A Data-driven Unified Framework for Predicting Citation Dynamics
IEEE Transactions on Big Data(2020)
摘要
With the rising interest in predicting the scientific output, various efforts have been made to predict a scientistu0027s h-index or the citation trajectory of a publication. In this work, we employ a dynamic categorization scientists to ensure at each stage of their careers a comparison amongst their peers combine this grouping with predictive models to estimate a scientistu0027s future impact, as expressed by citation counts. Moreover, we investigate a wide range of factors identifying their importance in determining the future of science different performance academic levels with particular emphasis on features describing a scholaru0027s position in multi-layered collaboration citation networks. The robustness of the approach is examined on a longitudinal dataset centered around 700,302 data points representing Computer Scientists in various time periods with their complete networks of over 18 million collaboration links 36 million citations. Our results indicate up to 30% improvement in prediction performance compared to baseline methods along with an average $R^2 = 0.96$ for short term and $R^2 = 0.91$ for long term predictions.
更多查看译文
关键词
Citation analysis,scientometrics,impact prediction,clustering
AI 理解论文
溯源树
样例
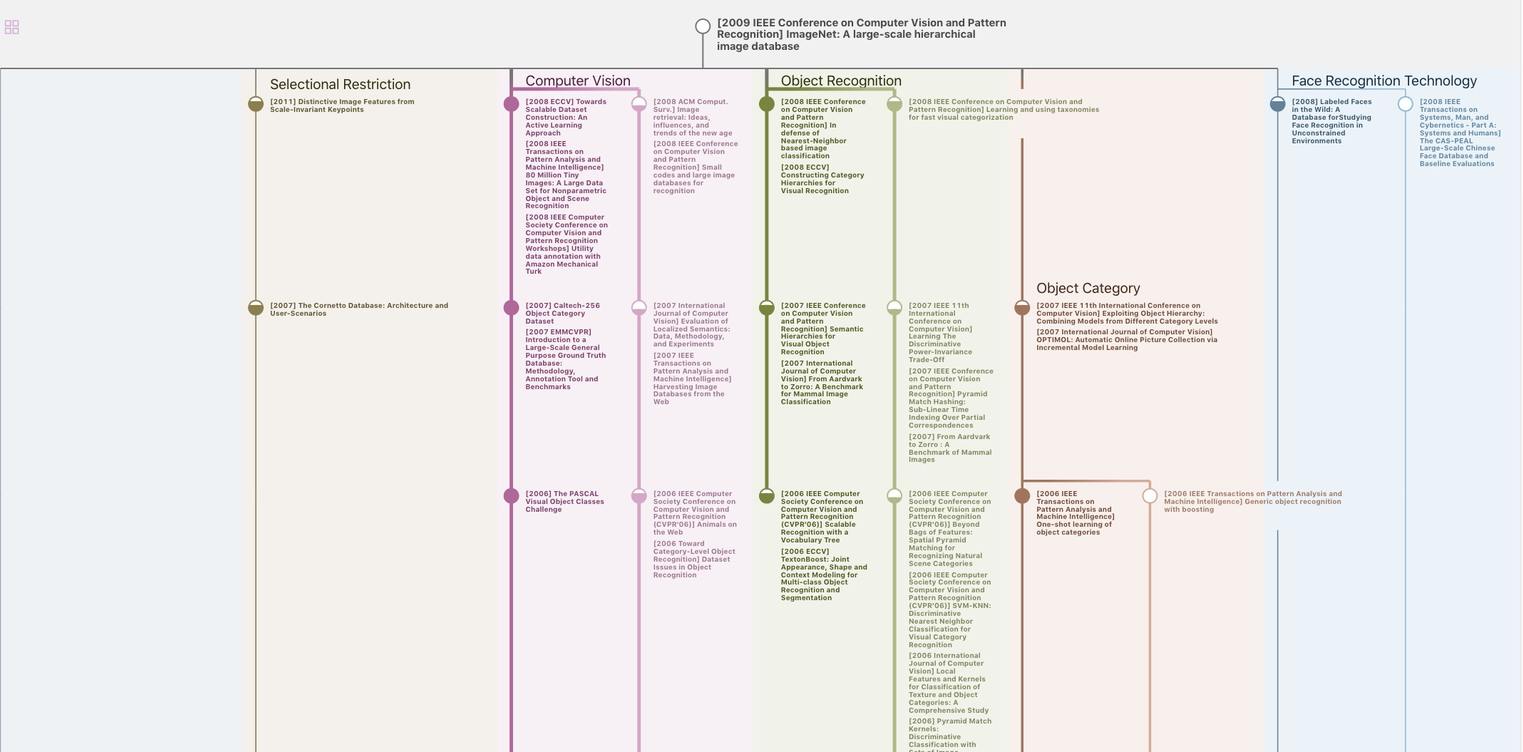
生成溯源树,研究论文发展脉络
Chat Paper
正在生成论文摘要