A hierarchical ensemble learning framework for energy-efficient automatic train driving
Tsinghua Science and Technology(2019)
摘要
Railway transportation plays an important role in modern society. As China's massive railway transportation network continues to grow in total mileage and operation density, the energy consumption of trains becomes a serious concern. For any given route, the geographic characteristics are known a priori, but the parameters (e.g., loading and marshaling) of trains vary from one trip to another. An extensive analysis of the train operation data suggests that the control gear operation of trains is the most important factor that affects the energy consumption. Such an observation determines that the problem of energy-efficient train driving has to be addressed by considering both the geographic information and the trip parameters. However, the problem is difficult to solve due to its high dimension, nonlinearity, complex constraints, and time-varying characteristics. Faced with these difficulties, we propose an energy-efficient train control framework based on a hierarchical ensemble learning approach. Through hierarchical refinement, we learn prediction models of speed and gear. The learned models can be used to derive optimized driving operations under real-time requirements. This study uses random forest and bagging - REPTree as classification algorithm and regression algorithm, respectively. We conduct an extensive study on the potential of bagging, decision trees, random forest, and feature selection to design an effective hierarchical ensemble learning framework. The proposed framework was testified through simulation. The average energy consumption of the proposed method is over 7% lower than that of human drivers.
更多查看译文
关键词
Rail transportation,Optimization,Energy consumption,Gears,Resistance,Real-time systems
AI 理解论文
溯源树
样例
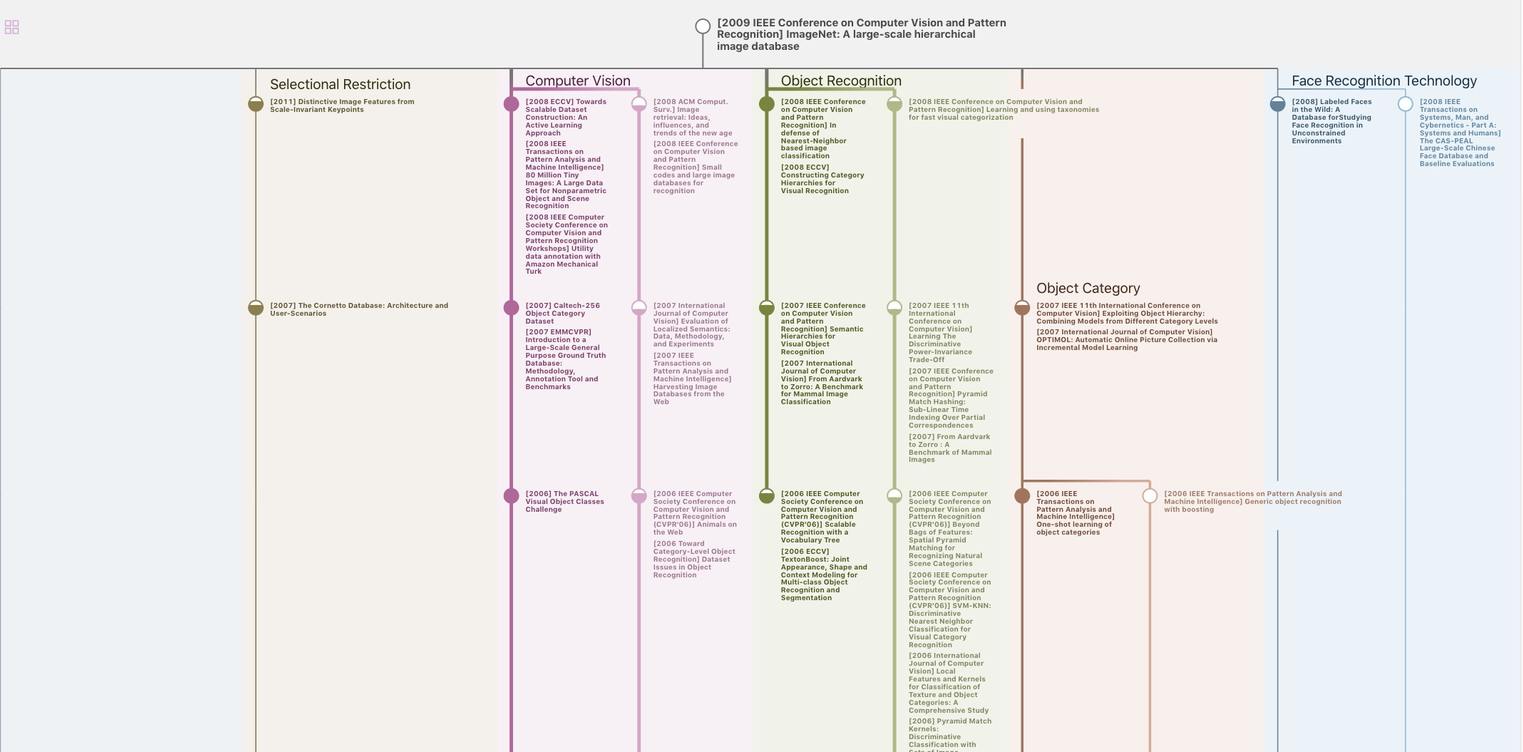
生成溯源树,研究论文发展脉络
Chat Paper
正在生成论文摘要