardiovascular diseases manifest themselves with abnormalities in blood flow. Ultrasound Vector Flow Imaging (VFI) can be used as an angle independent tool to visualize blood flow velocity with high spatio-temporal accuracy. Current methods of VFI include directional beamforming, transverse oscillation, speckle tracking or decorrelation and vector flow mapping. However, aliasing inaccuracies, low signal to noise ratio and the time to post-process limit their clinical application. Recently, Convolutional Neural Networks (CNNs) have shown great success in optical flow estimations. In CNNs the transformation of images into different useful representations results in characteristic learnable features; including the prediction of vector displacement fields. Once trained, CNNs are outperforming existing state of the art hand-engineered solutions both in accuracy and computational cost. In this study we demonstrated the efficacy of our VFI-CNN to estimate vector flow from synthetic aperture 2-D images.
更多查看译文
关键词
angle independent tool,blood flow velocity,optical flow estimations,vector displacement fields,VFI-CNN,deep learning approach,synthetic aperture Vector Flow,cardiovascular diseases,Ultrasound Vector Flow Imaging,synthetic aperture 2D images,high spatiotemporal accuracy,vector flow mapping,directional beamforming,transverse oscillation,speckle tracking,convolutional neural networks
AI 理解论文
溯源树
样例
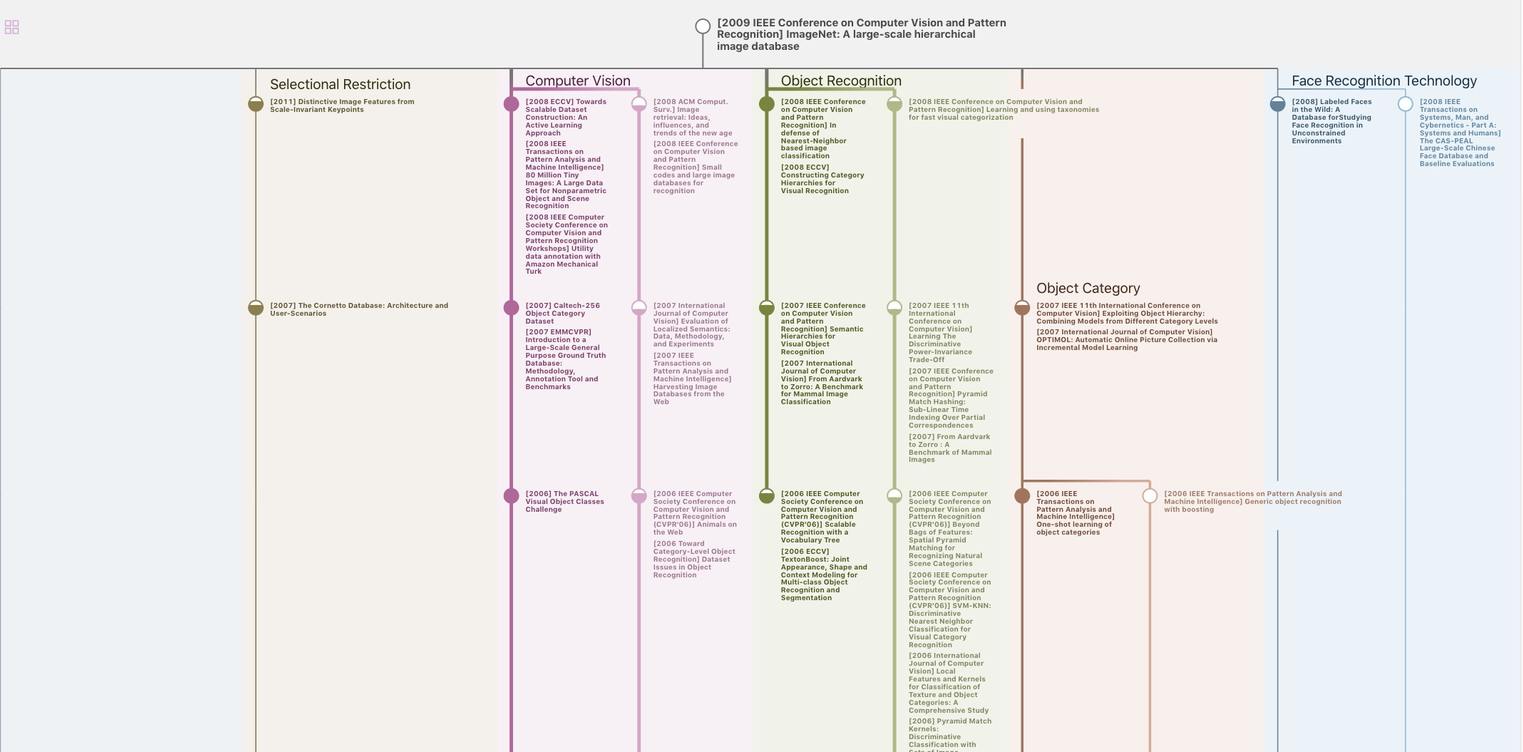
生成溯源树,研究论文发展脉络
Chat Paper
正在生成论文摘要