Learning a Visual Tracker from a Single Movie without Annotation
THIRTY-THIRD AAAI CONFERENCE ON ARTIFICIAL INTELLIGENCE / THIRTY-FIRST INNOVATIVE APPLICATIONS OF ARTIFICIAL INTELLIGENCE CONFERENCE / NINTH AAAI SYMPOSIUM ON EDUCATIONAL ADVANCES IN ARTIFICIAL INTELLIGENCE(2019)
摘要
The recent success of deep network in visual trackers learning largely relies on human labeled data, which are however expensive to annotate. Recently, some unsupervised methods have been proposed to explore the learning of visual trackers without labeled data, while their performance lags far behind the supervised methods. We identify the main bottleneck of these methods as inconsistent objectives between off-line training and online tracking stages. To address this problem, we propose a novel unsupervised learning pipeline which is based on the discriminative correlation filter network. Our method iteratively updates the tracker by alternating between target localization and network optimization. In particular, we propose to learn the network from a single movie, which could be easily obtained other than collecting thousands of video clips or millions of images. Extensive experiments demonstrate that our approach is insensitive to the employed movies, and the trained visual tracker achieves leading performance among existing unsupervised learning approaches. Even compared with the same network trained with human labeled bounding boxes, our tracker achieves similar results on many tracking benchmarks. Code is available at: https://github.com/ZijConan/UL-Tracker-AAA12019.
更多查看译文
AI 理解论文
溯源树
样例
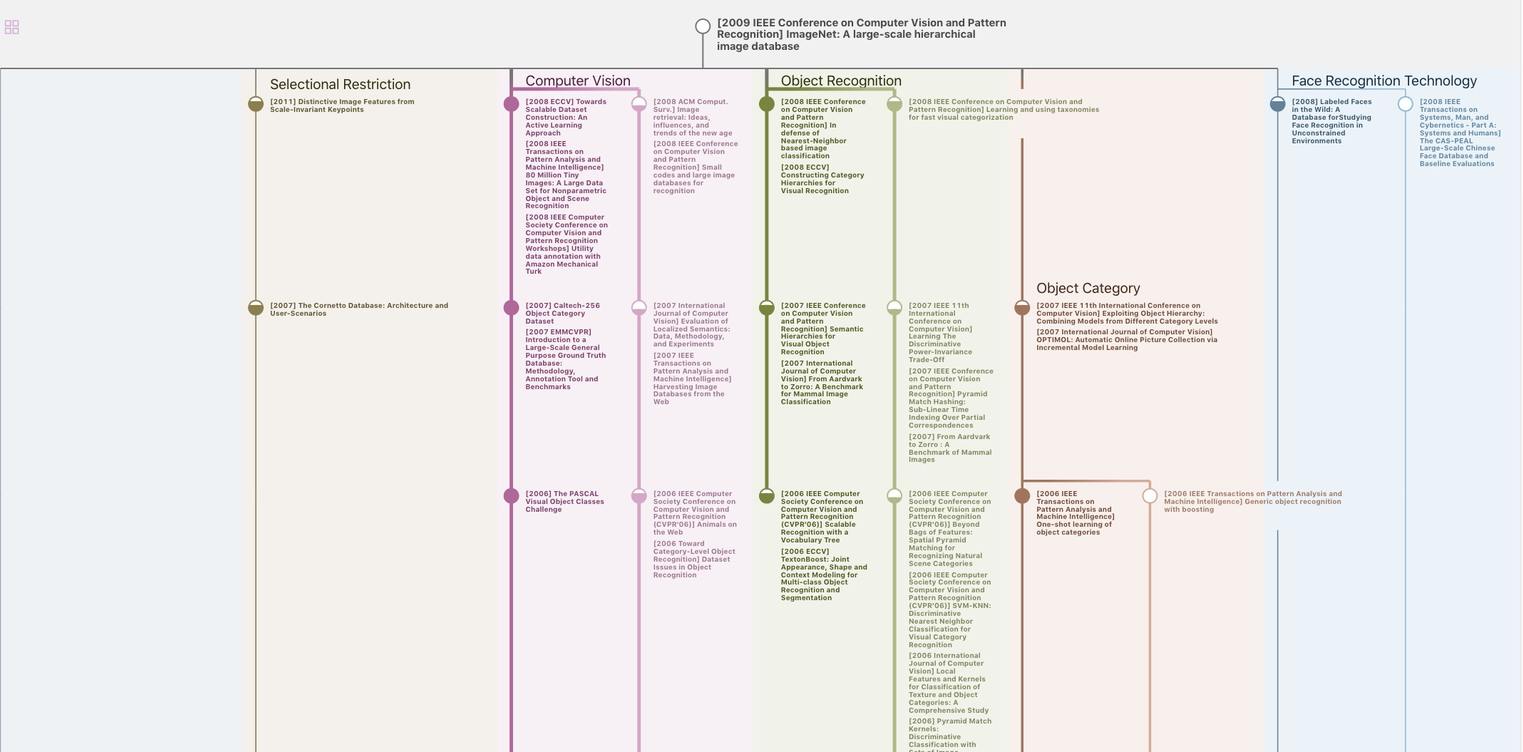
生成溯源树,研究论文发展脉络
Chat Paper
正在生成论文摘要