A Novel Semisupervised Deep Learning Method For Human Activity Recognition
IEEE TRANSACTIONS ON INDUSTRIAL INFORMATICS(2019)
摘要
Human activity recognition (HAR) based on inertial sensors has been investigated for many industrial informatics applications, such as healthcare and ubiquitous computing. Existing methods mainly rely on supervised learning schemes, which require large labeled training data. However, labeled data are sometimes difficult to acquire, while unlabeled data are readily available. Thus, we intend to make use of both labeled and unlabeled data with semisupervised learning for accurate HAR. In this paper, we propose a semisupervised deep learning approach, using temporal ensembling of deep long short-term memory, to recognize human activities with smartphone inertial sensors. With the deep neural network processing, features are extracted for local dependencies in the recurrent framework. Besides, with an ensemble approach based on both labeled and unlabeled data, we can combine together the supervised and unsupervised losses, so as to make good use of unlabeled data that the supervised learning method cannot leverage. Experimental results indicate the effectiveness of our proposed semisupervised learning scheme, when compared to several state-of-the-art semisupervised learning approaches.
更多查看译文
关键词
Deep long short-term memory (DLSTM), human activity recognition, semisupervised learning, temporal ensembling
AI 理解论文
溯源树
样例
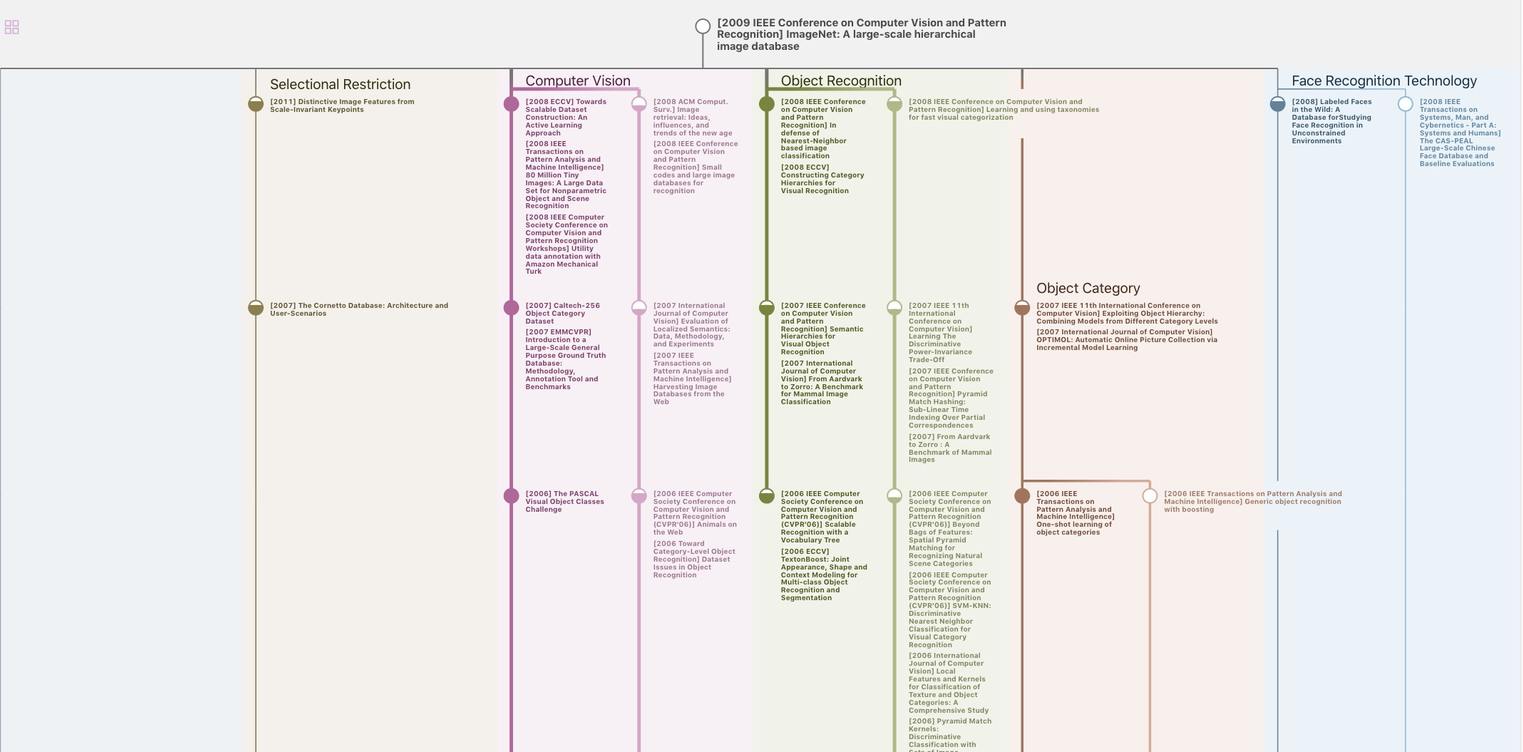
生成溯源树,研究论文发展脉络
Chat Paper
正在生成论文摘要