Bayes linear analysis of risks in sequential optimal design problems
ELECTRONIC JOURNAL OF STATISTICS(2018)
摘要
In a statistical or physical model, it is often the case that a set of design inputs must be selected in order to perform an experiment to collect data with which to update beliefs about a set of model parameters; frequently, the model also depends on a set of external variables which are unknown before the experiment is carried out, but which cannot be controlled. Sequential optimal design problems are concerned with selecting these design inputs in stages (at different points in time), such that the chosen design is optimal with respect to the set of possible outcomes of all future experiments which might be carried out. Such problems are computationally expensive. We consider the calculations which must be performed in order to solve a sequential design problem, and we propose a framework using Bayes linear emulators to approximate all difficult calculations which arise; these emulators are designed so that we can easily approximate expectations of the risk by integrating the emulator directly, and so that we can efficiently search the design input space for settings which may be optimal. We also consider how the structure of the design calculation can be exploited to improve the quality of the fitted emulators. Our framework is demonstrated through application to a simple linear modelling problem, and to a more complex airborne sensing problem, in which a sequence of aircraft flight paths must be designed so as to collect data which are informative for the locations of ground-based gas sources.
更多查看译文
关键词
Bayesian uncertainty analysis,emulation,sequential experimental design,decision support,remote sensing,uncertainty quantification
AI 理解论文
溯源树
样例
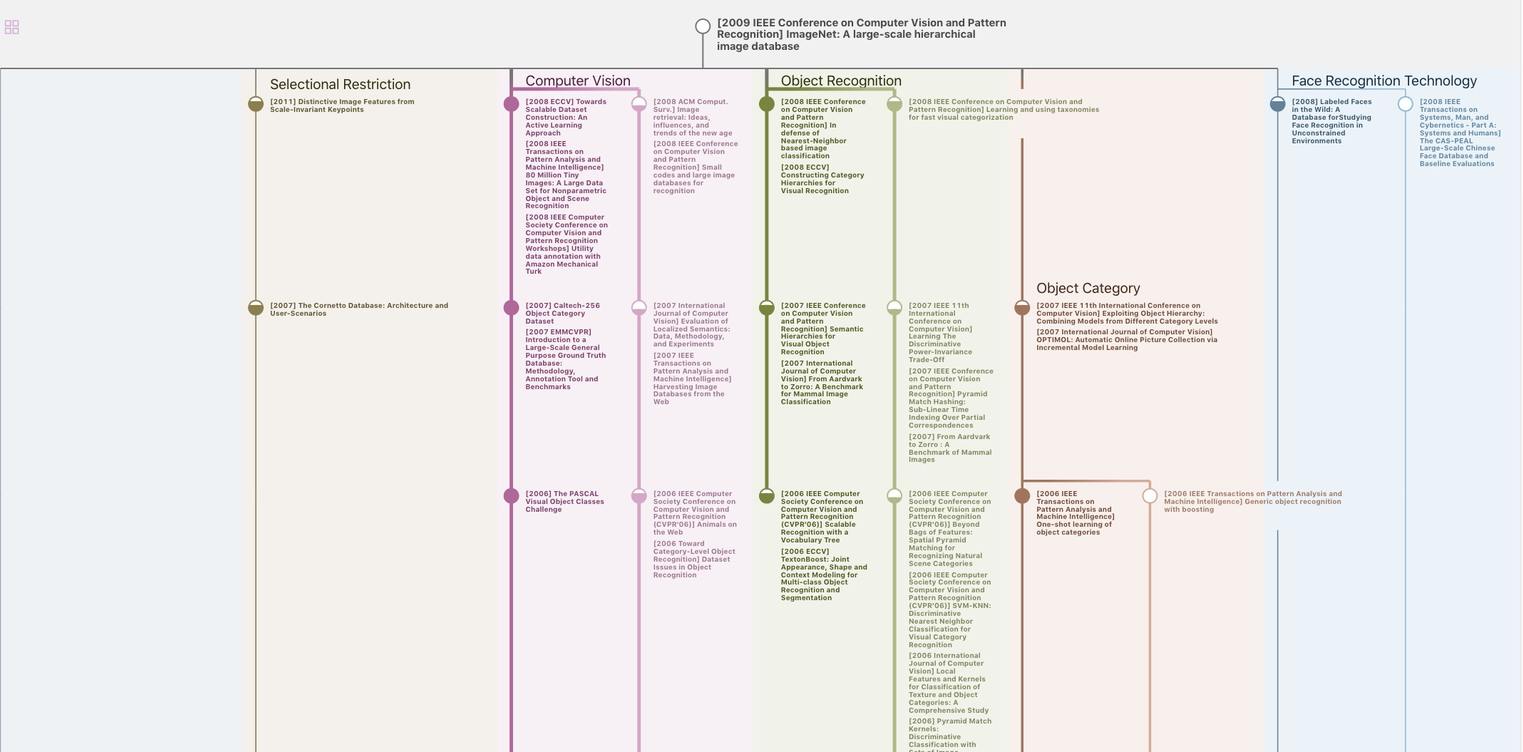
生成溯源树,研究论文发展脉络
Chat Paper
正在生成论文摘要