Dimensionality reduction based on binary encoding for hyperspectral data
INTERNATIONAL JOURNAL OF REMOTE SENSING(2019)
摘要
Binary encoding is an approach that aims at summarizing the information contained in various spectral bands into a single image that stores the meaningful information of the bands. In this paper, it is introduced a feature extraction approach to reduce the dimensionality of hyperspectral data with binary encoding for classification purposes. Different options to reduce the radiometric information of the pixels are introduced, such as using a single threshold or multiple thresholds. After the dimensionality reduction, the separation of the spectral classes was analysed and the thematic classification of the reduced data was performed. In order to evaluate the performance of the proposed approach, experiments on AVIRIS (Airborne Visible/Infrared Imaging Spectrometer) image, ROSIS (Reflection Optics System Imaging Spectrometer) hyperspectral image and HYDICE (Hyperspectral Digital Imagery Collection Experiment) hyperspectral image are presented. In the experiments, neighbouring spectral bands are grouped and coded and the results of the classification are compared. The results show that the use of binary encoding based on three thresholds by spectral region is more efficient than with the use of one threshold. The thematic mapping of the hyperspectral data with reduced dimension confirms the competitiveness of the binary encoding method compared with other dimension reduction methods, such as the Principal Component Analysis (PCA), the Principal Component Analysis - Fisher's Linear Discriminant Analysis (PCA-LDA), the Discriminant Analysis Feature Extraction (DAFE) and the Non-parametric Weighted Feature Extraction (NWFE). In this context, the present methodology shows to be promising, because it reduces the computational complexity and improves performance.
更多查看译文
AI 理解论文
溯源树
样例
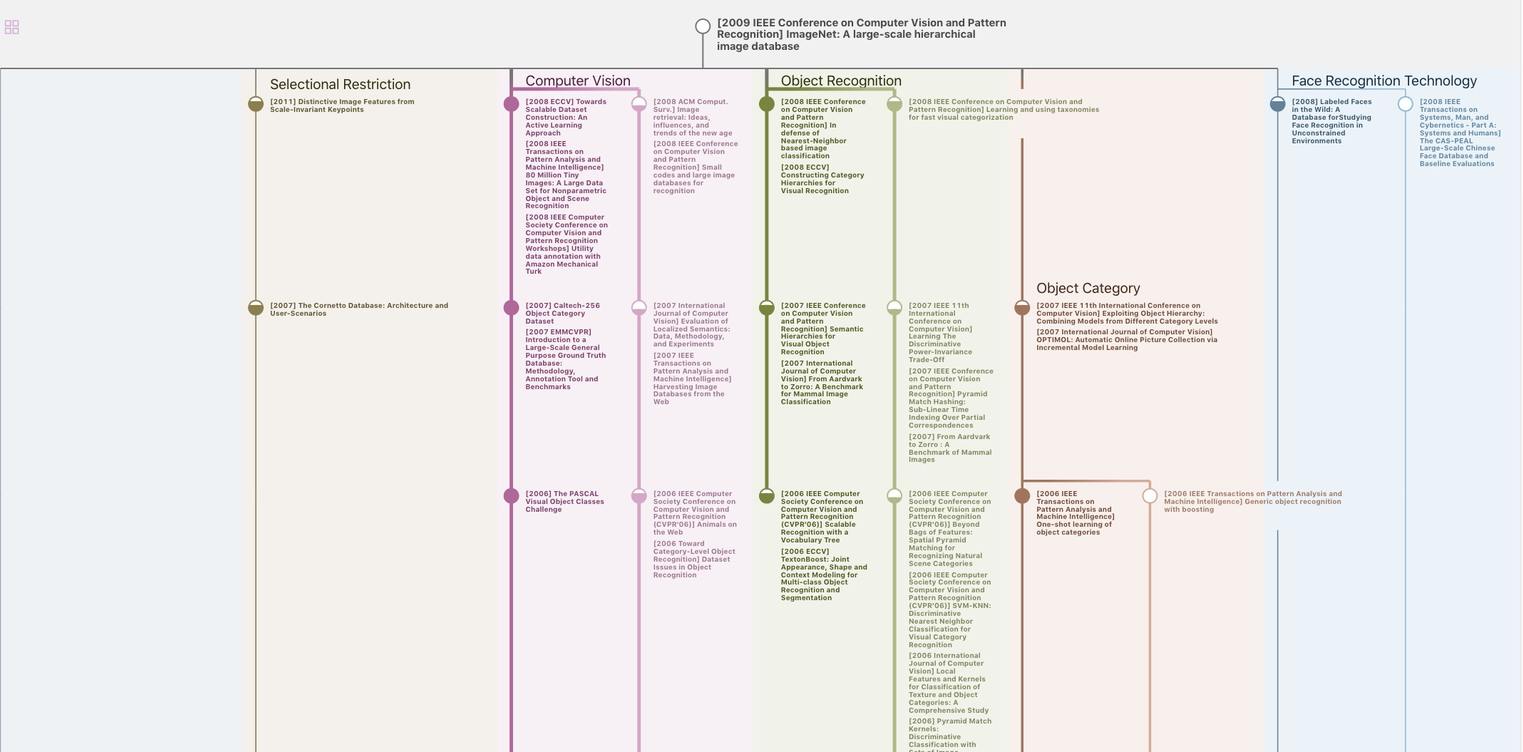
生成溯源树,研究论文发展脉络
Chat Paper
正在生成论文摘要