Autonomous Causally-Driven Explanation Of Actions
PROCEEDINGS 2017 INTERNATIONAL CONFERENCE ON COMPUTATIONAL SCIENCE AND COMPUTATIONAL INTELLIGENCE (CSCI)(2017)
摘要
We propose a cause-effect reasoning mechanism with which an autonomous system can justify planned actions to a human end user. The mechanism is based on a structure we call a "causal plan graph," which encodes the causal relationships between the actions, intentions, and goals of the autonomous system. Causal chains within this graph can potentially serve as intuitive, human-friendly justifications for the autonomous system's planned actions. A prototype of this mechanism is tested in simulation on a set of planning problems from an autonomous maintenance scenario. We demonstrate empirically that shortest path algorithms can effectively reduce a very large number of possible causal chains to a small, intelligible subset that might reasonably be inspected and ranked by a human. Consequently this work can serve as the basis for an experimental platform for future end user studies with human participants.
更多查看译文
关键词
cause-effect reasoning, explainable artificial intelligence (XAI), imitation learning, robotics
AI 理解论文
溯源树
样例
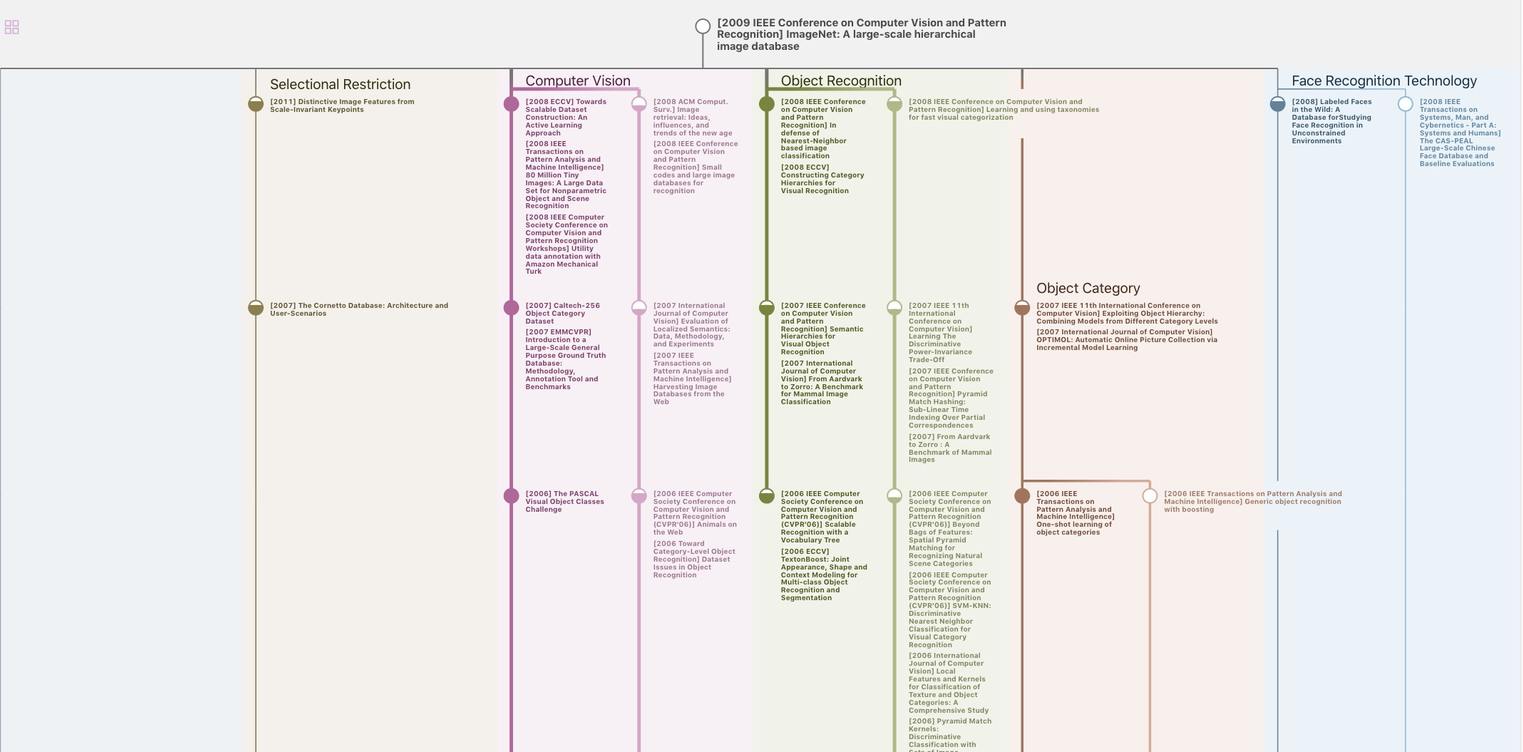
生成溯源树,研究论文发展脉络
Chat Paper
正在生成论文摘要